Performance Evaluation of LIBSVM and MSVM in Human Age Estimation and Gender Identification from Digital Images of Femur bone
crossref(2022)
Abstract
Abstract In forensic science, identification of age and gender from the skeletal remains is a time-consuming and challenging task. It is known that bones in the human skeleton develop from several separate centers of ossification. It is challenging to determine the gender and the age of fatalities in mass disasters, aviation crashes, wildfire victims, exhumations, and bomb explosions. Therefore, the use of computer-assisted techniques is necessary and important in medical image analysis since they enable forensic science to produce results quickly. Manual assessment techniques based on a visual inspection of the bones reveal their incapacity for precise classification. Long bone plays an important role in medical and legal investigations since they resist environmental impacts. Hence, digital x-ray images of the femur bone are considered as input for the experiment. In this paper, the major contributing features of the femur are identified which will facilitate determining the age and gender of a person. We have developed a classification system using SVM Classifiers. For age estimation, the Multiclass SVM (MSVM) classifier is used, and gender determination uses the LIBSVM classifier. As per the medical literature, the average neck-shaft angle is 132° for men and 121° for women. The average head diameter is 46 mm for men and 39 mm for women. Males' femur neck angles can range from 122° to 149°, while females can range from 113° to 141°. Males' head diameters can range from 33.6 mm to 51.9 mm, while female head diameters can range from 31.4 mm to 46.7 mm. From the experimental results, the age estimation and gender identification are above 90% using Multiclass SVM (MSVM) and LIBSVM respectively. The findings of the current study reveal that there are differences in statistical significance between male and female femur radiograph measurements, with male feature values marginally predominating over female feature values in most of the samples.
MoreTranslated text
AI Read Science
Must-Reading Tree
Example
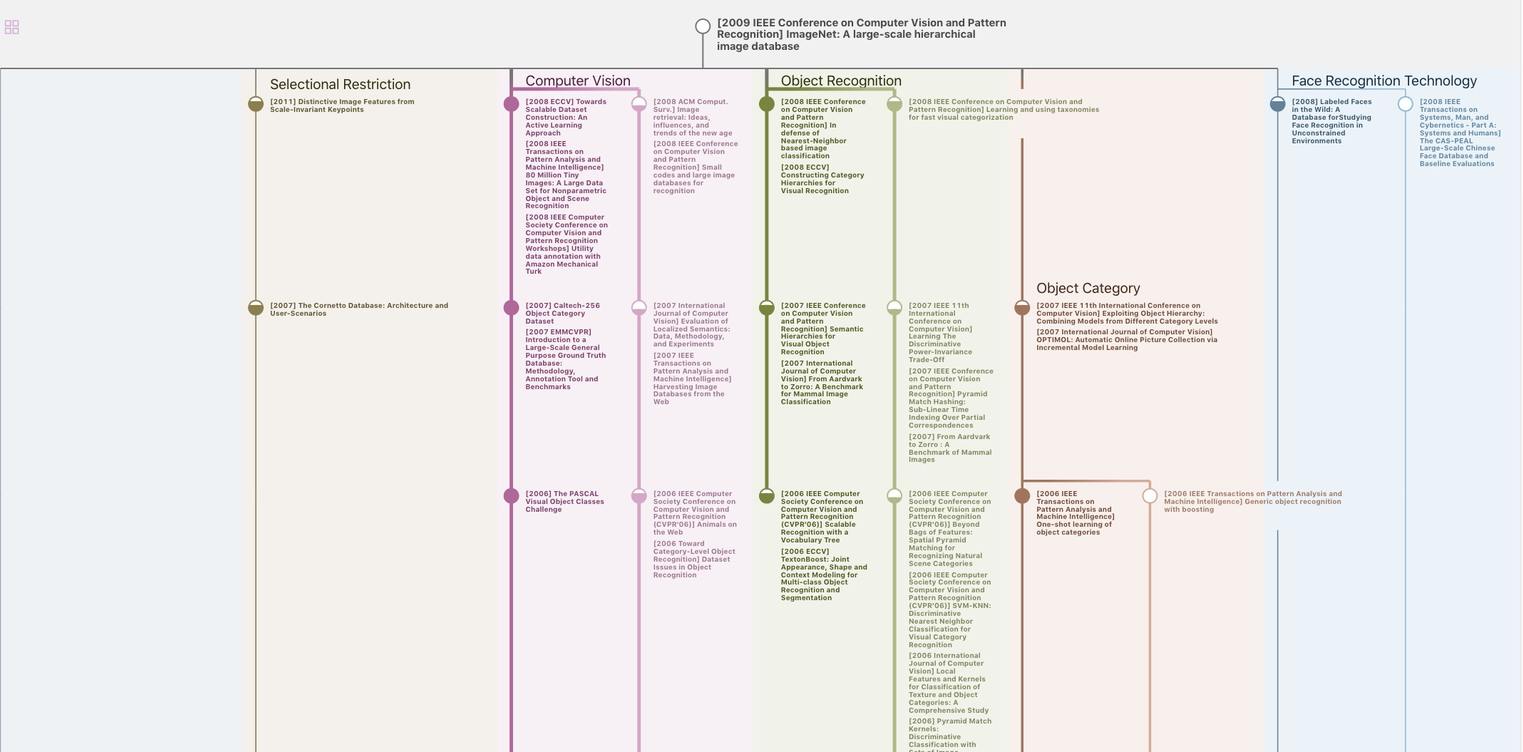
Generate MRT to find the research sequence of this paper
Chat Paper
Summary is being generated by the instructions you defined