Machine Learning-Based Improvement of Aerosol Optical Depth from CHIMERE Simulations using MODIS Satellite Observations
crossref(2022)
摘要
We develop a machine learning (ML) approach for improving the accuracy of the horizontal dis-tribution of the aerosol optical depth (AOD) simulated by the CHIMERE chemistry-transport model over Northern Africa using Moderate-Resolution Imaging Spectroradiometer (MODIS) AOD satellite observations. These observations are used during the training phase of the ML method for deriving a regional bias correction of AODs simulated by CHIMERE. The results are daily maps of regional bias corrected AODs with full horizontal coverage over Northern Africa. We test four types of ML models: multiple linear regression (MLR), random forests (RF), gradi-ent boosting (XGB), and multiple layer perceptron networks (NN). We perform comparisons with satellite and independent ground-based observations of AOD that are not used in the training phase. They suggest that all models have overall comparable performances with a slight advantage of the RF model which expresses less spatial artifacts. While the method slightly underestimates the very high AODs, it significantly reduces biases and absolute errors, and clearly enhances linear correlations with respect to independent observations. This im-provement for deriving the AOD is particularly relevant for high dust pollution regions like the Sahara Desert, which dramatically lack ground-based measurements for validations of chemis-try-transport modeling which currently remains challenging and imprecise.
更多查看译文
AI 理解论文
溯源树
样例
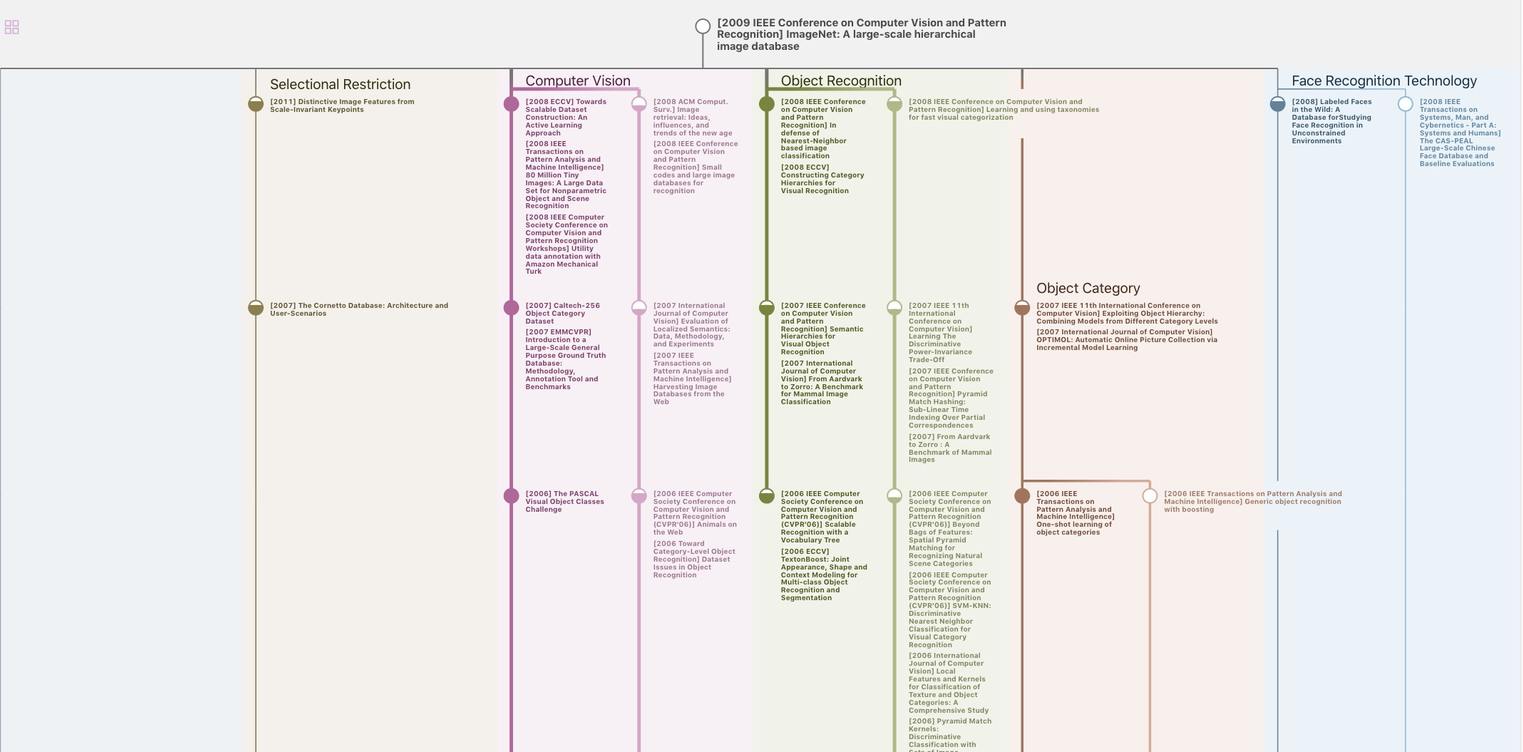
生成溯源树,研究论文发展脉络
Chat Paper
正在生成论文摘要