Improving Rare Tree Species Classification Using Domain Knowledge
IEEE Geosci. Remote. Sens. Lett.(2023)
摘要
Forest inventory forms the foundation of forest management. Remote sensing (RS) is an efficient means of measuring forest parameters at scale. Remotely sensed species classification can be used to estimate species abundances, distributions, and to better approximate metrics such as aboveground biomass. State-of-the-art methods of RS species classification rely on deep-learning models such as convolutional neural networks (CNNs). These models have two major drawbacks: they require large samples of each species to classify well and they lack explainability. Therefore, rare species are poorly classified causing poor approximations of their associated parameters. We show that the classification of rare species can be improved by as much as eight F1-points using a neuro-symbolic (NS) approach that combines CNNs with an NS framework. The framework allows for the incorporation of domain knowledge into the model through the use of mathematically represented rules, improving model explainability.
更多查看译文
关键词
Biological system modeling,Forestry,Data models,Vegetation,Mathematical models,Task analysis,Convolutional neural networks,Convolutional neural network (CNN),explainable machine learning,neuro-symbolics (NS),remote sensing (RS),tree species classification
AI 理解论文
溯源树
样例
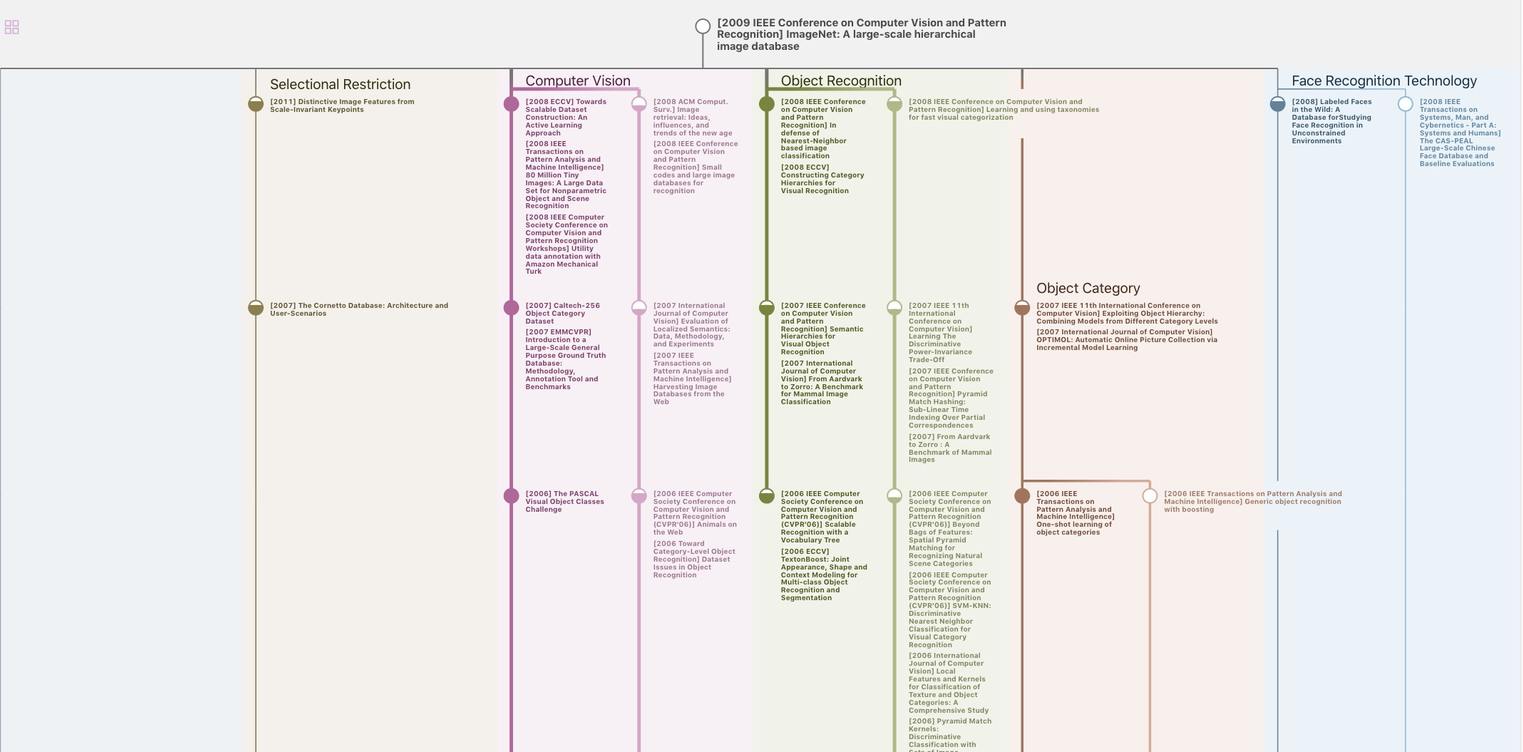
生成溯源树,研究论文发展脉络
Chat Paper
正在生成论文摘要