Machine Learning dimensionality reduction to detect EEG channel differences between PTSD cases and healthy controls
crossref(2022)
摘要
Abstract The goal of this study is to characterize EEG channel(s) that can help distinguish between PTSD and Healthy cases. For this purpose, we introduce a dimensionality reduction method that combines clustering and Support Vector Machine (SVM) weights to identify new feature weights for prediction of PTSD. We apply k-means clustering to dataset of each subject to classify the data points into 6 clusters, then utilize linear SVM to extract the weight matrix that shows the importance of channels in constituting the cluster classification of data points within each subject’s dataset. The significant SVM weights of F4, F8, and Pz are smaller in PTSD than in healthy subjects. This new approach can be used as a tool to better understand the interaction of EEG signals and diagnosis.
更多查看译文
AI 理解论文
溯源树
样例
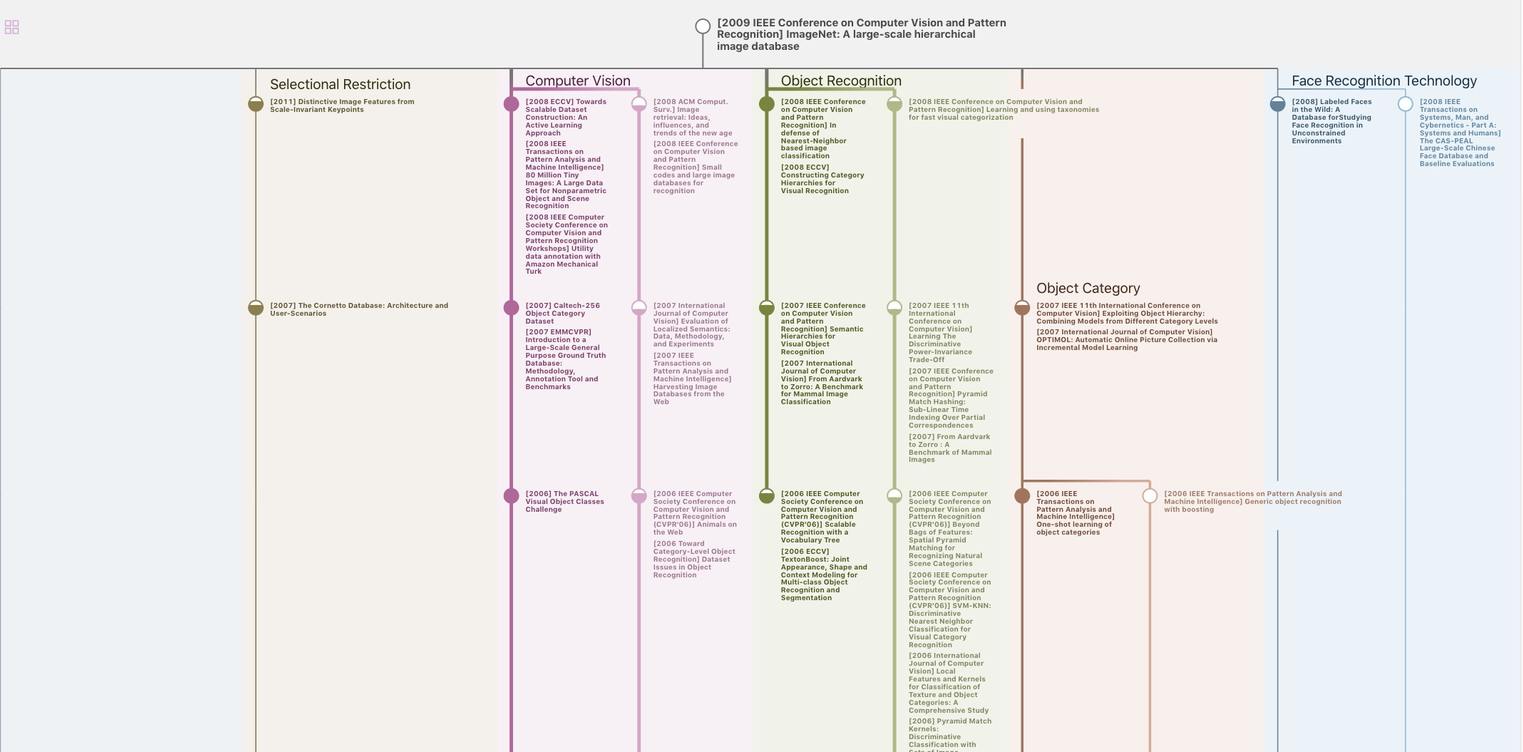
生成溯源树,研究论文发展脉络
Chat Paper
正在生成论文摘要