Performance of data-driven inner speech decoding with same-task EEG-fMRI data fusion and bimodal models
CoRR(2023)
摘要
Decoding inner speech from the brain signal via hybridisation of fMRI and EEG data is explored to investigate the performance benefits over unimodal models. Two different bimodal fusion approaches are examined: concatenation of probability vectors output from unimodal fMRI and EEG machine learning models, and data fusion with feature engineering. Same task inner speech data are recorded from four participants, and different processing strategies are compared and contrasted to previously-employed hybridisation methods. Data across participants are discovered to encode different underlying structures, which results in varying decoding performances between subject-dependent fusion models. Decoding performance is demonstrated as improved when pursuing bimodal fMRI-EEG fusion strategies, if the data show underlying structure.
更多查看译文
关键词
data-driven fusion,inner speech,same-task,eeg-fmri
AI 理解论文
溯源树
样例
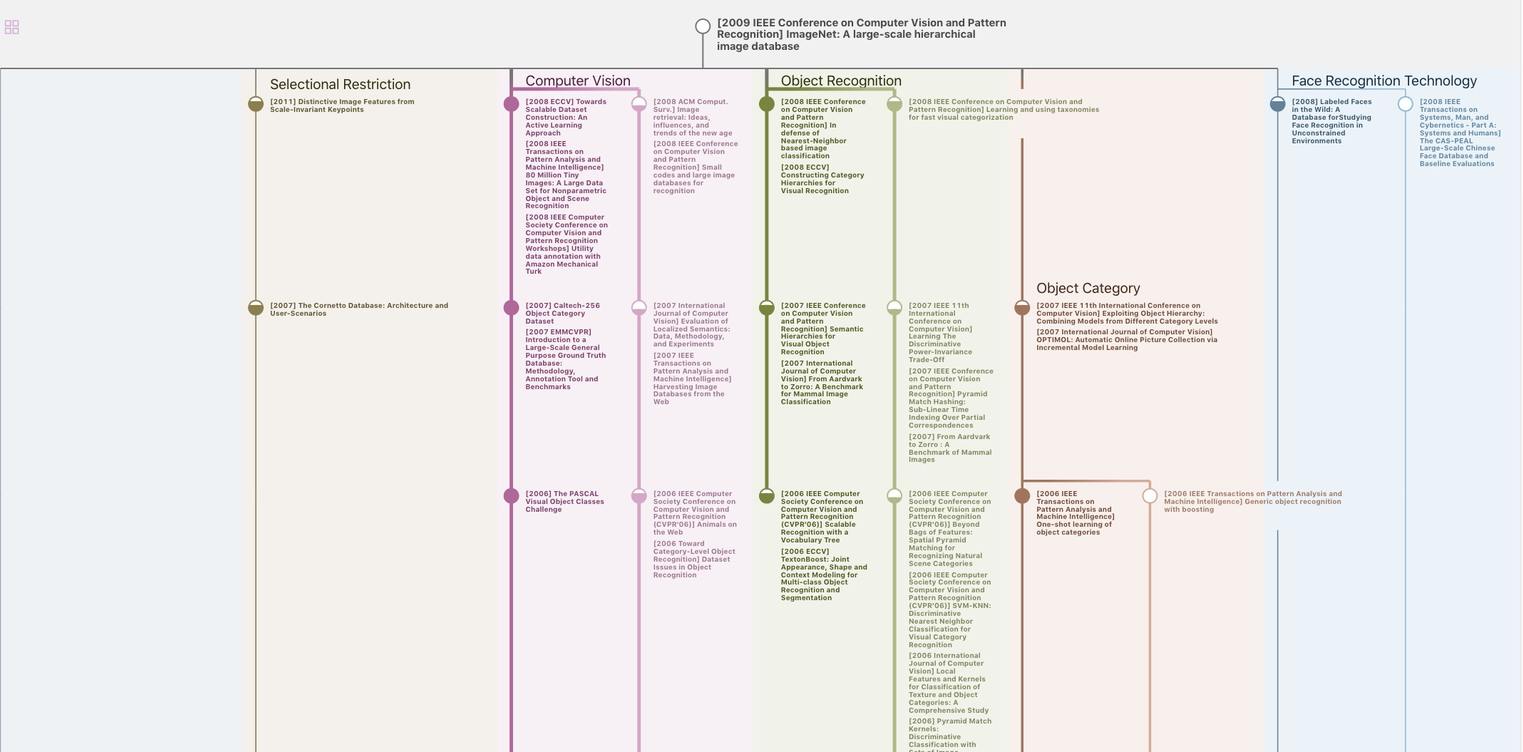
生成溯源树,研究论文发展脉络
Chat Paper
正在生成论文摘要