Learning High-Dimensional Nonparametric Differential Equations via Multivariate Occupation Kernel Functions
CoRR(2023)
摘要
Learning a nonparametric system of ordinary differential equations (ODEs) from $n$ trajectory snapshots in a $d$-dimensional state space requires learning $d$ functions of $d$ variables. Explicit formulations scale quadratically in $d$ unless additional knowledge about system properties, such as sparsity and symmetries, is available. In this work, we propose a linear approach to learning using the implicit formulation provided by vector-valued Reproducing Kernel Hilbert Spaces. By rewriting the ODEs in a weaker integral form, which we subsequently minimize, we derive our learning algorithm. The minimization problem's solution for the vector field relies on multivariate occupation kernel functions associated with the solution trajectories. We validate our approach through experiments on highly nonlinear simulated and real data, where $d$ may exceed 100. We further demonstrate the versatility of the proposed method by learning a nonparametric first order quasilinear partial differential equation.
更多查看译文
关键词
kernel,learning,high-dimensional
AI 理解论文
溯源树
样例
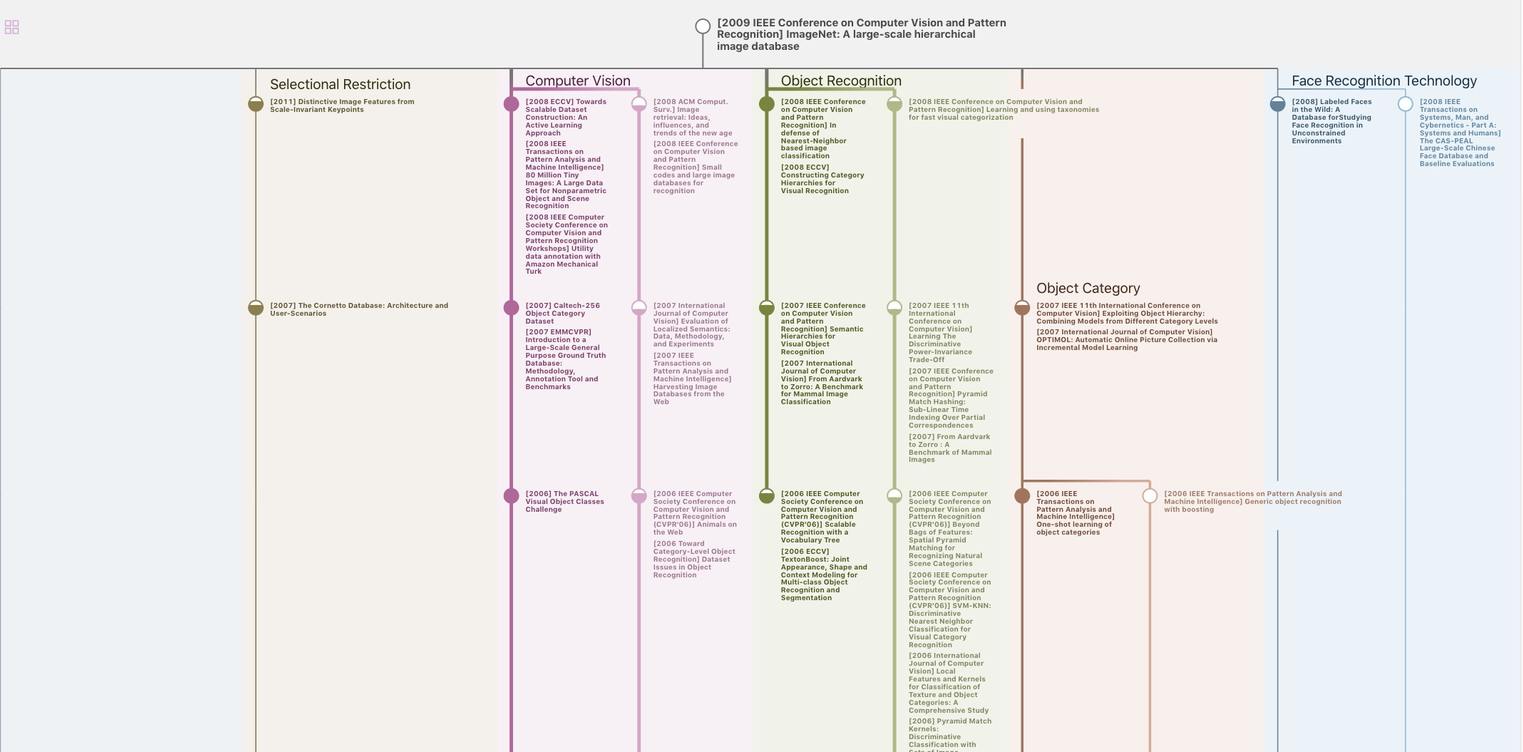
生成溯源树,研究论文发展脉络
Chat Paper
正在生成论文摘要