SemanticAnchors: Sequential Fusion using Lidar Point Cloud and Anchors with Semantic Annotations for 3D Object Detection
2022 IEEE 17th Conference on Industrial Electronics and Applications (ICIEA)(2022)
Abstract
3D object detection is an important task in autonomous driving scenario, which is the basis of perception and understanding of 3D scenes. LiDAR and camera are two conunonly used sensors in 3D object detection tasks. However, using a single sensor to collect data will make some objects difficult to detect because both sensors have insurmountable shortcomings Unexpectedly, LiDAR-only detection methods tend to show better performance than the multi -sensor methods in public benchmarks. This shows that people need to further explore the methods of combining the data of the two sensors. Recently, PointPainting has been presented to combine the data of LiDAR and camera more efficiently by attaching the result of image semantic segmentation to point cloud data as new channels. In this paper, we propose an error anchor punishment mechanism based on image semantic segmentation results. After the semantic augmentation of the point cloud data, we judge whether the semantic result of each point is correct by traversing the groundtruth boxes. Further, we assign different weights to each anchor according to the error points contained in each anchor. Experimental results on the KITTI valid set show that SemanticAnchors achieves better performance in both 3D and birds eyes view benchmarks. In particular, our method adds little extra computation and achieves performance improvement in all categories.
MoreTranslated text
Key words
3D object detection,autonomous driving,multi sensor
AI Read Science
Must-Reading Tree
Example
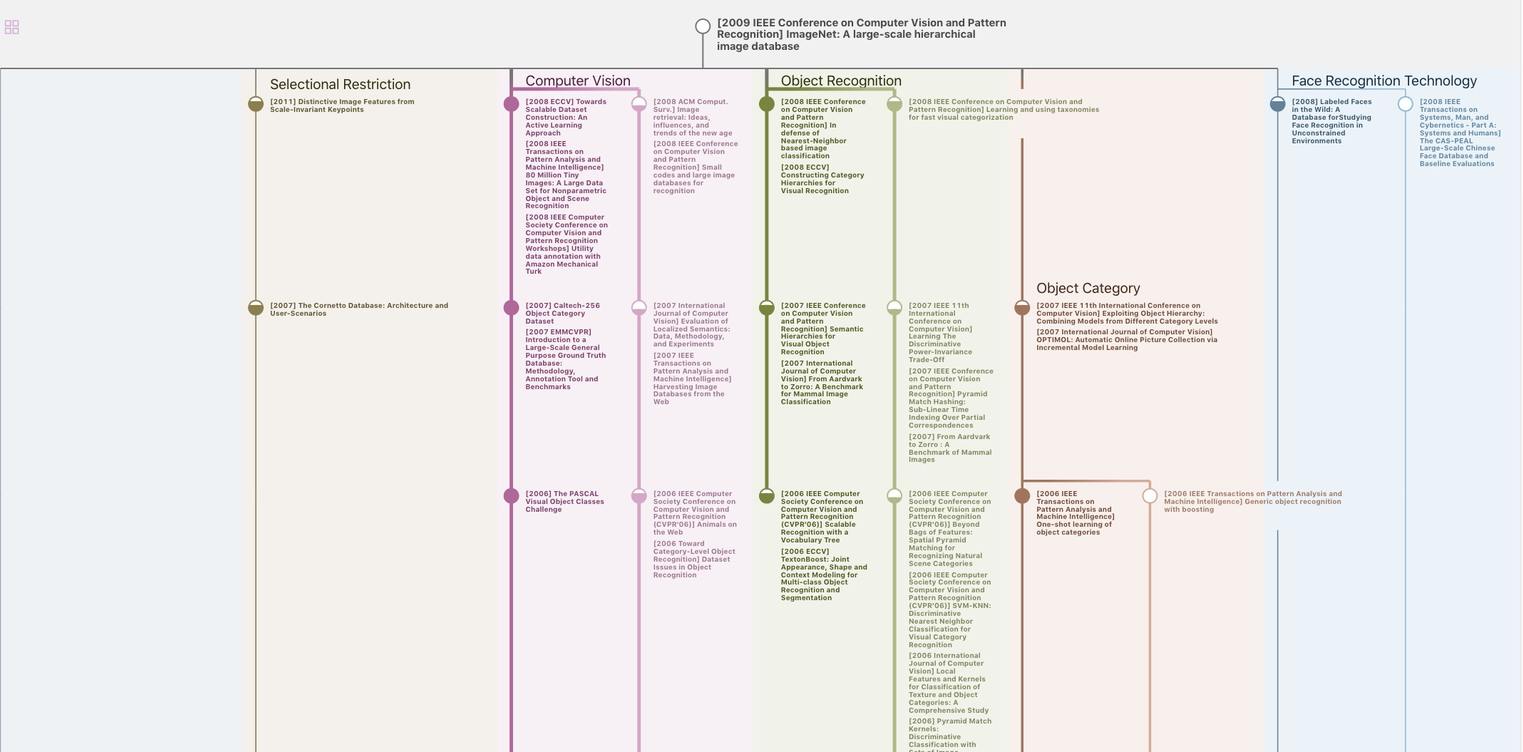
Generate MRT to find the research sequence of this paper
Chat Paper
Summary is being generated by the instructions you defined