TRUNet: Transformer-Recurrent-U Network for Multi-channel Reverberant Sound Source Separation
Interspeech 2022(2022)
摘要
In recent years, many deep learning techniques for single-channel sound source separation have been proposed using recurrent, convolutional and transformer networks. When multiple microphones are available, spatial diversity between speakers and background noise in addition to spectro-temporal diversity can be exploited by using multi-channel filters for sound source separation. Aiming at end-to-end multi-channel source separation, in this paper we propose a transformer-recurrent-U network (TRUNet), which directly estimates multi-channel filters from multi-channel input spectra. TRUNet consists of a spatial processing network with an attention mechanism across microphone channels aiming at capturing the spatial diversity, and a spectro-temporal processing network aiming at capturing spectral and temporal diversities. In addition to multi-channel filters, we also consider estimating single-channel filters from multi-channel input spectra using TRUNet. We train the network on a large reverberant dataset using a combined compressed mean-squared error loss function, which further improves the sound separation performance. We evaluate the network on a realistic and challenging reverberant dataset, generated from measured room impulse responses of an actual microphone array. The experimental results on realistic reverberant sound source separation show that the proposed TRUNet outperforms state-of-the-art single-channel and multi-channel source separation methods.
更多查看译文
关键词
separation,transformer-recurrent-u,multi-channel
AI 理解论文
溯源树
样例
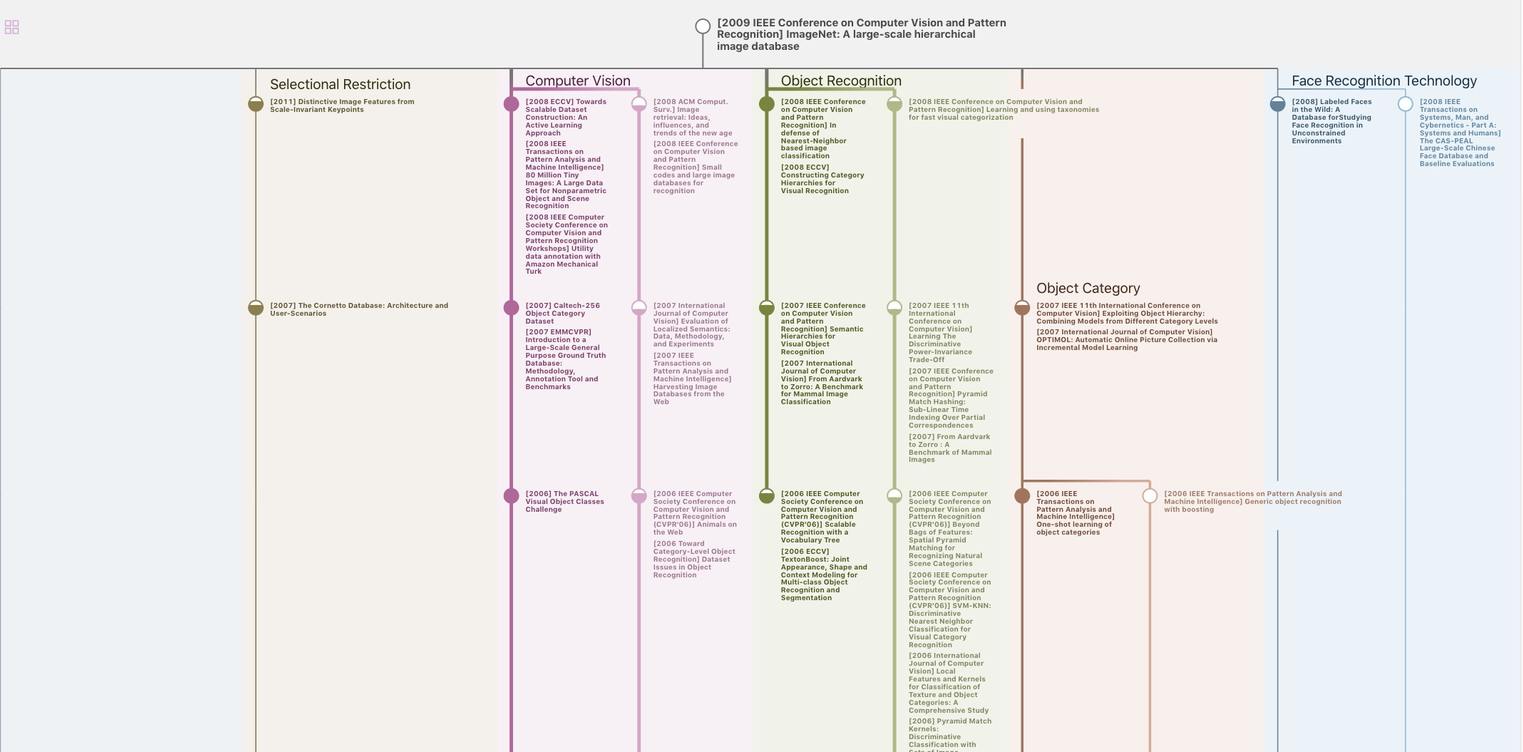
生成溯源树,研究论文发展脉络
Chat Paper
正在生成论文摘要