Capture of local sources variation using a hierarchical Bayesian spatiotemporal model for the spatial prediction of air pollution levels
ISEE Conference Abstracts(2022)
摘要
Background and aim The spatial predictions obtained from models based on land use regression (LUR), even the most advanced ones, do not fully capture local variations in the levels of air pollutants. This is because they do not control for unobserved variation, including spatial and temporal dependencies. Our objective is to present a hierarchical Bayesian spatiotemporal model for the spatial prediction of air pollution levels that, considering unobserved variation, both spatial heterogeneity and spatio-temporal dependence, controls for fine scale spatial variability. Methods We use information on the hourly levels of air pollution for 2018-2019 from 116 monitoring stations located throughout Catalonia, Spain. The pollutants we are interested in for making spatial predictions are PM10, PM2.5, NO2 and O3. We carried out the spatial predictions at a point level. We specified a hierarchical spatiotemporal model using the Stochastic Partial Differential Equations of the integrated nested Laplace approximation. As predictors we use the levels of other air pollutants, both measured at the monitoring stations and high-resolution satellite and reanalysis data, and land use variables (altitude of the monitoring station, NDVI, land cover, density). We control for unobserved variation, both spatial heterogeneity (using unstructured random effects) and spatial and temporal dependence (using structured random effects), considering them non-independent but separable. Results As preliminary results, the predictions using our model are much better than using an advanced LUR model. The mean absolute percentage error (MAPE, in %) and the root mean squared prediction errors (RMSPE, μg/m3) of our model are: PM10: 7.55-2.62 (MAPE-RMSPE), PM2.5: 9.99-4.50, NO2: 4.37-2.14; and O3: 6.83-2.78, while those of the LUR models are: 10.66-5.26, 15.99-5.61, 13.090-2.181, 33.21-13.85, respectively. Conclusions By controlling for unobserved variation, both heterogeneity and space-time dependency, our model can capture variation at the fine spatial scale and thus achieve more accurate spatial predictions.
更多查看译文
关键词
hierarchical bayesian spatiotemporal model,spatial prediction,local sources variation
AI 理解论文
溯源树
样例
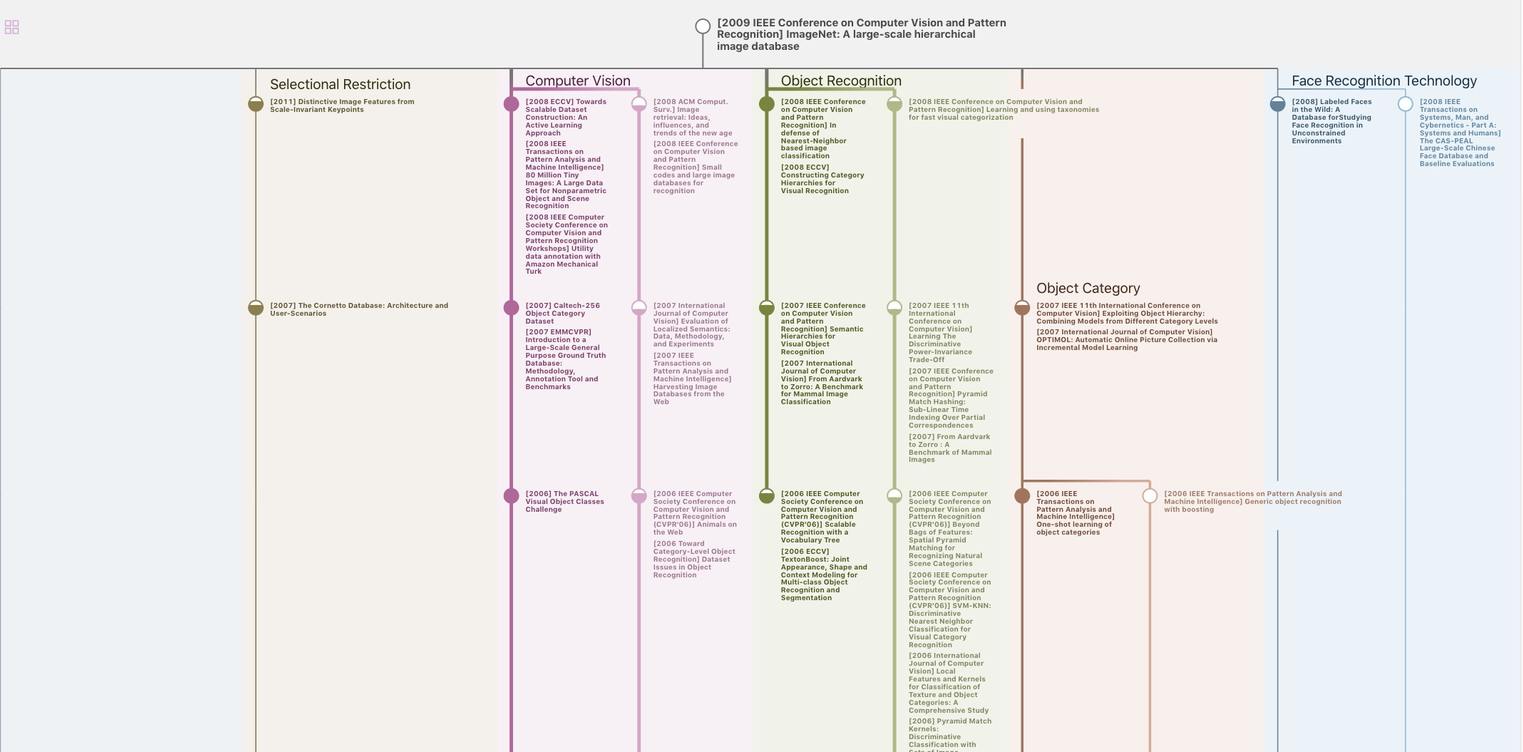
生成溯源树,研究论文发展脉络
Chat Paper
正在生成论文摘要