Unsupervised Open-domain Keyphrase Generation
conf_acl(2023)
摘要
In this work, we study the problem of unsupervised open-domain keyphrase generation, where the objective is a keyphrase generation model that can be built without using human-labeled data and can perform consistently across domains. To solve this problem, we propose a seq2seq model that consists of two modules, namely \textit{phraseness} and \textit{informativeness} module, both of which can be built in an unsupervised and open-domain fashion. The phraseness module generates phrases, while the informativeness module guides the generation towards those that represent the core concepts of the text. We thoroughly evaluate our proposed method using eight benchmark datasets from different domains. Results on in-domain datasets show that our approach achieves state-of-the-art results compared with existing unsupervised models, and overall narrows the gap between supervised and unsupervised methods down to about 16\%. Furthermore, we demonstrate that our model performs consistently across domains, as it overall surpasses the baselines on out-of-domain datasets.
更多查看译文
关键词
generation,open-domain
AI 理解论文
溯源树
样例
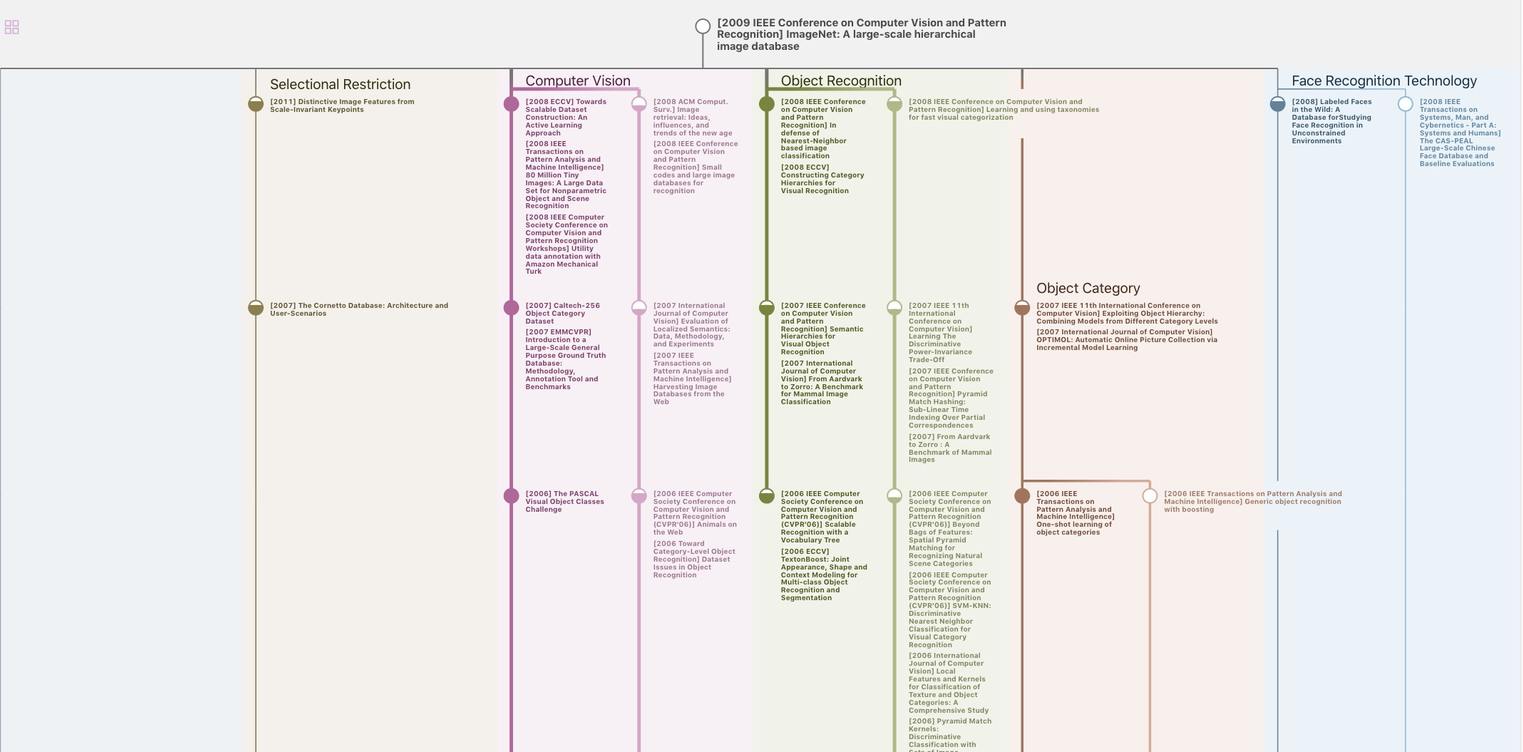
生成溯源树,研究论文发展脉络
Chat Paper
正在生成论文摘要