Simultaneous Estimation of a Model-Derived Input Function for Quantifying Cerebral Glucose Metabolism with [18F]FDG PET
EJNMMI Physics(2024)
Key words
[18F]FDG,Cerebral metabolic rate of glucose,Image-derived input function,Microparameters,Model-derived input function,Non-invasive measurement,PET,Simultaneous estimation
AI Read Science
Must-Reading Tree
Example
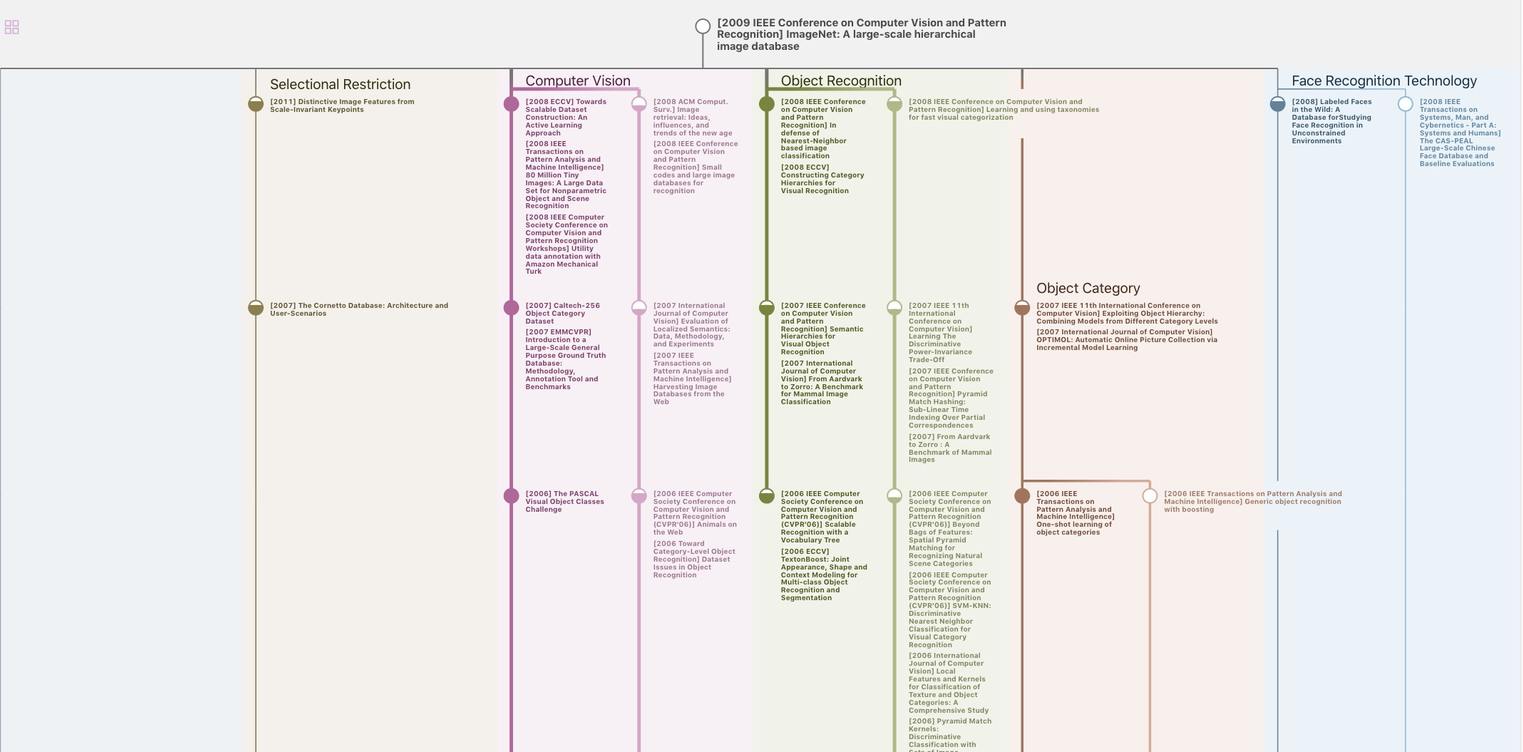
Generate MRT to find the research sequence of this paper
Chat Paper
Summary is being generated by the instructions you defined