EVOPOSE: A Recursive Transformer For 3D Human Pose Estimation With Kinematic Structure Priors
CoRR(2023)
Abstract
Transformer is popular in recent 3D human pose estimation, which utilizes long-term modeling to lift 2D keypoints into the 3D space. However, current transformer-based methods do not fully exploit the prior knowledge of the human skeleton provided by the kinematic structure. In this paper, we propose a novel transformer-based model EvoPose to introduce the human body prior knowledge for 3D human pose estimation effectively. Specifically, a Structural Priors Representation (SPR) module represents human priors as structural features carrying rich body patterns, e.g. joint relationships. The structural features are interacted with 2D pose sequences and help the model to achieve more informative spatiotemporal features. Moreover, a Recursive Refinement (RR) module is applied to refine the 3D pose outputs by utilizing estimated results and further injects human priors simultaneously. Extensive experiments demonstrate the effectiveness of EvoPose which achieves a new state of the art on two most popular benchmarks, Human3.6M and MPI-INF-3DHP.
MoreTranslated text
Key words
3D human pose estimation,Transformer,kinematic structure,recursive refinement
AI Read Science
Must-Reading Tree
Example
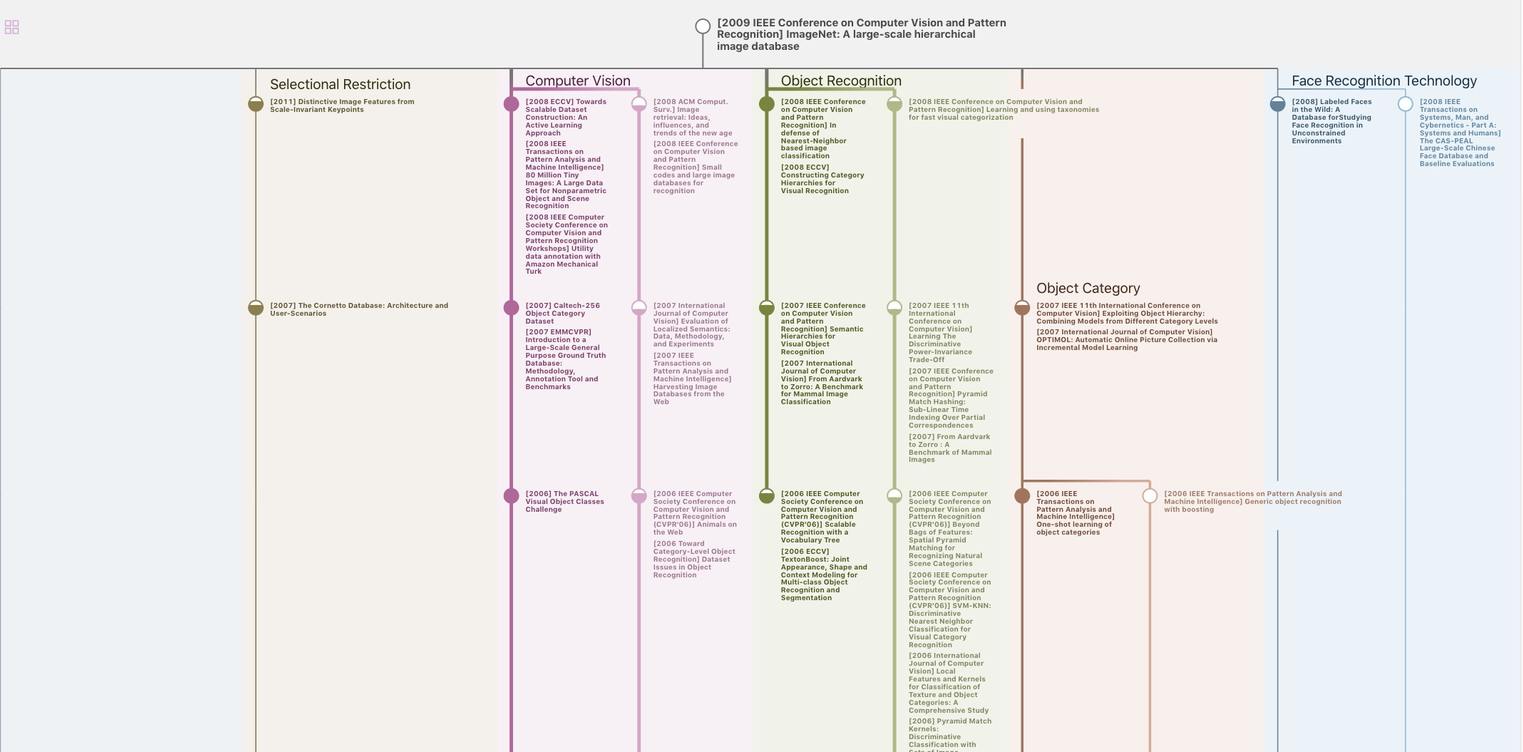
Generate MRT to find the research sequence of this paper
Chat Paper
Summary is being generated by the instructions you defined