Crowdsourcing and Evaluating Text-Based Audio Retrieval Relevances
CoRR(2023)
摘要
This paper explores grading text-based audio retrieval relevances with crowdsourcing assessments. Given a free-form text (e.g., a caption) as a query, crowdworkers are asked to grade audio clips using numeric scores (between 0 and 100) to indicate their judgements of how much the sound content of an audio clip matches the text, where 0 indicates no content match at all and 100 indicates perfect content match. We integrate the crowdsourced relevances into training and evaluating text-based audio retrieval systems, and evaluate the effect of using them together with binary relevances from audio captioning. Conventionally, these binary relevances are defined by captioning-based audio-caption pairs, where being positive indicates that the caption describes the paired audio, and being negative applies to all other pairs. Experimental results indicate that there is no clear benefit from incorporating crowdsourced relevances alongside binary relevances when the crowdsourced relevances are binarized for contrastive learning. Conversely, the results suggest that using only binary relevances defined by captioning-based audio-caption pairs is sufficient for contrastive learning.
更多查看译文
关键词
audio,text-based
AI 理解论文
溯源树
样例
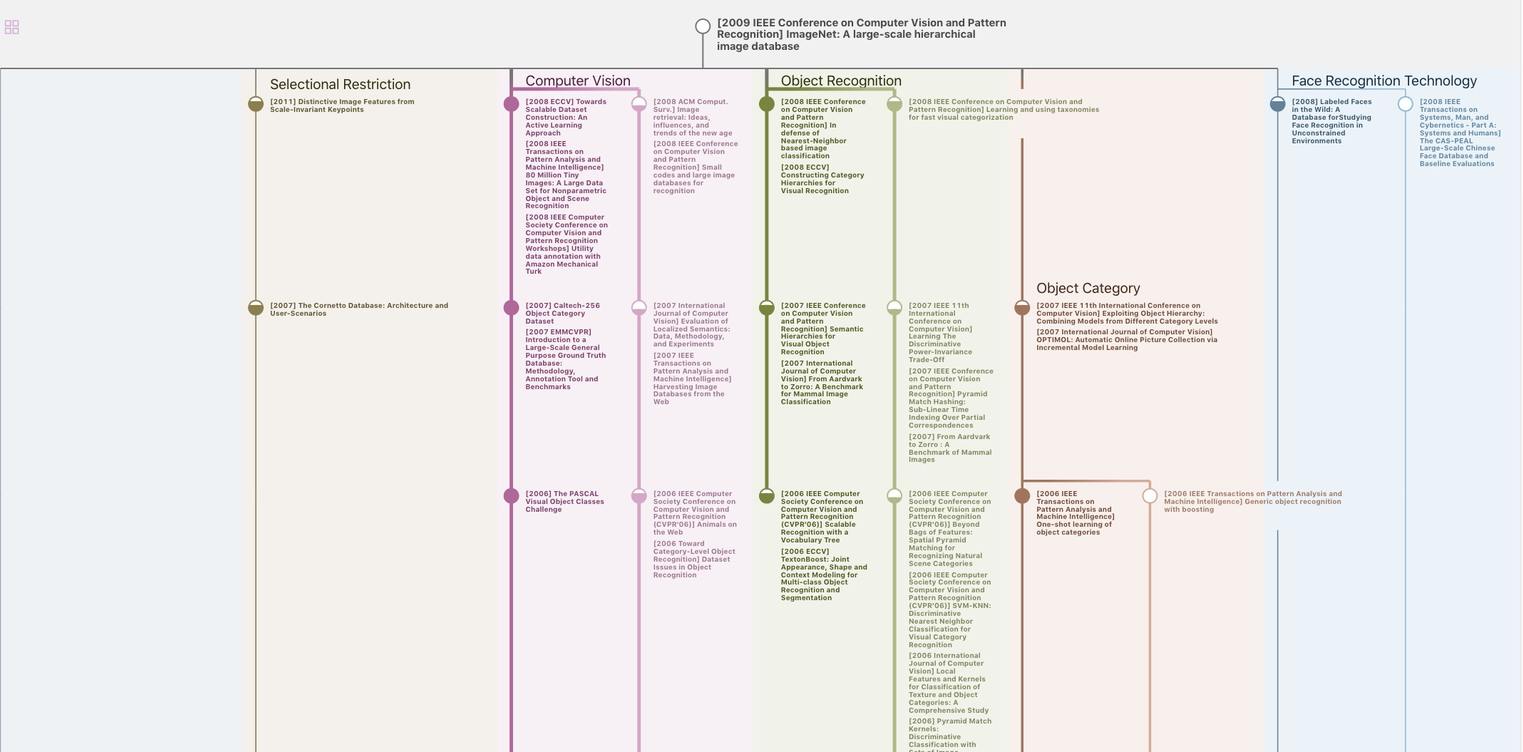
生成溯源树,研究论文发展脉络
Chat Paper
正在生成论文摘要