On the benefits of automated tuning of hyper-parameters: an experiment related to temperature prediction on UAV computers
Anais do XIX Encontro Nacional de Inteligência Artificial e Computacional (ENIAC 2022)(2022)
摘要
Finding the best configuration of a neural network to solve a problem has been challenging given the numerous possibilities of values of the hyper-parameters. Thus, tuning of hyper-parameters is one important approach and researchers suggest doing this automatically. However, it is important to verify when it is suitable to perform automated tuning which is usually very costly financially and also in terms of hardware infrastructure. In this study, we analyze the advantages of using a hyper-parameter optimization framework as a way of optimizing the automated search for hyper-parameters of a neural network. To achieve this goal, we used data from an experiment related to temperature prediction of computers embedded in unmanned aerial vehicles (UAVs), and the models Long Short-Term Memory (LSTM) and Gated Recurrent Unit (GRU) to perform these predictions. In addition, we compare the hyper-parameter optimization framework to the hyper-parameter exhaustive search technique varying the size of the training dataset. Results of our experiment shows that designing a model using a hyper-parameter optimizer can be up to 36.02% better than using exhaustive search, in addition to achieving satisfactory results with a reduced dataset.
更多查看译文
AI 理解论文
溯源树
样例
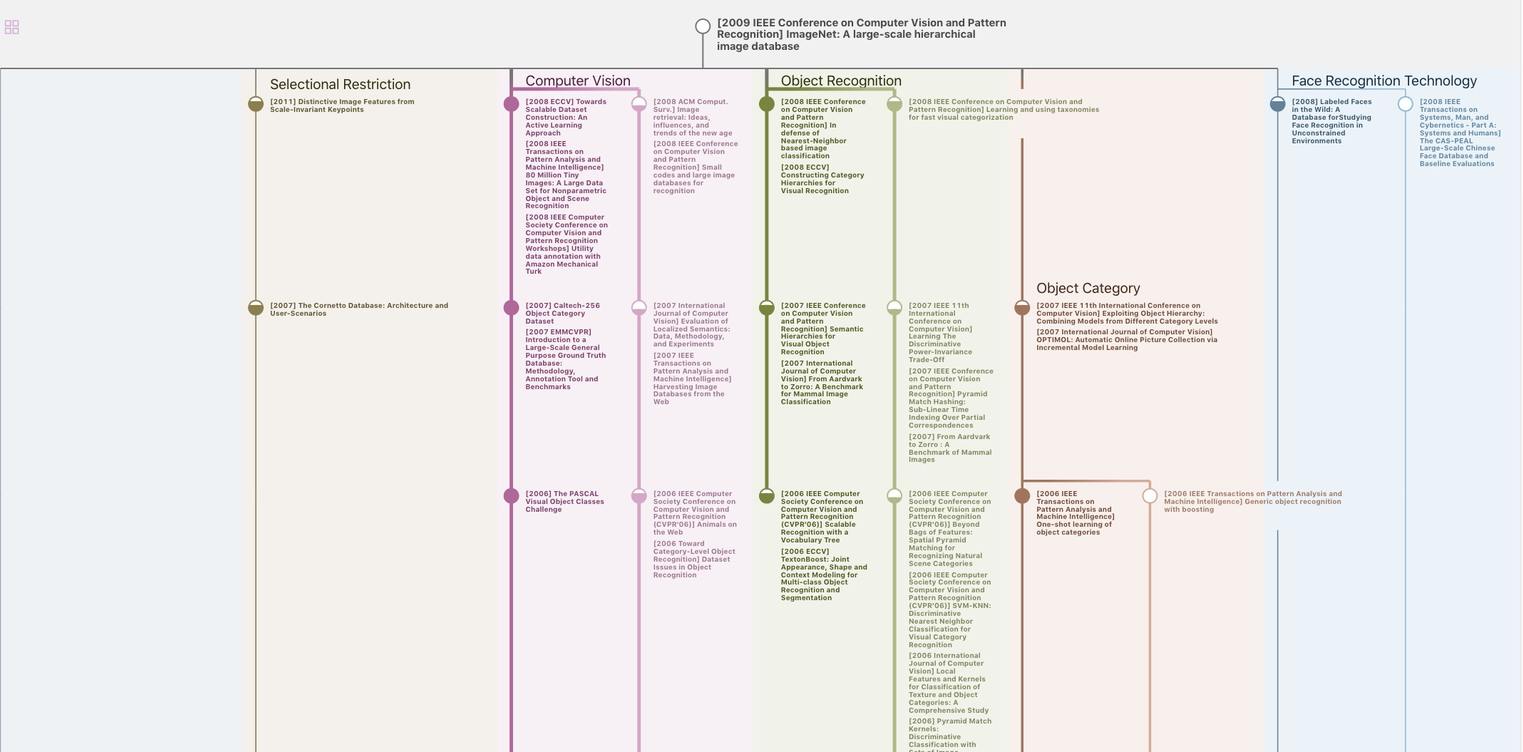
生成溯源树,研究论文发展脉络
Chat Paper
正在生成论文摘要