Kokiri: Random-Forest-Based Comparison and Characterization of Cohorts
bioRxiv (Cold Spring Harbor Laboratory)(2022)
摘要
AbstractWe propose an interactive visual analytics approach to characterizing and comparing patient subgroups (i.e., cohorts). Despite having the same disease and similar demographic characteristics, patients respond differently to therapy. One reason for this is the vast number of variables in the genome that influence a patient’s outcome. Nevertheless, most existing tools do not offer effective means of identifying the attributes that differ most, or look at them in isolation and thus ignore combinatorial effects. To fill this gap, we present Kokiri, a visual analytics approach that aims to separate cohorts based on user-selected data, ranks attributes by their importance in distinguishing between cohorts, and visualizes cohort overlaps and separability. With our approach, users can additionally characterize the homogeneity and outliers of a cohort. To demonstrate the applicability of our approach, we integrated Kokiri into the Coral cohort analysis tool to compare and characterize lung cancer patient cohorts.
更多查看译文
关键词
cohorts,random-forest-based
AI 理解论文
溯源树
样例
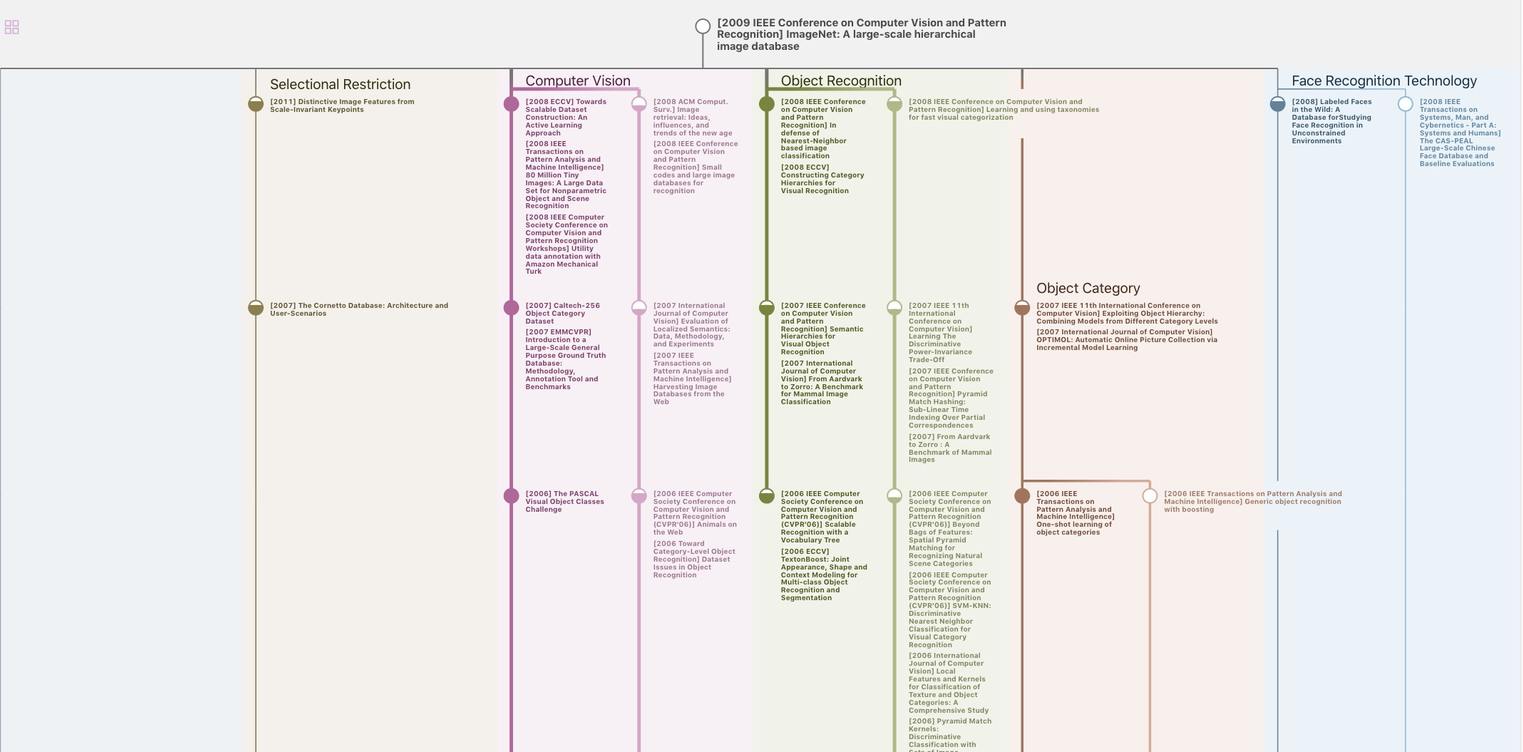
生成溯源树,研究论文发展脉络
Chat Paper
正在生成论文摘要