Multi-Level Reservoir Identification with Logs Based on Machine Learning
SPWLA 63rd Annual Symposium Transactions(2022)
Abstract
Machine learning algorithms have become powerful tools for modeling in the engineering field. They are suitable for solving problems that can't be effectively solved by traditional physical models or empirical models due to the complex relationship of variables. Since the traditional interpretation method of log data is based on petrophysical mechanisms and models, many assumptions are needed, which may lead to deviations in practical application. Therefore, it is of great significance to achieve reservoir fluid identification when using machine learning processing and interpreting log data. The existing reservoir identification methods have not thoroughly mined the internal relationships of log data. Moreover, the distribution of reservoir categories is seriously unbalanced. Reservoirs with similar physical properties are easily confused in identification. We propose an effective method of machine learning to solve the above problems. A long short-term memory network (LSTM) is used to characterize the time series characteristics of logs varying with depth domain. The kernel of the convolutional neural network (CNN) is used to slide on log curves to characterize their relationships. Considering the unbalanced distribution and the different development values of reservoirs categories, the weighted cross-entropy loss function is used to improve the weight of oil-bearing reservoirs with less distribution but higher development value when model training. According to the difference and similarity of reservoir physical properties, a multi-level reservoir identification process is designed: Level-I (reservoir and non-reservoir), Level-II (oil-bearing reservoirs, water-bearing reservoirs, and dry layer), and Level-III (oil layer, oil-water layer, poor oil layer, and water layer, oily-water layer). This method is verified on the log data of oil fields, in which the reservoir categories distribution is highly unbalanced. Moreover, the fraction of oil-bearing reservoirs is 9%, which agreement with the actual industrial situation. A series of comparative experiments proved that the parallel network structure of LSTM and CNN can fully examine the internal relationships and sequence characteristics of log curves. The weighted cross-entropy loss function significantly improves the fluid identification accuracy of oil-bearing reservoirs. Moreover, the multi-level reservoir identification method is more accurate in avoiding the identification confusion of reservoirs with similar physical properties. The experimental results demonstrate that this method is very practical and useful to help geological experts and engineers find reservoirs and complete evaluation.
MoreTranslated text
Key words
logs,machine learning,multi-level
AI Read Science
Must-Reading Tree
Example
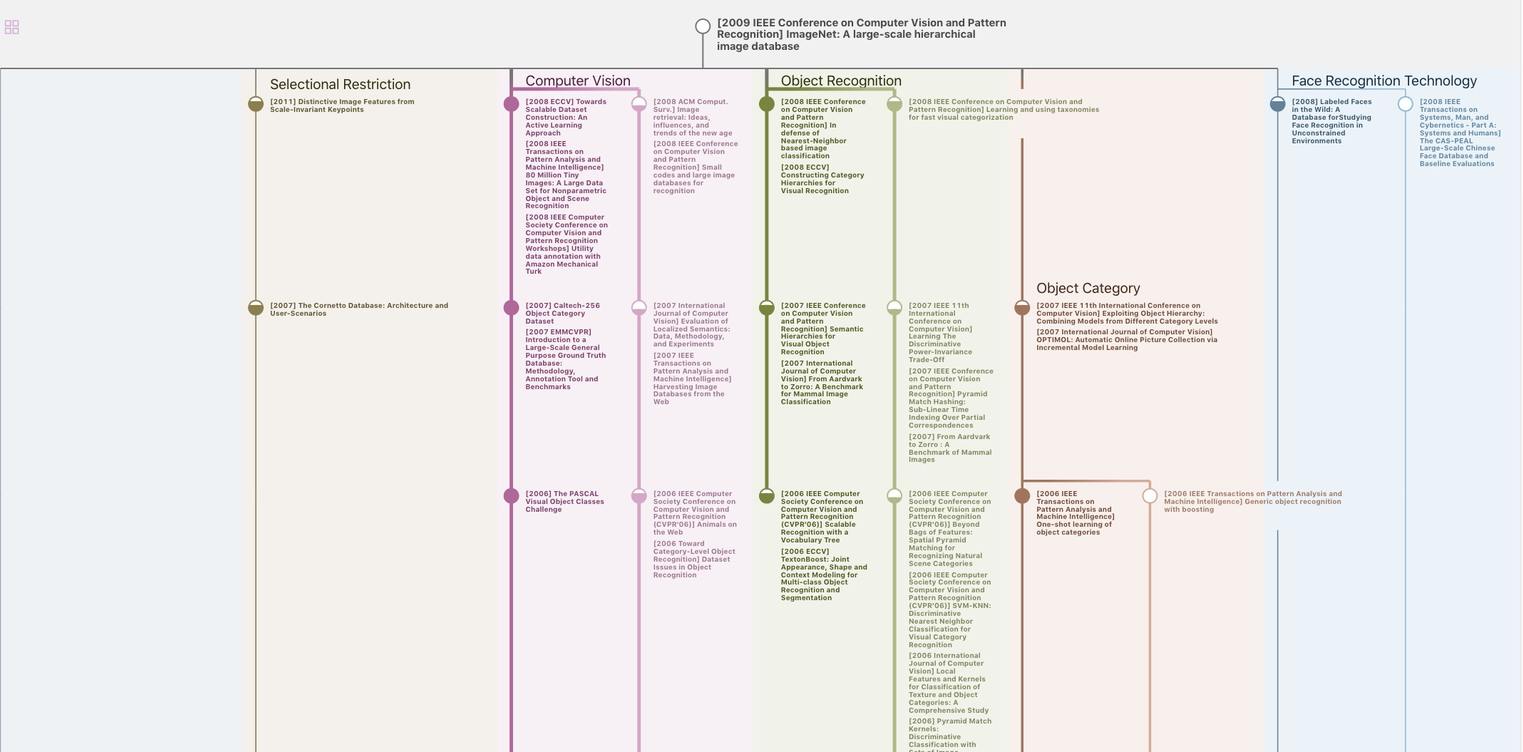
Generate MRT to find the research sequence of this paper
Chat Paper
Summary is being generated by the instructions you defined