Few-shot partial multi-label learning with synthetic features network
Knowledge and Information Systems(2024)
摘要
In partial multi-label learning (PML) problems, each training sample is partially annotated with a candidate label set, among which only a subset of labels are valid. The major hardship for PML is that its training procedure is prone to be misled by false positive labels concealed in the candidate label set. To train a noise-robust multi-label predictor for PML problem, most existing methods hold the assumption that sufficient training samples are available. However, in actual fact, especially when dealing with new tasks, we more often only have a few PML samples for the target task. In this paper, we propose a unified model called FsPML-SF (Few-shot Partial Multi-Label Learning with Synthetic Features Network). FsPML-SF includes three modules: label disambiguation, data augmentation and classifier induction. Specifically, FsPML-SF attempts to update the label credibility of each PML sample by leveraging the feature and semantic similarities, the label credibility of other samples and label co-occurrence in a unified objective function. Next, FsPML-SF introduces a synthetic feature network to generate more training samples from pairs of given samples with corresponding label credibility values. FsPML-SF then utilizes the original and synthesized samples to induce a noise-tolerant multi-label classifier. We conducted extensive experiments on benchmark datasets, FsPML-SF outperforms recent competitive PML baselines and few-shot solutions. Both the label denoising and data augmentation improve the performance of PML on few-shot data.
更多查看译文
关键词
Partial Multi-label learning,Few-shot learning,Weakly-supervised learning,Noisy labels,Label correlations,Data augmentation
AI 理解论文
溯源树
样例
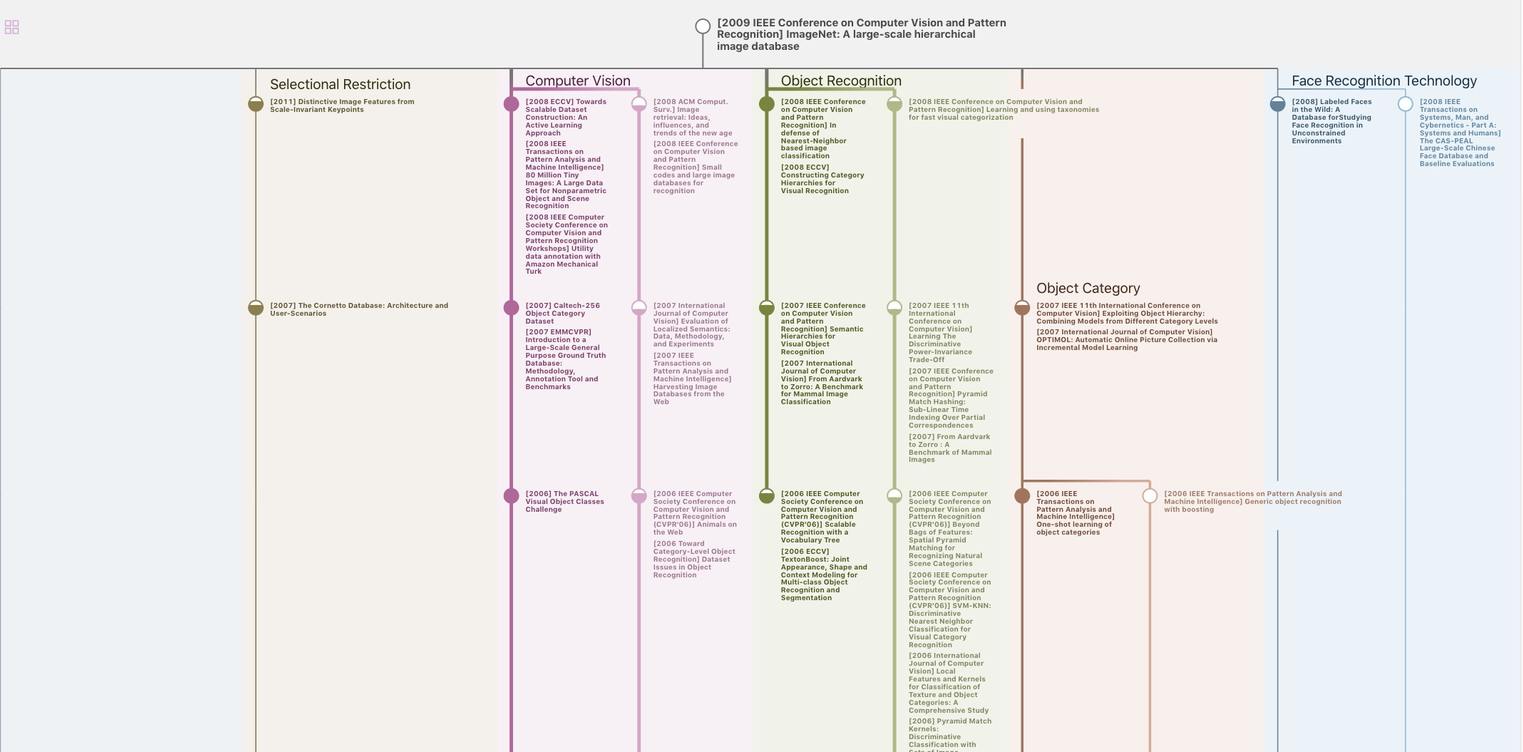
生成溯源树,研究论文发展脉络
Chat Paper
正在生成论文摘要