CNN-LSTM-based Prediction of the Anode Effect in Aluminum Electrolytic Cell
2022 41st Chinese Control Conference (CCC)(2022)
摘要
The prediction of the anode effect and its active extinguishing have been a major research focus in the modern aluminum electrolysis industry as an abatement in the non-active anode effect can greatly improve the current efficiency of aluminum electrolysis and reduce energy consumption and greenhouse gas emissions. Despite the extensive application of neural networks and deep learning networks in industrial fault diagnosis, the neural network structure alone is poor at capturing the underlying information related to the anode effect. In response to the above issues, an XGBoost-CNN-LSTM hybrid network model has been proposed. Applying the XGBoost algorithm to feature engineering on the original data, the model extracts the underlying information related to the camera effect from multiple features through the double-layer CNN, and then learns the features through the LSTM network to work out the probability of the anode effect. According to the results of testing and verification of this model on the actual production data set of an aluminum plant in Guizhou, this model outperforms the LSTM network in the prediction accuracy.
更多查看译文
关键词
Deep learnng network, aluminum electrolysis industry, anode effect
AI 理解论文
溯源树
样例
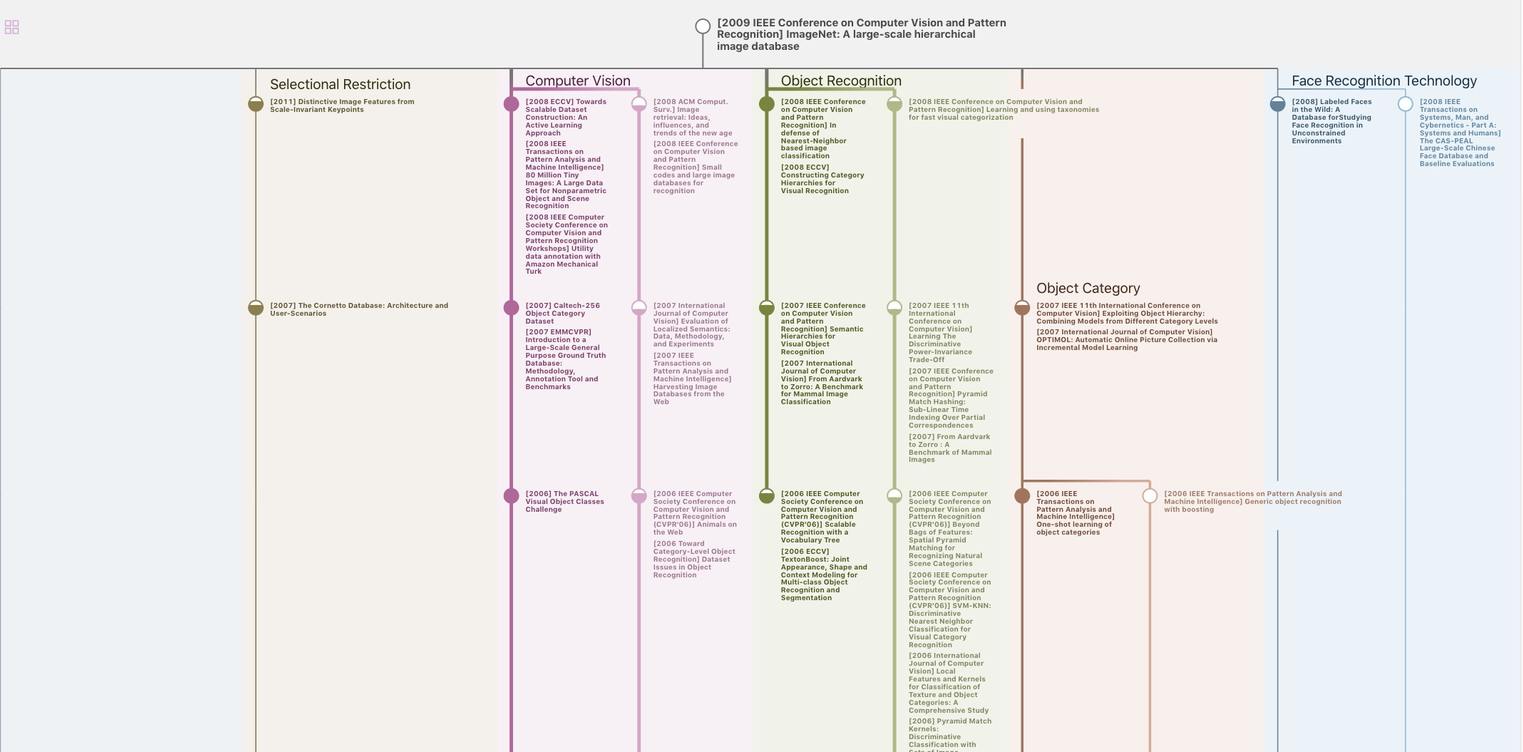
生成溯源树,研究论文发展脉络
Chat Paper
正在生成论文摘要