Towards an automated machine learning and image processing supported procedure for crack monitoring
Proceedings of the 5th Joint International Symposium on Deformation Monitoring - JISDM 2022(2022)
摘要
Development of automated and remotely controlled procedures for accurate crack detection and analysis is an advantageous solution when compared to time-consuming and subjective crack examination conducted by operators. Recent studies have demonstrated that Machine Learning (ML) algorithms have sufficient potential for crack measurements. However, training of large amount of data is essential. When working on single sites with permanently installed fixed cameras adoption of ML solutions may be redundant. The purpose of this work is to assess the performance of a procedure for crack detection based on an easy to implement workflow supported by the use of ML and image processing algorithms. The datasets used in this work are composed of temporal sequence of single digital images. The workflow proposed includes three main modules covering acquisition, optimization and crack detection. Each module is automated and basic manual input by an operator is only required to train the classifier. The processing modules are implemented in modular open-source programs (e.g., ImageJ and Ilastik). Results obtained in controlled conditions led to a satisfactory level of detection (about 99% of the crack pattern detected). Experiments conducted on real-sites highlighted variable detection capabilities of the proposed approach (from 12 to 96%). The main limitation of the approach is the production of false-positive detection due to significant variation in illumination conditions. Further work is being conducted to define scalability of the approach and to verify deformation detection capabilities.
更多查看译文
AI 理解论文
溯源树
样例
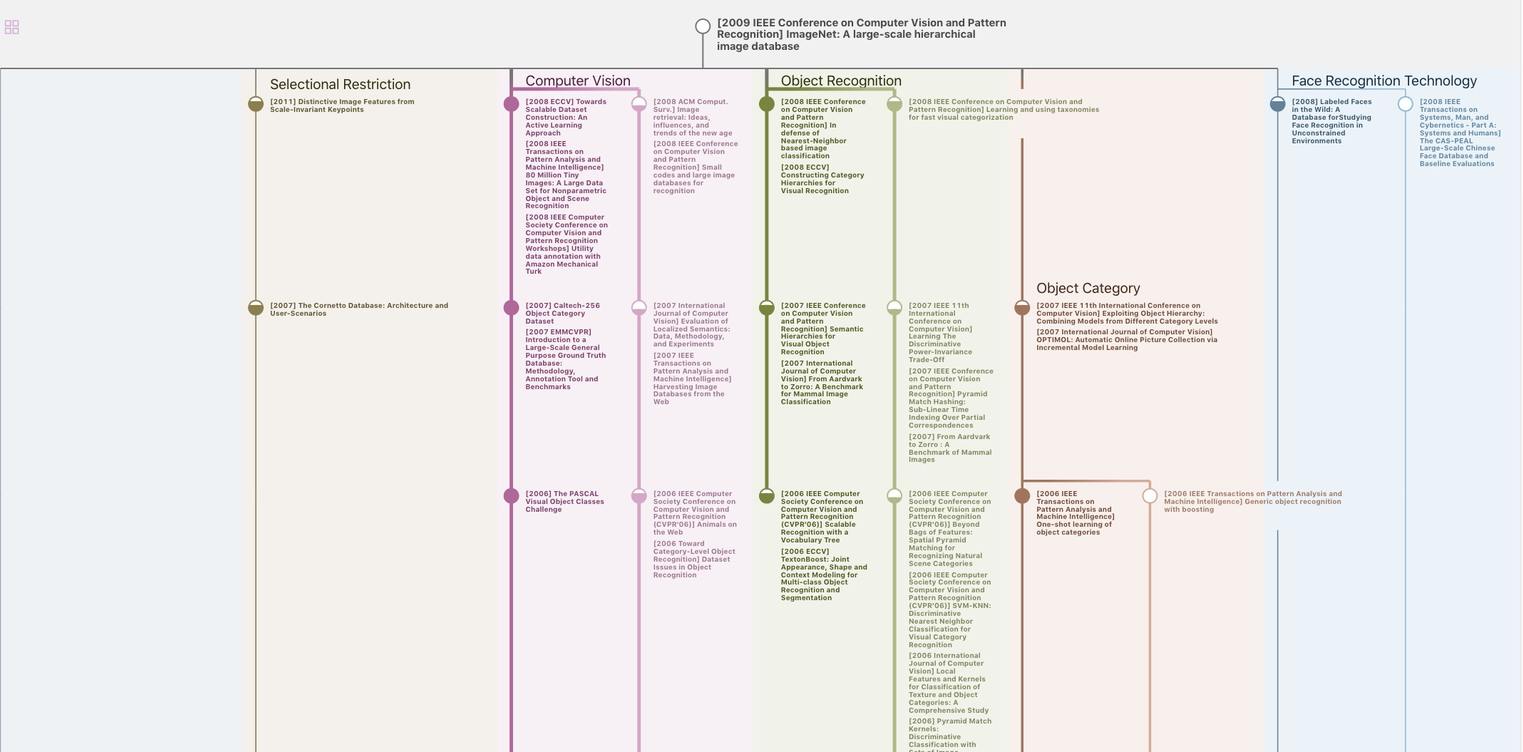
生成溯源树,研究论文发展脉络
Chat Paper
正在生成论文摘要