Supplementary Learning Control for Energy Management Strategy of Hybrid Electric Vehicles at Scale
IEEE Transactions on Vehicular Technology(2023)
Abstract
Deep reinforcement learning (DRL) based energy management strategy (EMS) for hybrid electric vehicles (HEVs) has shown its effectiveness in energy saving. However, there is a huge gap between simulation and the real application of DRL-based methods due to its uncertainty and low converge speed. This paper proposes a supplementary learning controller (SLC) based on DRL to compensate for an existing rule-based EMS. The proposed SLC searches for an optimal solution for the action which works alongside the rule-based EMS. Due to the existing rule-based EMS, the SLC can get an optimal solution while reducing the uncertainty of algorithm to the system. In order to improve the converge speed, a distributed architecture is designed for the DRL-based HEV SLC at scale. The actor of SLC in each vehicle interacts with its own driving cycle by searching actions according to a shared neural network, and sends the experience to the cloud. The learner in the cloud replays samples of experience and updates the neural network. The performance of the proposed SLC for HEV EMS at scale was demonstrated in different conditions. The proposed HEV SLC is a good step for the DRL-based HEV EMS method transferring from simulation to real application.
MoreTranslated text
Key words
Deep reinforcement learning,energy management strategy,hybrid electric vehicle,supplementary learning
AI Read Science
Must-Reading Tree
Example
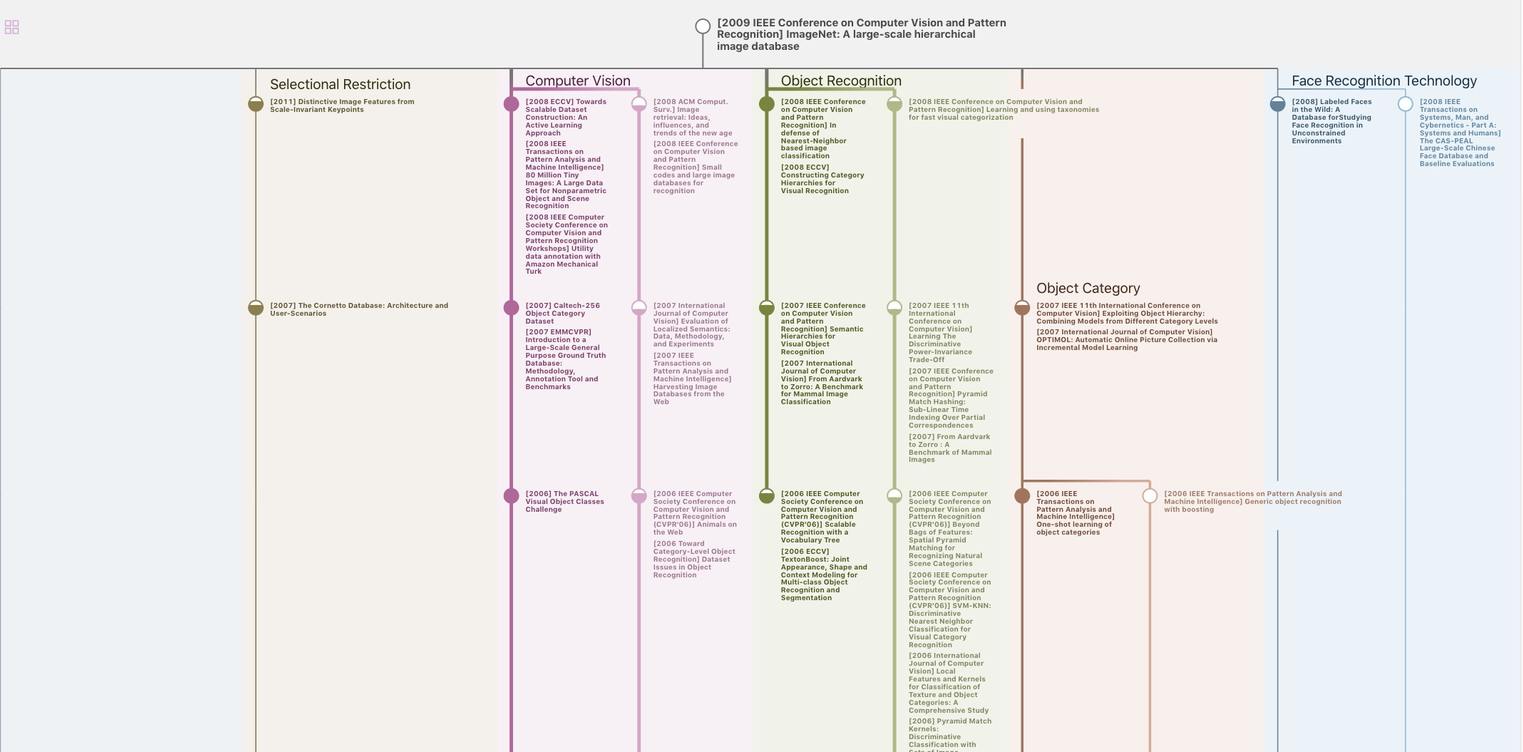
Generate MRT to find the research sequence of this paper
Chat Paper
Summary is being generated by the instructions you defined