Direct Bayesian inference for fault severity assessment in Digital-Twin-Based fault diagnosis
SSRN Electronic Journal(2023)
摘要
For applications in condition-based maintenance of nuclear systems, the assessment of fault severity is crucial. In this work, we developed a framework that allows for direct inference of the probability distributions of possible faults in a system. We employed a model-based approach with model residuals generated from analytical redundancy relations provided by physics-based models of the system components. From real-time sensor readings, the values of the model residuals can be calculated, and the posterior probability distributions of the faults can be computed directly using the methods of Bayesian networks. From the posterior distribution of each fault, one can estimate the fault probability based on a chosen threshold and assess the severity of the fault. By eliminating the discretization and simplifications in middle steps, this approach allows us to leverage the available computational resources to provide more accurate fault probability estimates and severity assessments.
更多查看译文
关键词
Model-based diagnosis,Fault detection,Bayesian inference,Probabilistic programming,Digital twin
AI 理解论文
溯源树
样例
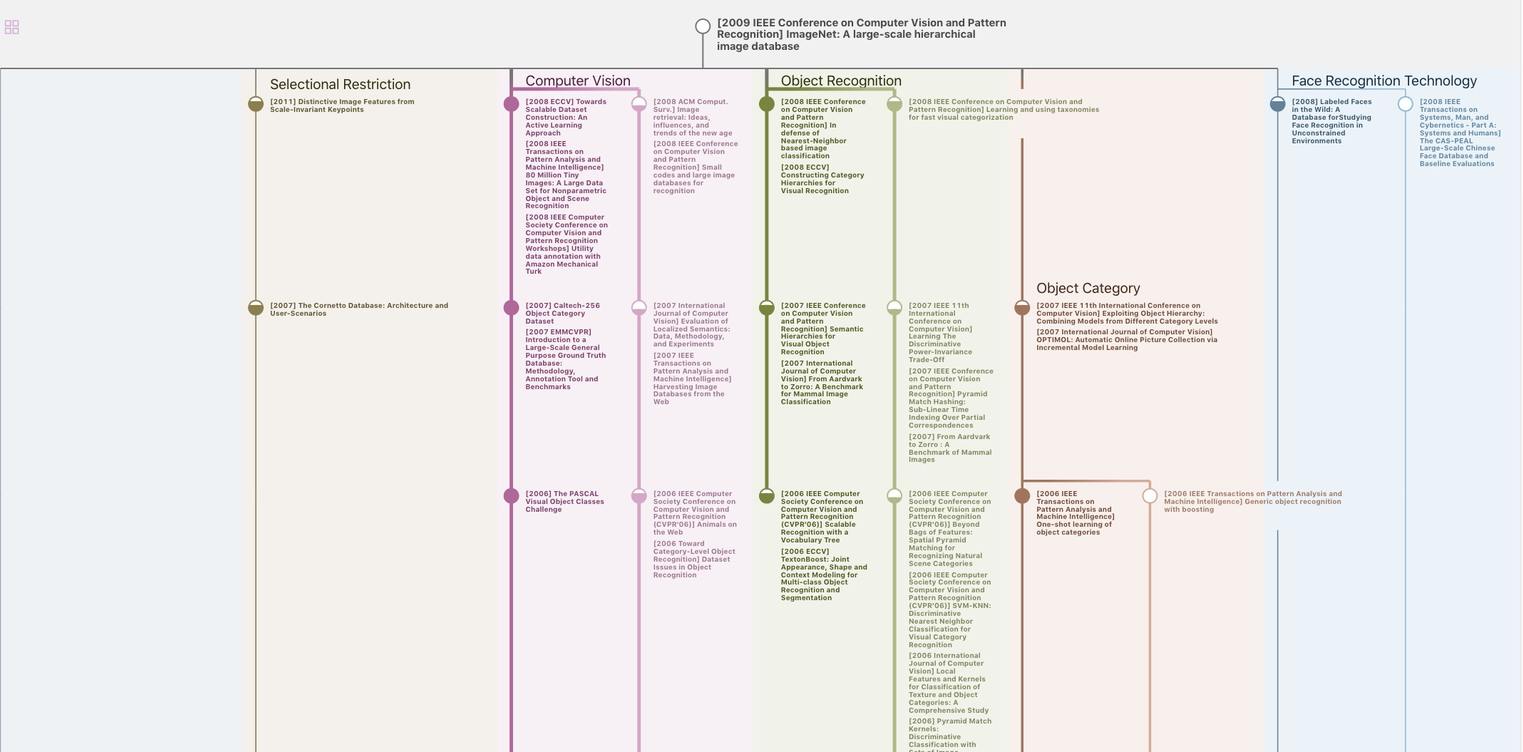
生成溯源树,研究论文发展脉络
Chat Paper
正在生成论文摘要