Measurement Error Correction for Ambient PM2.5 Exposure Using Stratified Regression Calibration: Effects on All-Cause Mortality
ISEE Conference Abstracts(2022)
摘要
Background and Aim We define measurement error as the difference between actual and predicted PM2.5 concentrations near subjects’ home. Previous studies on the impact of measurement error for PM2.5 were mostly simulation studies or used single regression calibration model to correct for measurement error. However, the relationship between actual and error-prone PM2.5 concentration may vary by time and region. Here we aim to correct the measurement error of PM2.5 predictions using stratified regression calibration and investigate how the measurement error biases the association between PM2.5 and mortality. Methods The "gold-standard” measurements of PM2.5 were daily monitoring data from 2,156 sites across the United States between 2000-2016. The error-prone measure was PM2.5 estimated by ensemble machine learning models. PM2.5 data was stratified by seasons, elevation and census divisions. We regressed daily monitoring PM2.5 on modeled PM2.5 from the same location and time using model below by strata. E(PM2.5monitoring)= β0j+ β1j PM2.5modeled+εj Calibrated PM2.5 was calculated with stratum-specific calibration parameters β0j and β1j for each ZIP code and season and aggregated to annual level. Association between calibrated and error-prone annual PM2.5 and all-cause mortality were estimated using mortality data from Medicare beneficiaries with Quasi-Poisson regression models adjusting for demographics, socioeconomic status, access to healthcare, population density and meteorologic factors. Results Across 72 strata, the median of β0 and β1 were 0.40 (25% 0.06, 75% 0.95) and 0.93 (25% 0.83, 75% 0.98). From calibrated and error-prone PM2.5 data, we estimated that each 10µg/m3 increase in PM2.5 was associated with 12.2% (95%CI 11.9-12.4) and 11.6% (95%CI 11.4-11.9) increase in the probability of death among Medicare beneficiaries, conditional on confounders. Conclusions Regression calibration parameters of PM2.5 varied by season and region. Using error-prone measure of PM2.5 underestimated the association between PM2.5 and all-cause mortality. Modern exposure models produce relatively small bias. Keywords PM2.5; measurement error
更多查看译文
关键词
stratified regression calibration,exposure,mortality,all-cause
AI 理解论文
溯源树
样例
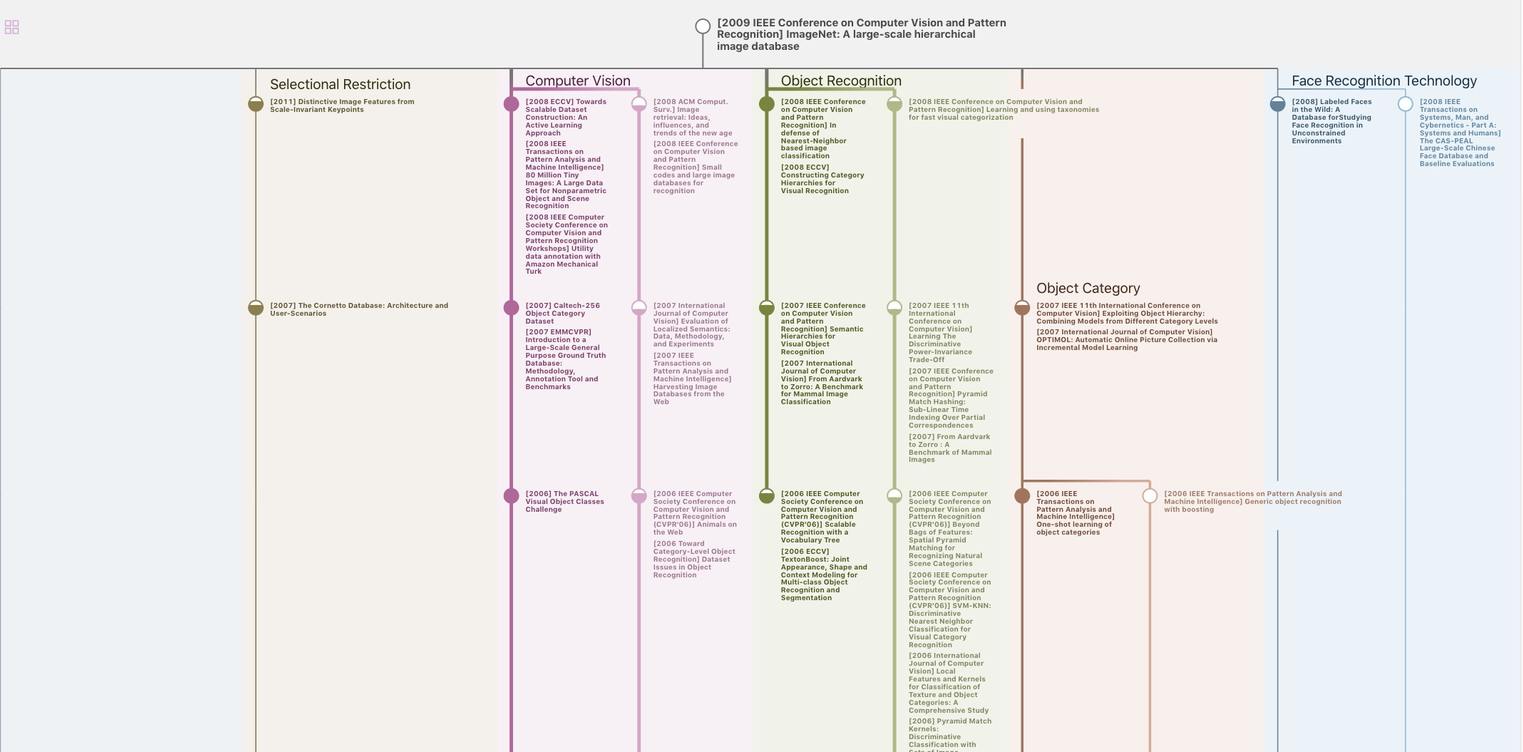
生成溯源树,研究论文发展脉络
Chat Paper
正在生成论文摘要