The limits of statistical learning in word segmentation: Accumulation of predictive information from unstructured input in the absence of (declarative) memory
crossref(2022)
摘要
Learning statistical regularities from the environment is ubiquitous across domains and species. It has been argued to support the earliest stages of language acquisition, including identifying and learning words from fluent speech (i.e., word-segmentation). Here, we ask how the statistical learning mechanisms involved in word-segmentation interact with the memory mechanisms needed to remember words, and if these mechanisms are tuned to specific learning situations. We show that, when completing a memory recall task after exposure to continuous, statistically structured speech sequences, participants track the statistical structure of the speech stream and are thus sensitive to probable syllable transitions, but hardly remember any items at all and initiate their productions with random syllables (rather than word-onsets). Only discrete familiarization sequences with isolated words produce memories of actual items. We provide computational evidence that such results are incompatible with extant memory-based chunking models of statistical learning. Further, we show that memory-less Hebbian learning mechanisms can account for earlier results purportedly showing that statistical learning leads to memories for chunks. Conversely, statistical learning predominantly operates in continuous speech sequences like those used in earlier experiments, but not in discrete chunk sequences likely encountered during language acquisition. Taken together, these results suggest that statistical learning might be specialized to accumulate distributional information, and that it is dissociable from the (declarative) memory mechanisms needed to acquire words.
更多查看译文
AI 理解论文
溯源树
样例
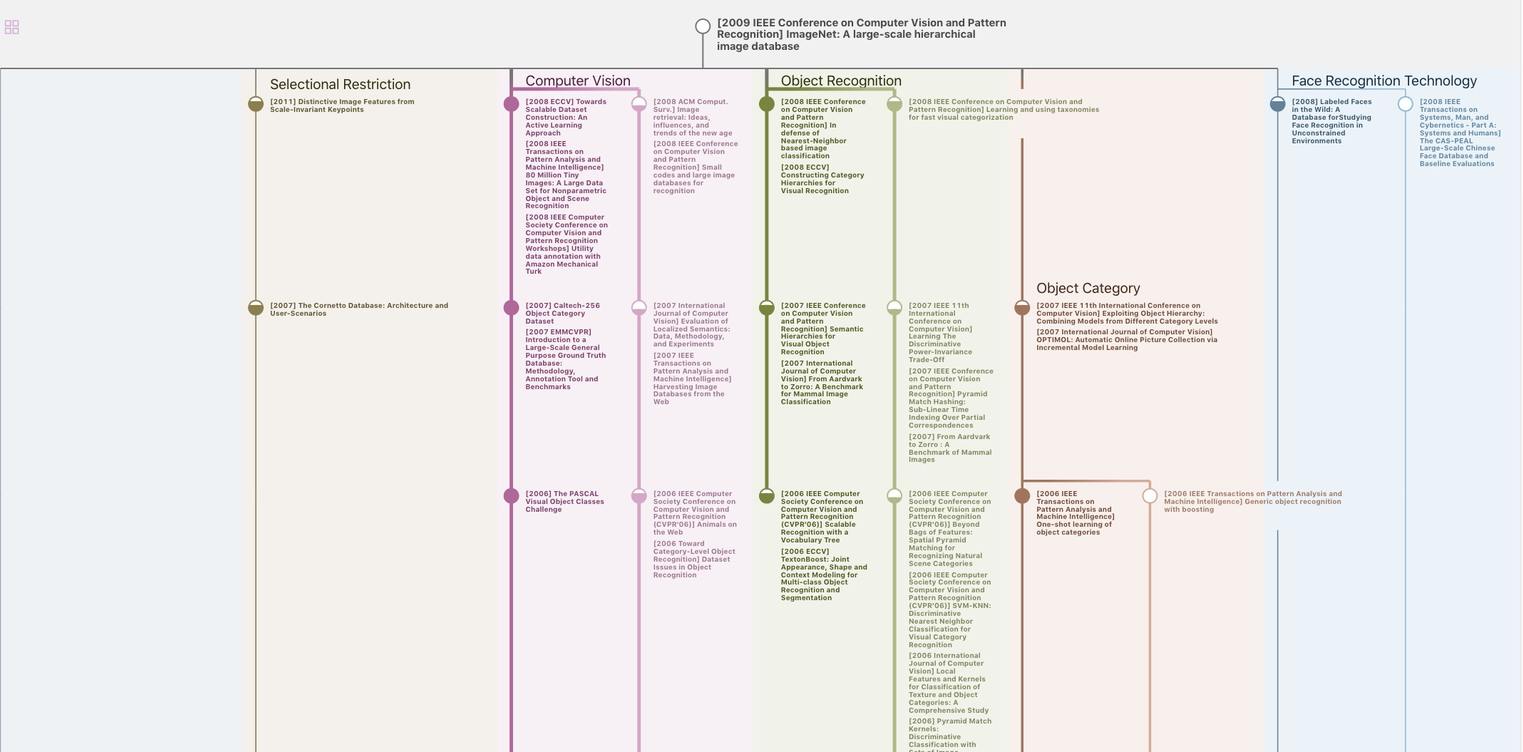
生成溯源树,研究论文发展脉络
Chat Paper
正在生成论文摘要