Dual-Gated Fusion with Prefix-Tuning for Multi-Modal Relation Extraction
conf_acl(2023)
摘要
Multi-Modal Relation Extraction (MMRE) aims at identifying the relation between two entities in texts that contain visual clues. Rich visual content is valuable for the MMRE task, but existing works cannot well model finer associations among different modalities, failing to capture the truly helpful visual information and thus limiting relation extraction performance. In this paper, we propose a novel MMRE framework to better capture the deeper correlations of text, entity pair, and image/objects, so as to mine more helpful information for the task, termed as DGF-PT. We first propose a prompt-based autoregressive encoder, which builds the associations of intra-modal and inter-modal features related to the task, respectively by entity-oriented and object-oriented prefixes. To better integrate helpful visual information, we design a dual-gated fusion module to distinguish the importance of image/objects and further enrich text representations. In addition, a generative decoder is introduced with entity type restriction on relations, better filtering out candidates. Extensive experiments conducted on the benchmark dataset show that our approach achieves excellent performance compared to strong competitors, even in the few-shot situation.
更多查看译文
关键词
fusion,relation,dual-gated,prefix-tuning,multi-modal
AI 理解论文
溯源树
样例
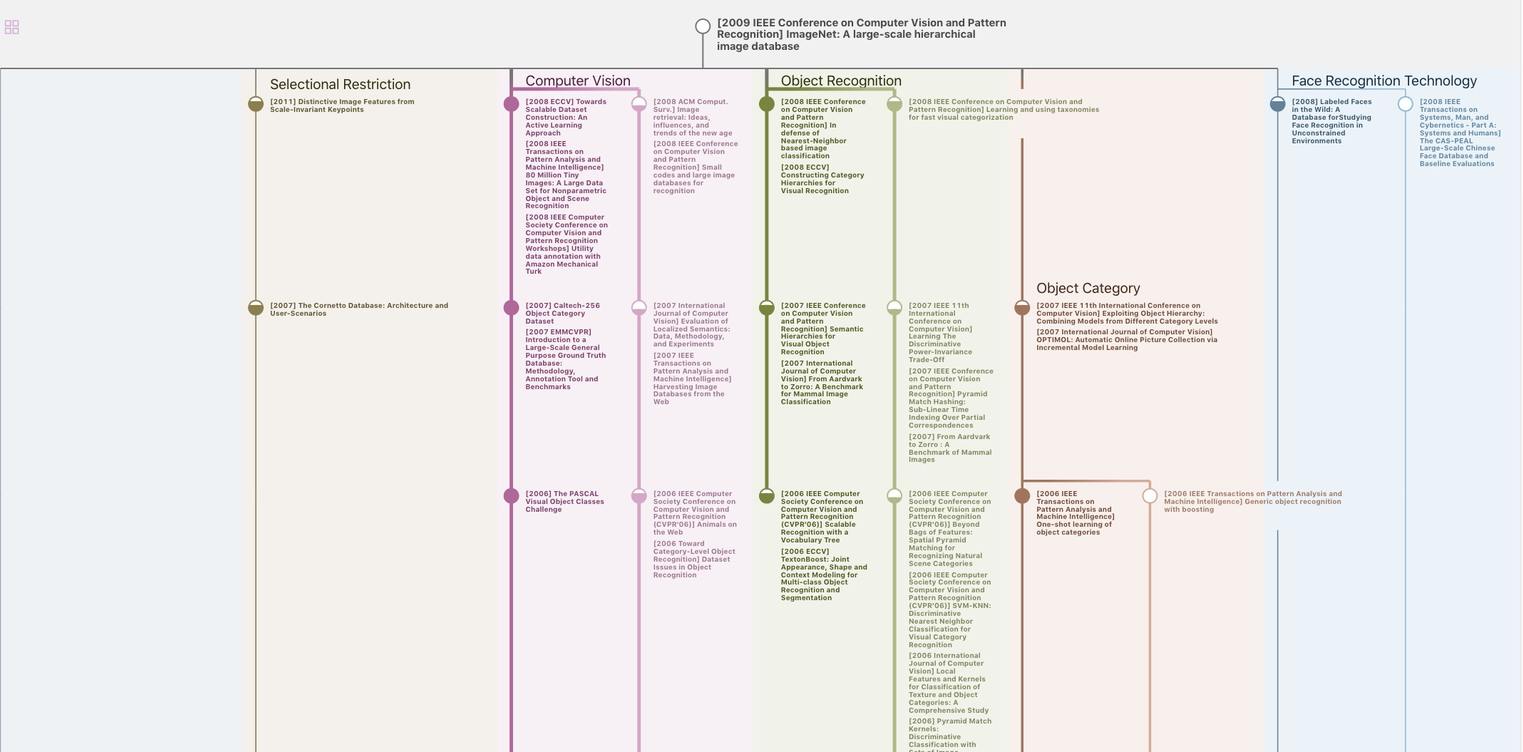
生成溯源树,研究论文发展脉络
Chat Paper
正在生成论文摘要