Generating X-ray Reports Using Global Attention
Anais do XIX Encontro Nacional de Inteligência Artificial e Computacional (ENIAC 2022)(2022)
摘要
The use of images for the diagnosis, treatment, and decision-making in health is frequent. A large part of the radiologist’s work is the interpretation and production of potentially diagnostic reports. However, they are professionals with high workloads doing tasks operator dependent, that is being subject to errors in case of non-ideal conditions. With the COVID-19 pandemic, healthcare systems were overwhelmed, extending to the X-ray analysis process. In this way, the automatic generation of reports can help to reduce the workload of radiologists and define the diagnosis and treatment of patients with suspected COVID-19. In this article, we propose to generate suggestions for chest radiography reports evaluating two architectures based on: (i) Long short-term memory (LSTM), and (ii) LSTM with global attention. The extraction of the most representative features from the X-ray images is performed by an encoder based on a pre-trained DenseNet121 network for the ChestX-ray14 dataset. Experimental results in a private set of 6650 images and reports indicate that the LSTM model with global attention yields the best result, with a BLEU-1 of 0.693, BLEU-2 of 0.496, BLEU-3 of 0.400, and BLEU-4 of 0.345. The quantitative and qualitative results demonstrate that our method can effectively suggest high-quality radiological findings and demonstrate the possibility of using our methodology as a tool to assist radiologists in chest X-ray analysis.
更多查看译文
AI 理解论文
溯源树
样例
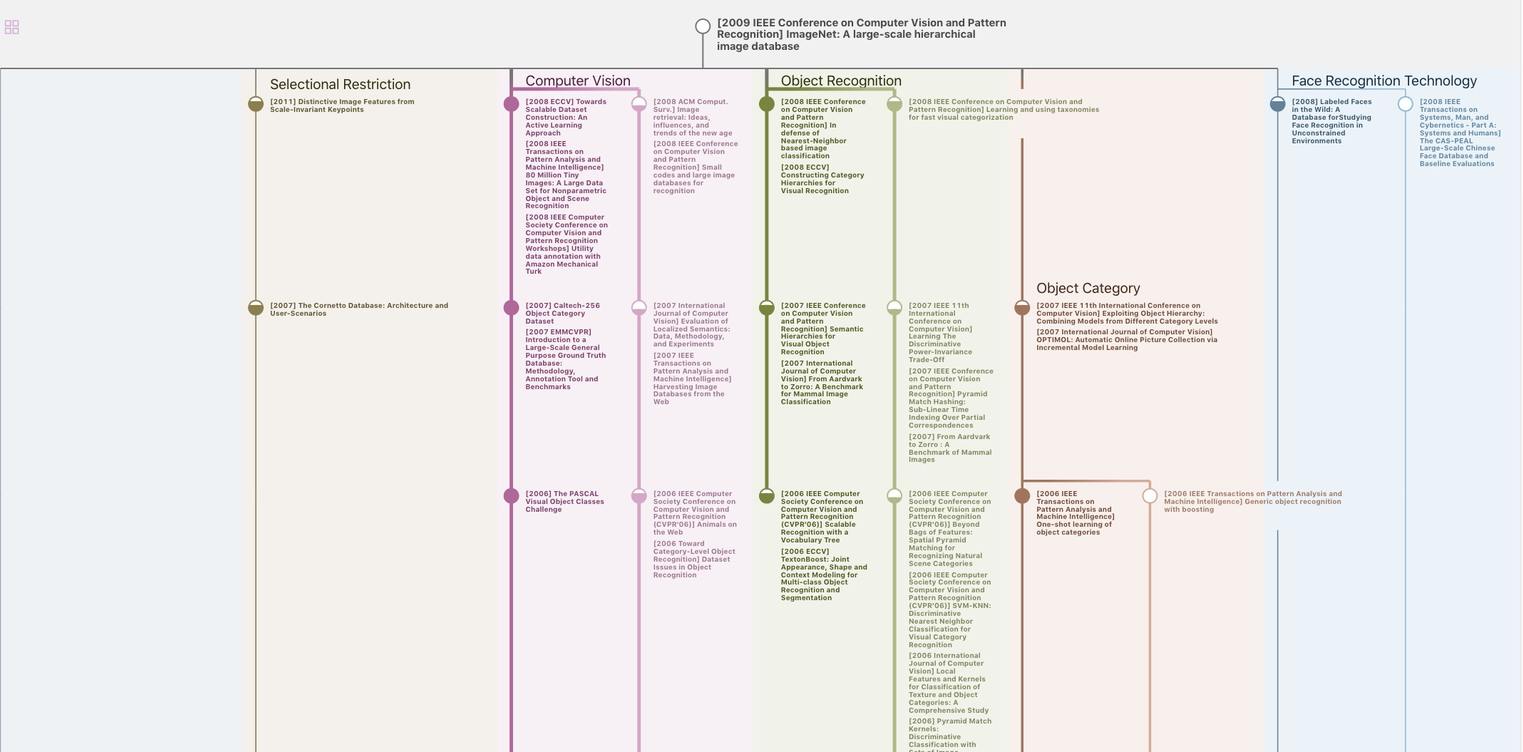
生成溯源树,研究论文发展脉络
Chat Paper
正在生成论文摘要