Investigation of Vehicular S-LSTM NOMA Over Time Selective Nakagami-m Fading with Imperfect CSI
Journal of Telecommunications and Information Technology(2022)
Abstract
In this paper, the performance of a deep learning based multiple-input multiple-output (MIMO) non-orthogonal multiple access (NOMA) system is investigated for 5G radio communication networks. We consider independent and identically distributed (i.i.d.) Nakagami-m fading links to prove that when using MIMO with the NOMA system, the outage probability (OP) and end-to-end symbol error rate (SER) improve, even in the presence of imperfect channel state information (CSI) and successive interference cancellation (SIC) errors. Further more, the stacked long short-term memory (S-LSTM) algorithm is employed to improve the system’s performance, even under time-selective channel conditions and in the presence of terminal’s mobility. For vehicular NOMA networks, OP, SER, and ergodic sum rate have been formulated. Simulations show that an S-LSTM-based DL-NOMA receiver outperforms least square (LS) and minimum mean square error (MMSE) receivers. Furthermore, it has been discovered that the performance of the end-to-end system degrades with the growing amount of node mobility, or if CSI knowledge remains poor. Simulated curves are in close agreement with the analytical results.
MoreTranslated text
AI Read Science
Must-Reading Tree
Example
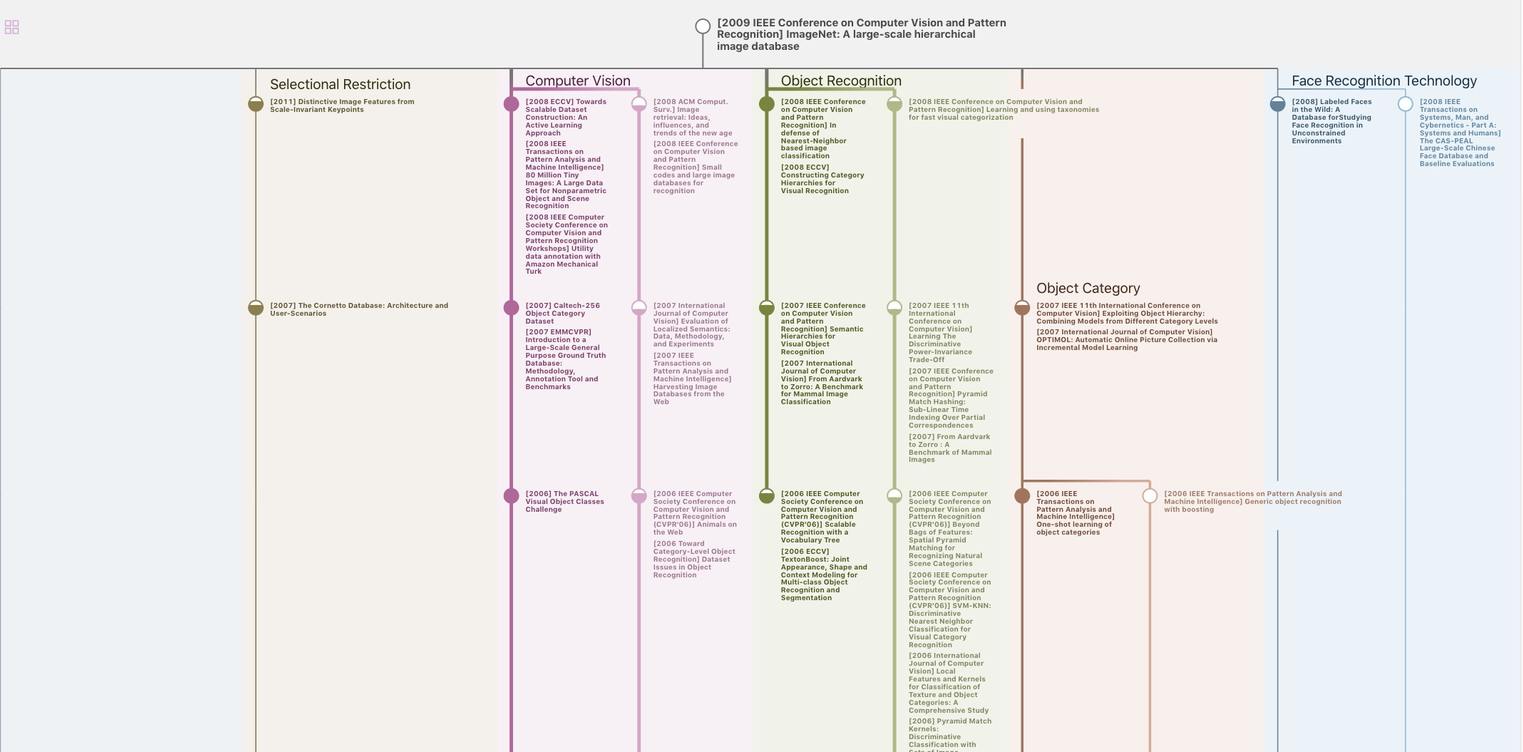
Generate MRT to find the research sequence of this paper
Chat Paper
Summary is being generated by the instructions you defined