Pharmacophore-based virtual screening of large compound databases can aid “big data” problems in drug discovery
Elsevier eBooks(2023)
Abstract
From available billion drug-like compounds for targeting approximately 105 human proteins, search for a lead compound against a disease-specific target is obviously a challenging task for high-throughput screens (HTS). In silico virtual screening of large compound databases serve complementary to HTS by facilitating the selection of a smaller number of compounds. Machine learning (ML) methods with intelligent augmentation (IA) can enable rapid shortlisting of potentially bioactive compounds. In this goal, pharmacophore modeling can be an additional powerful IA tool to ML methods for further improving the efficiency of the methods. Training machine learning models with pharmacophore for a disease-specific target should better enable the down selection process of compounds. This chapter focuses on the potential of pharmacophores in IA for improving virtual screening. In addition to reviewing big data problems of pharmaceutical industries and their possible solutions, basics of pharmacophore modeling, its possible usefulness for ML methods along with two case studies from the author’s laboratory are presented and discussed.
MoreTranslated text
Key words
large compound databases,big databases,virtual screening,drug,pharmacophore-based
AI Read Science
Must-Reading Tree
Example
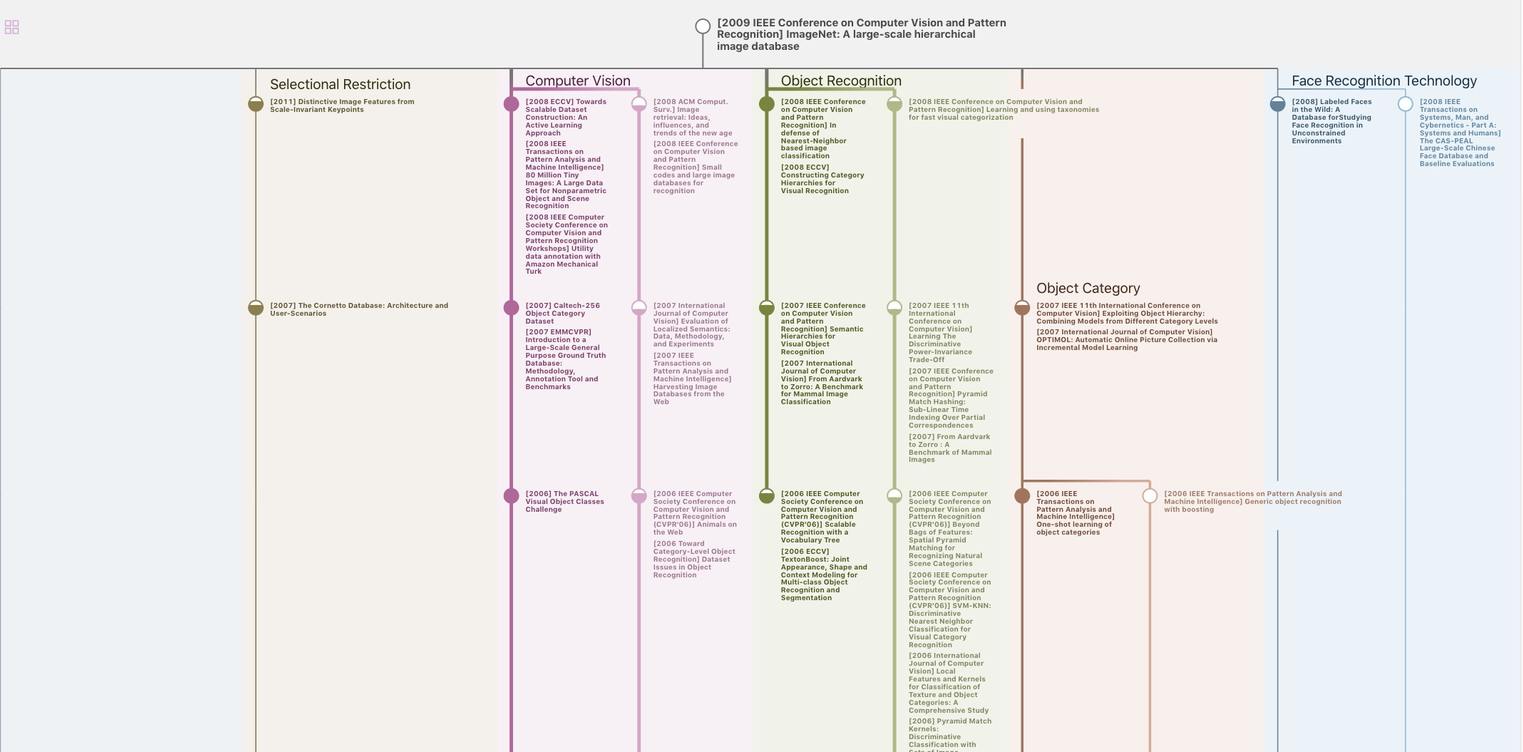
Generate MRT to find the research sequence of this paper
Chat Paper
Summary is being generated by the instructions you defined