Automated MR Image Prescription of the Liver Using Deep Learning: Development, Evaluation, and Prospective Implementation
Journal of Magnetic Resonance Imaging(2022)
摘要
Background There is an unmet need for fully automated image prescription of the liver to enable efficient, reproducible MRI. Purpose To develop and evaluate artificial intelligence (AI)‐based liver image prescription. Study Type Prospective. Population A total of 570 female/469 male patients (age: 56 ± 17 years) with 72%/8%/20% assigned randomly for training/validation/testing; two female/four male healthy volunteers (age: 31 ± 6 years). Field Strength/Sequence 1.5 T, 3. 0 T ; spin echo, gradient echo, bSSFP . Assessment A total of 1039 three‐plane localizer acquisitions (26,929 slices) from consecutive clinical liver MRI examinations were retrieved retrospectively and annotated by six radiologists. The localizer images and manual annotations were used to train an object‐detection convolutional neural network (YOLOv3) to detect multiple object classes (liver, torso, and arms) across localizer image orientations and to output corresponding 2D bounding boxes. Whole‐liver image prescription in standard orientations was obtained based on these bounding boxes. 2D detection performance was evaluated on test datasets by calculating intersection over union (IoU) between manual and automated labeling. 3D prescription accuracy was calculated by measuring the boundary mismatch in each dimension and percentage of manual volume covered by AI prescription. The automated prescription was implemented on a 3 T MR system and evaluated prospectively on healthy volunteers. Statistical Tests Paired t ‐tests (threshold = 0.05) were conducted to evaluate significance of performance difference between trained networks. Results In 208 testing datasets, the proposed method with full network had excellent agreement with manual annotations, with median IoU > 0.91 (interquartile range < 0.09) across all seven classes. The automated 3D prescription was accurate, with shifts <2.3 cm in superior/inferior dimension for 3D axial prescription for 99.5% of test datasets, comparable to radiologists' interreader reproducibility. The full network had significantly superior performance than the tiny network for 3D axial prescription in patients. Automated prescription performed well across single‐shot fast spin‐echo, gradient‐echo, and balanced steady‐state free‐precession sequences in the prospective study. Data Conclusion AI‐based automated liver image prescription demonstrated promising performance across the patients, pathologies, and field strengths studied. Evidence Level 4. Technical Efficacy Stage 1.
更多查看译文
关键词
liver,image prescription,deep learning
AI 理解论文
溯源树
样例
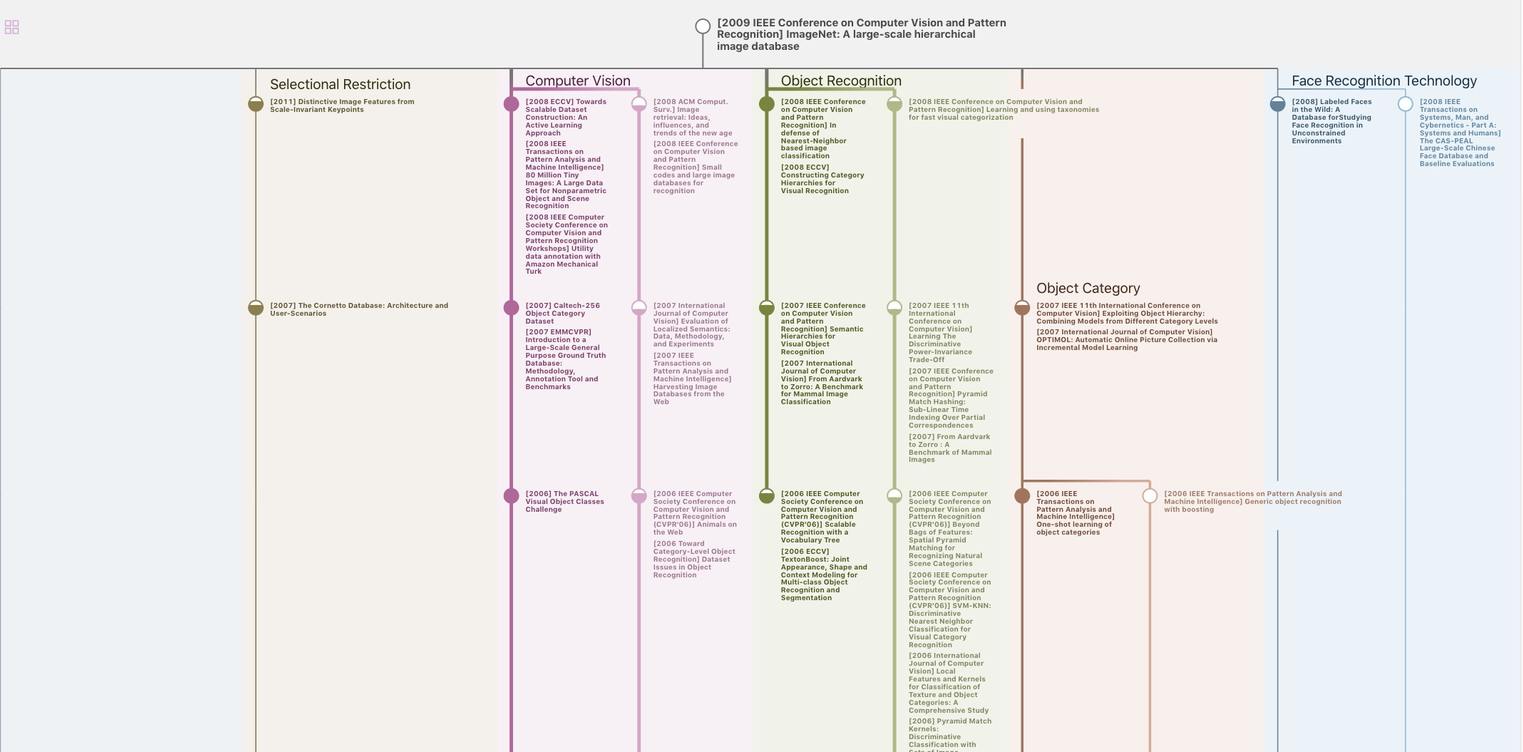
生成溯源树,研究论文发展脉络
Chat Paper
正在生成论文摘要