Federated learning for feature-fusion based requirement classification
Cluster Computing(2023)
摘要
With the increasing complexity of products, the utilization of automated requirements classification in the process of requirement engineering is of positive significance to improve the efficiency of product development. There have been many experiments on the requirements classification based on artificial intelligence. However, many studies only use a single feature in semantic features or statistical features to classify requirements and put forward the problem of small-scale requirement datasets caused by the confidentiality of requirement data, which limits the application research of requirement classification in a large number of fields. Therefore, we propose a requirement classification model that integrates semantic and statistical features, and design a federated learning framework based on knowledge distillation without centralized servers in a ring architecture. In this paper, we extract a large amount of requirement data from a large number of requirement documents, and conduct experiments in the centralized learning environment and the federated learning environment respectively. The final experimental results show that our method can maintain the confidentiality of the requirement data and improve the communication efficiency in the process of model training with a slight decrease in the effect of requirement classification compared with centralized learning.
更多查看译文
关键词
Requirement classification,Federated learning,Knowledge distillation,Requirement engineering
AI 理解论文
溯源树
样例
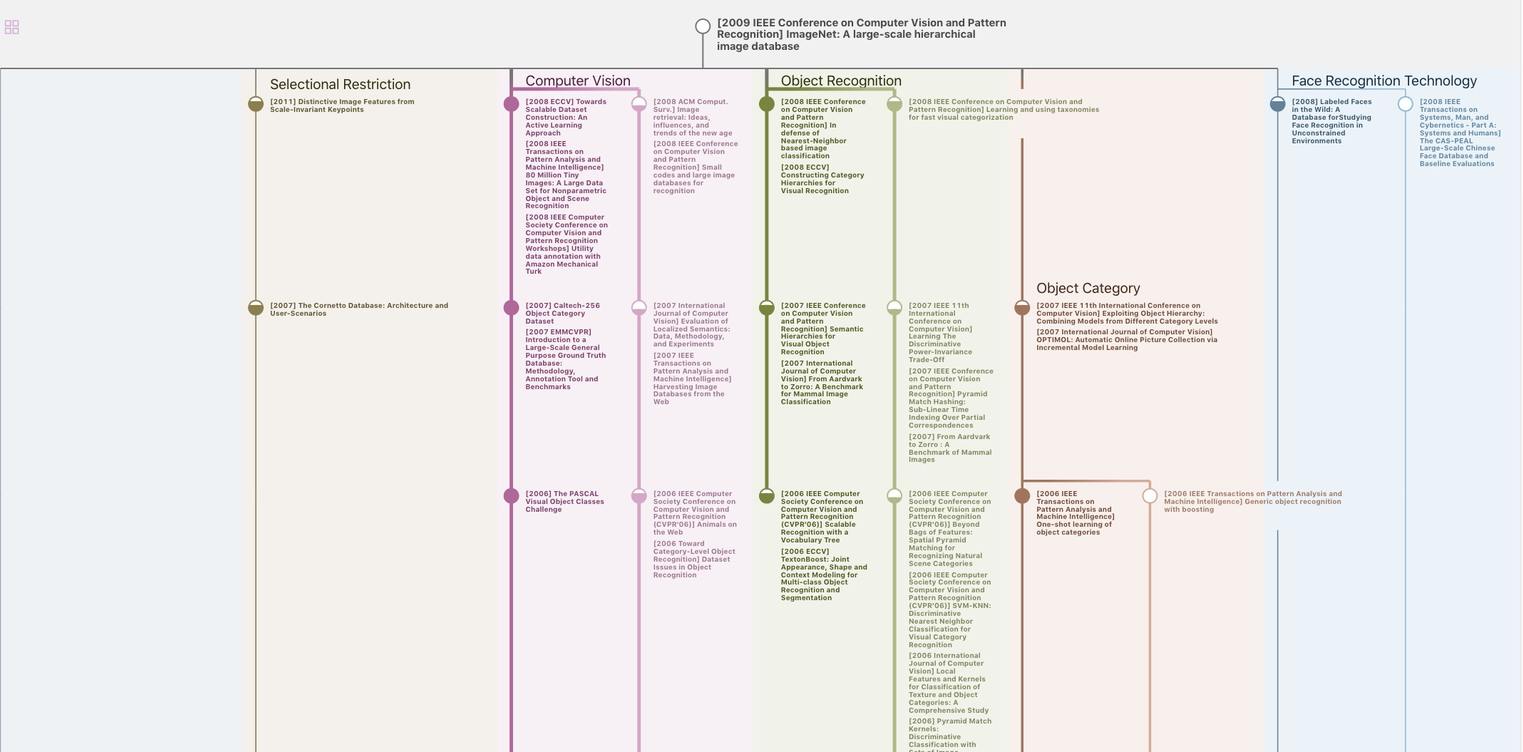
生成溯源树,研究论文发展脉络
Chat Paper
正在生成论文摘要