Error-based updating of event representations enables prediction of human activity at human scale
crossref(2022)
摘要
Humans form sequences of event models—representations of the immediate situation—topredict how activity will unfold. Two challenging questions about event models are “How isactivity segmented into events?” and “How is knowledge about different event types learned andrepresented?” We constructed a computational model combining a recurrent neural network forshort-term dynamics with Bayesian inference over event types for event-to-event transitions.This architecture learns schemas representing knowledge about event types and uses them,along with observed perceptual information, to construct a series of event models, updatingthem when predictions are violated. The architecture learned to predict activity dynamics fromone pass through an 18-hour corpus of naturalistic human activity. When tested on another 3.5hours of activities, it switched schemas at times corresponding with human segmentation andformed human-like event categories—despite being given no feedback about segmentation orcategorization. These results establish that event schemas and updating based on predictionerror can ground learning and can naturally reproduce two important features of humancomprehension.
更多查看译文
AI 理解论文
溯源树
样例
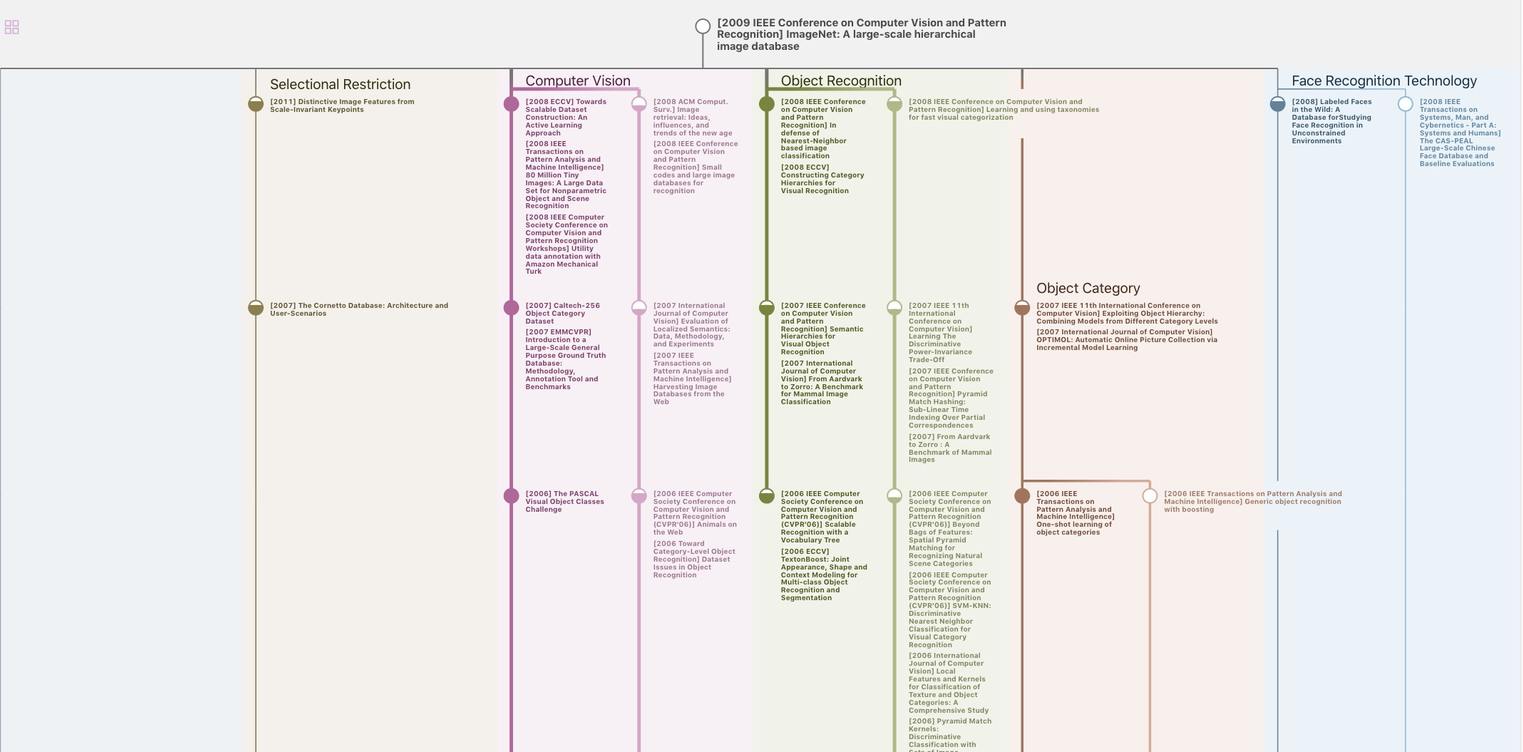
生成溯源树,研究论文发展脉络
Chat Paper
正在生成论文摘要