Slow Component Analysis Based Interictal-Preictal EEG Prediction
2022 E-Health and Bioengineering Conference (EHB)(2022)
摘要
An accurate and compact epileptic seizure prediction system would be significant to intractable patients as it can significantly improve the quality of their lives. This paper presents a novel approach for prediction of seizures in epileptic patients based on scalp electroencephalography (EEG) recordings. In this study, we explored meaningful underlying representation to derive latent predictive characteristics of EEG signals, at the same time make it possible to design a lightweight, compact solution for seizure prediction. This paper proposes a novel approach that explores the underlying representation of the signals by introducing Slow Component Analysis (SCA) for the differentiation. In addition, extracting several epileptiform-band signals from the original EEGs prior to the slowness analysis helps find the intrinsic change of the brain states. The performance of the proposed methodology was evaluated with the publicly available CHB-MIT dataset. This method achieved an accuracy of 94.41%, a sensitivity of 94.27%, a specificity of 95.61%. The experimental evaluation indicated that the proposed lightweight solution has acceptably satisfactory prediction performance.
更多查看译文
关键词
prediction,interictal-preictal
AI 理解论文
溯源树
样例
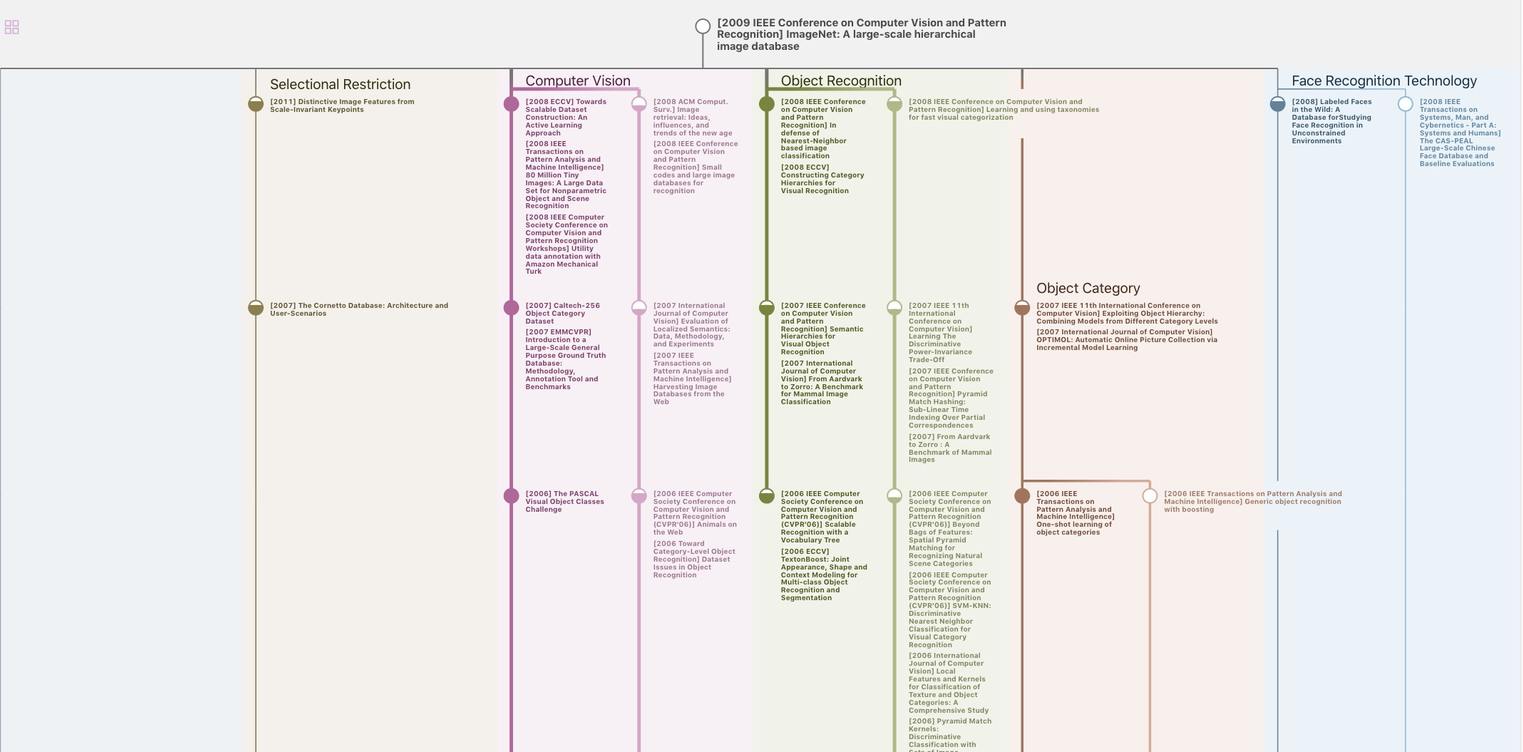
生成溯源树,研究论文发展脉络
Chat Paper
正在生成论文摘要