DeepWiPos: A Deep Learning-Based Wireless Positioning Framework to Address Fingerprint Instability
IEEE Transactions on Vehicular Technology(2023)
摘要
Wireless fingerprint localization technologies have drawn significant attention because of their ubiquitous infrastructure and low energy consumption. However, they often suffer from the Received Signal Strength (RSS) instability (i.e., RSS variations across heterogeneous devices and RSS fluctuations over time) and fingerprint spatial ambiguity issues. Although various deep learning-based methods have been proposed recently, most of them only pursue remarkable accuracy in an ideal environment and escape the above-mentioned problems. The co-existence of these factors would make most existing methods suboptimal or invalid when applied in real scenarios. In this paper, to address the RSS instability, we define the fingerprint spatial gradient (FSG), which can convert the vulnerable and unstable fingerprints to stable relative values but lose absolute information for positioning. Next, to relieve the fingerprint instability and model the temporal sequential dependencies in a trajectory, we propose a Long Short-Term Memory network (LSTM)-based framework to fuse FSG and RSS, where an attention module is used to guide the fusion according to their contributions to the result. Finally, the beam search strategy is used to search for the optimal solution from outputs of the LSTM, eliminating the spatial ambiguity of fingerprints. The experiments show that DeepWiPos reduces average positioning error by 23.44% and 22.18% on Bluetooth and Wi-Fi, respectively when compared with the state-of-the-art.
更多查看译文
关键词
Deep learning,fingerprint spatial gradient,indoor positioning,LSTM
AI 理解论文
溯源树
样例
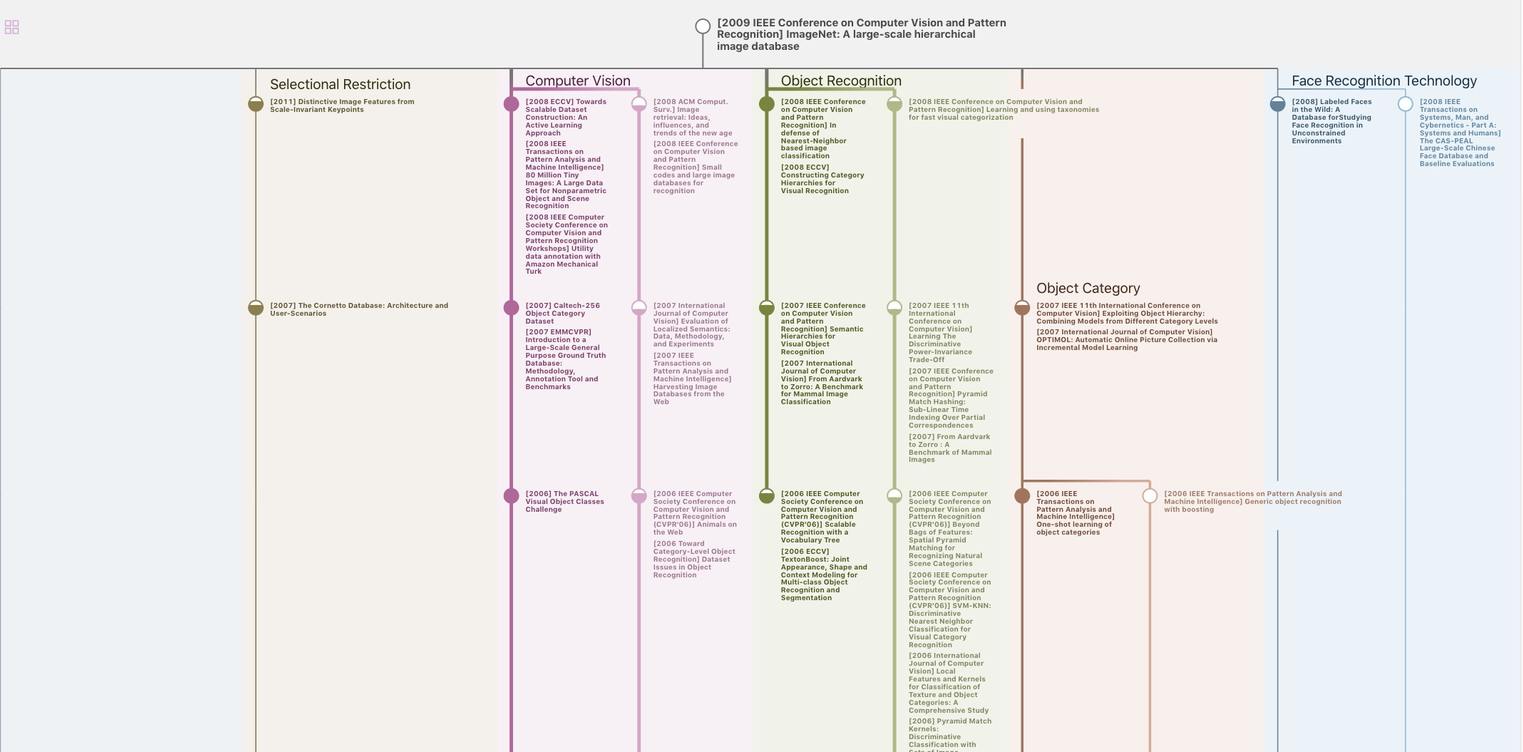
生成溯源树,研究论文发展脉络
Chat Paper
正在生成论文摘要