Data-Heterogeneous Hierarchical Federated Learning with Mobility
CoRR(2023)
摘要
Federated learning enables distributed training of machine learning (ML) models across multiple devices in a privacy-preserving manner. Hierarchical federated learning (HFL) is further proposed to meet the requirements of both latency and coverage. In this paper, we consider a data-heterogeneous HFL scenario with mobility, mainly targeting vehicular networks. We derive the convergence upper bound of HFL with respect to mobility and data heterogeneity, and analyze how mobility impacts the performance of HFL. While mobility is considered as a challenge from a communication point of view, our goal here is to exploit mobility to improve the learning performance by mitigating data heterogeneity. Simulation results verify the analysis and show that mobility can indeed improve the model accuracy by up to 15.1\% when training a convolutional neural network on the CIFAR-10 dataset using HFL.
更多查看译文
关键词
mobility,learning,data-heterogeneous
AI 理解论文
溯源树
样例
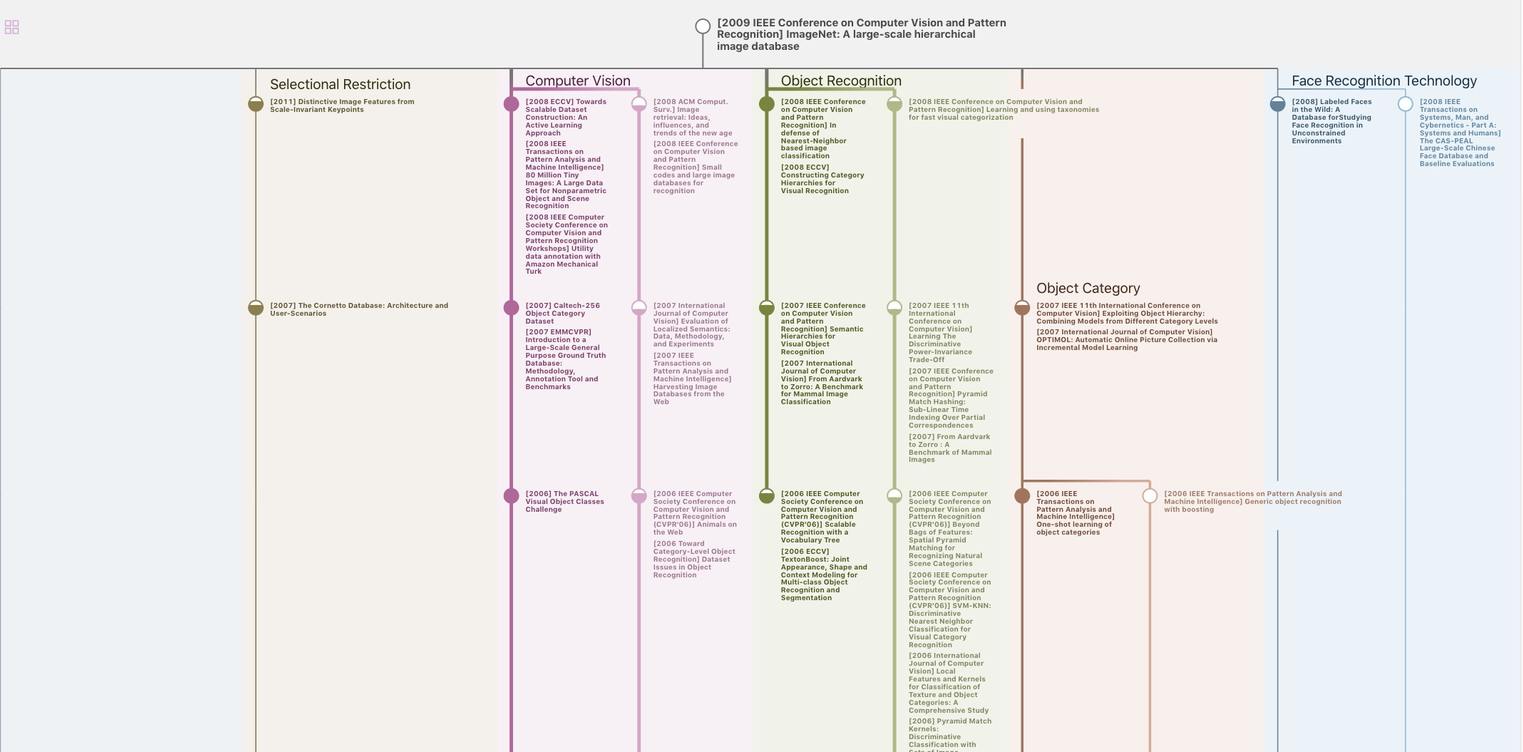
生成溯源树,研究论文发展脉络
Chat Paper
正在生成论文摘要