Multi-target Tracking with Random Finite Set in Decentralized Large-scale Sensor Networks
2022 IEEE International Conference on Unmanned Systems (ICUS)(2022)
摘要
This paper presents the multi-target tracking (MTT) problem in decentralized large-scale sensor networks. A novel random finite set (RFS)-based framework is designed, including an improved Probability Hypothesis Density filter with Gaussian Mixture (GM-PHD) representation, and an improved Generalized Covariance Intersection (GCI) fusion algorithm. For the local GM-PHD filter, the intensity of new-born targets is initialized by pre-segmenting the measurement set to address the uncertainty of measurement origin and the computational burden of large-scale measurements. Then, a sensor node-dependent clutter model is established to deal with the cardinality overestimation problem brought by the decentralized structure. For the proposed fusion algorithm, i.e., fusing the posterior PHDs generated by the proposed GM-PHD filters in a distributed manner, it is based on the idea of compensating the severe missed detection problem of GCI in the case of large-scale fusing resources by arithmetic average (AA) fusion. In this way, GCI and AA can be fully utilized to guarantee the estimation accuracy for common targets and, respectively, the robustness for exclusive targets. A simulation of a decentralized large-scale network demonstrates the effectiveness of the proposed framework with respect to estimation accuracy and computational cost.
更多查看译文
关键词
multi-target tracking,random finite set,probability hypothesis density,large-scale,sensor networks,distributed
AI 理解论文
溯源树
样例
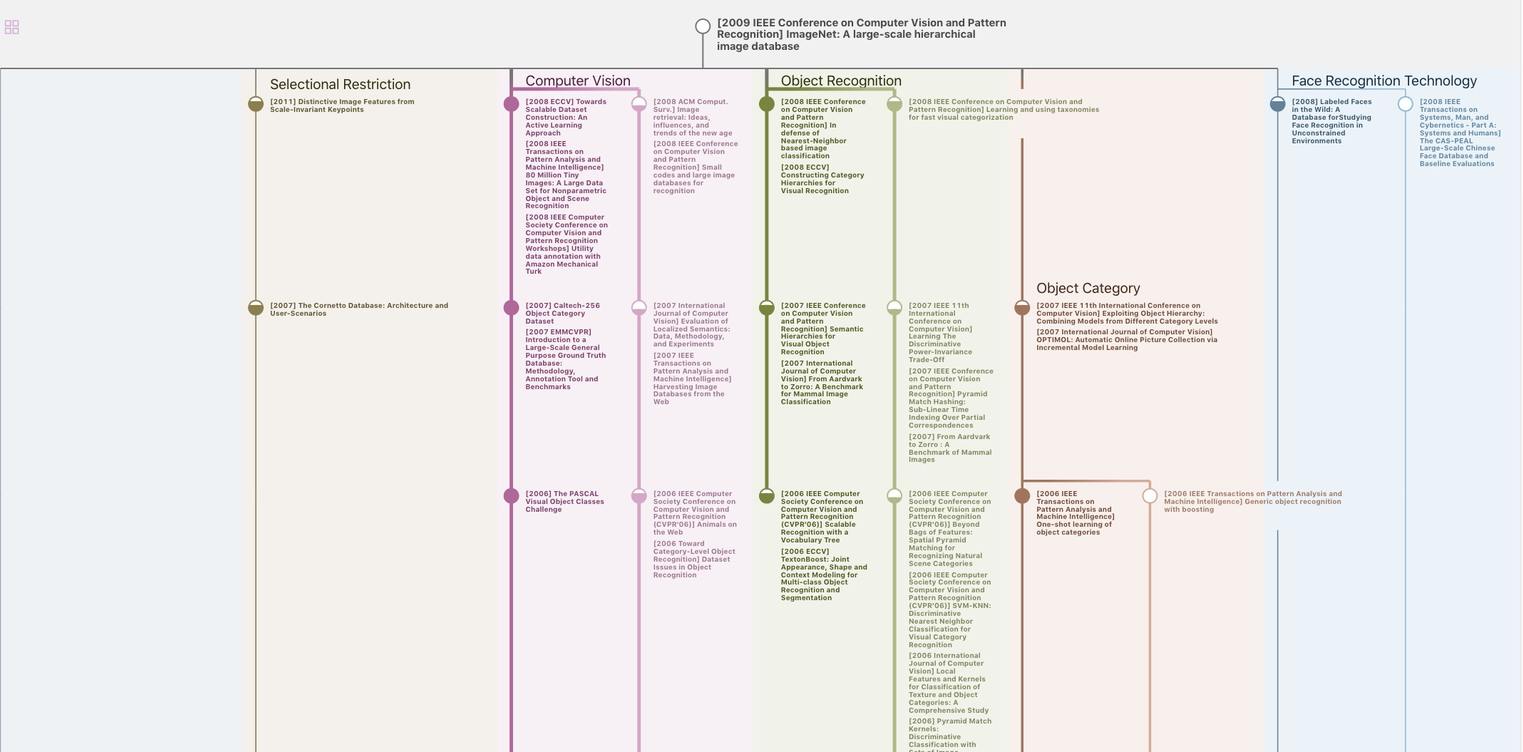
生成溯源树,研究论文发展脉络
Chat Paper
正在生成论文摘要