Ground target classification using mmWave radar with bidirectional long short-term memory networks
Journal of Applied Remote Sensing(2022)
摘要
We propose an approach for millimeter wave radar targets classification based on the concatenated spectrogram and range-Doppler features. A publicly available dataset that contains raw single radar data for fast- and slow-walking people is utilized. First, the received channels are summed to obtain a higher signal-to-noise ratio. Next, range-Doppler and spectrogram plots are obtained after the two-dimensional fast Fourier transform of raw radar signals. Then the spectrogram dataset is normalized and augmented using a mixup algorithm and fed into the proposed encoder and decoder structures sequentially for the classification task. In addition to the bidirectional long short-term memory layer, dropout and batch normalization layers are added for regularization in the proposed network. Parameter optimization is done for each network for a certain number of parameters. Finally, the results show that with the proposed approach, a 0.961 mean F1 score is obtained, and it outperformed some state-of-the-art methods, such as convolutional neural networks (CNNs) and hybrid CNNs-long short-term memory networks. An ablation study of the proposed method is also presented. (c) 2022 Society of Photo-Optical Instrumentation Engineers (SPIE)
更多查看译文
关键词
mmWave radars, frequency modulated continuous wave radars, range-Doppler plots, spectrograms, time series classification
AI 理解论文
溯源树
样例
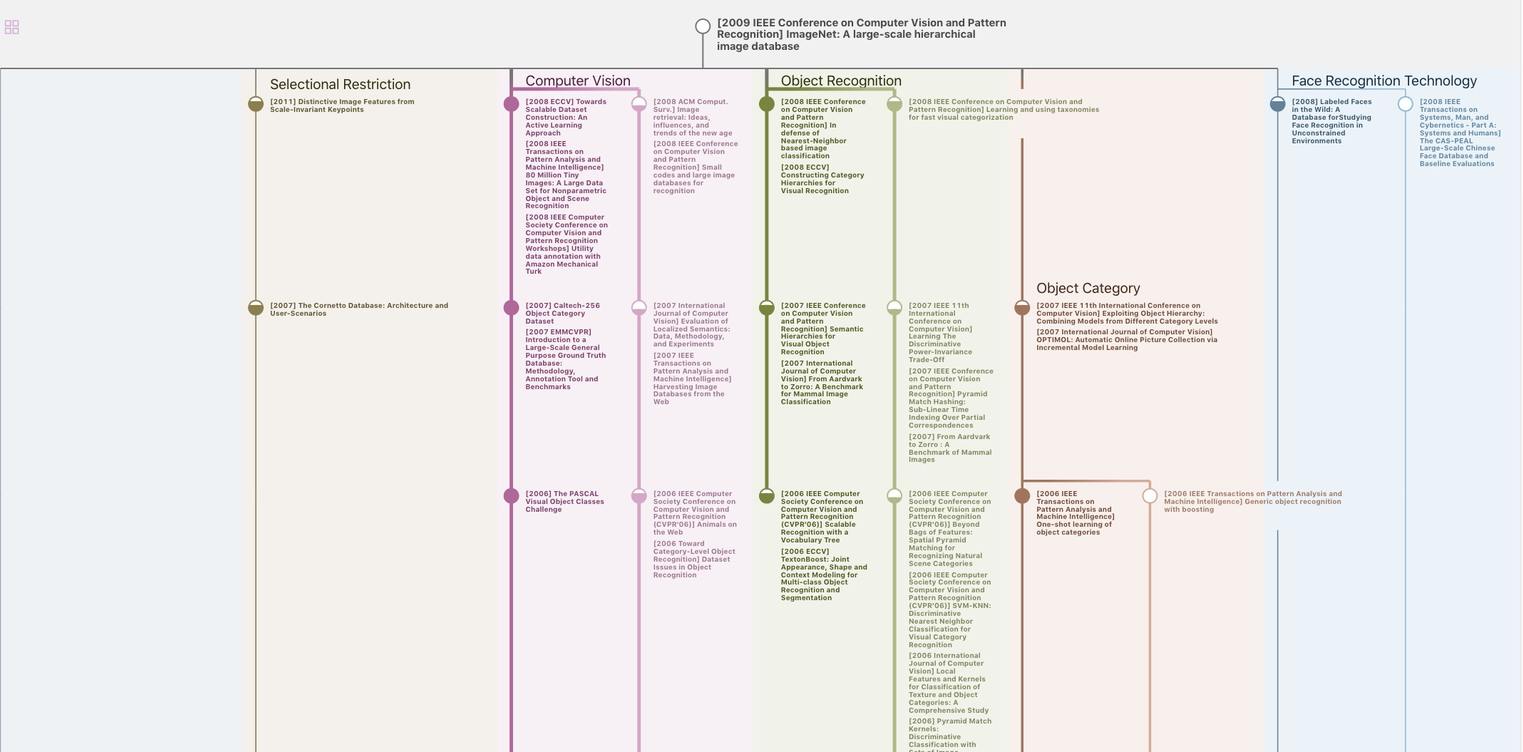
生成溯源树,研究论文发展脉络
Chat Paper
正在生成论文摘要