Model Selection for Deep Learning with Gradient Norms: An Empirical Study
Research Square (Research Square)(2023)
Abstract
Abstract Recent theoretical works [1–3] propose to exploit the loss gradient norm averaged across training samples as a measure of overfitting and generalization performance of DNNs, to investigate the upper bound of generalization error of deep neural networks (DNNs). However, the extremely high computational cost makes such gradient norms infeasible to obtain in practice. In this work, we carry out empirical studies by proposing a formulated mean empirical gradient norms (MEGAN), where we use a fast implementation of Fully-Connected (FC) Layer gradient norms [4] to compute MEGAN. Our empirical studies find that the sum of MEGAN over the optimization path of deep learning (i.e. epochs) could accurately predict the generalization performance of DNNs. Our empirical studies also include extensive experiments to demonstrate the potential of MEGAN for model selection of DNNs in hyper-parameter search settings. Our in-depth analyses interpret the behavior of MEGAN during training epochs and confirm MEGAN as an efficient and effective way to measuring the generalization performance of DNN with the training set only.
MoreTranslated text
Key words
deep learning,gradient norms,selection
AI Read Science
Must-Reading Tree
Example
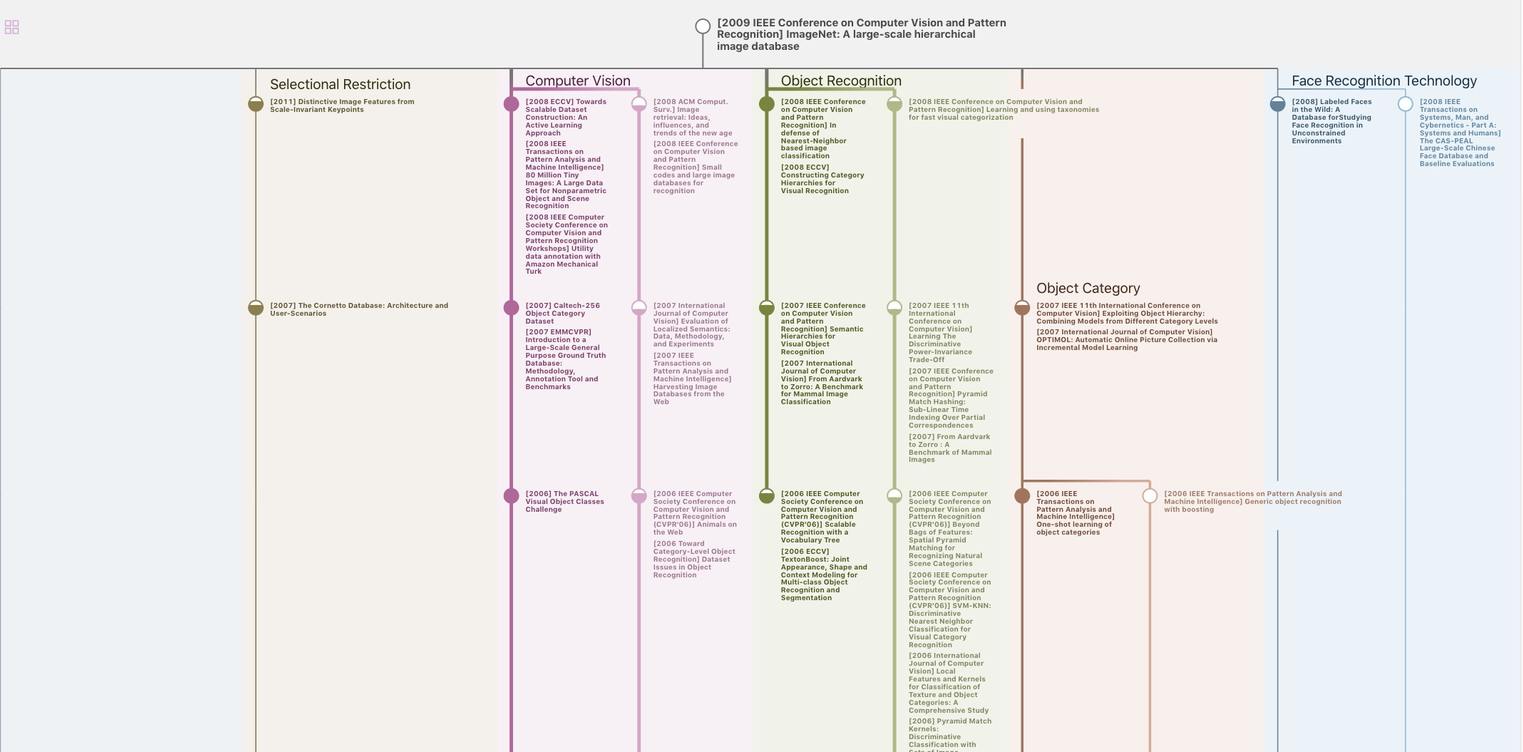
Generate MRT to find the research sequence of this paper
Chat Paper
Summary is being generated by the instructions you defined