Genomic prediction of agronomic traits in sugarcane using machine learning and quantitative genetics
Research Square (Research Square)(2022)
摘要
Abstract To improve the efficiency of sugarcane breeding, introduction of genomic selection (GS) into breeding programs is anticipated. For sugarcane cultivars with highly heterozygous polyploid genomes, non-additive genetic effects must be considered in genomic prediction models. In this context, the use of machine learning techniques is an effective approach to model nonadditive genetic effects. In addition, pedigree information that tracks sugarcane breeding lineages can be used to calculate the degree of association among genotypes and as input for predictive models. In the present study, we used the breeding population used in a KARC/NARO sugarcane breeding program in Japan as experimental material and verified the following attributes. Specifically, the prediction accuracy of BLUP was validated based on genomic and pedigree information. In addition, a new machine learning method that can explicitly process the interaction between whole-genome markers and genetic background was developed, and comparative verification was performed using the conventional random forest method. Pedigree information showed high predictive accuracy, and when columns of the numerator relationship matrices were used as input for the machine learning models, their accuracy was identical or superior to that of BLUP depending on traits. Finally, we confirmed the effectiveness of the proposed machine learning method using interactions among markers as a model. Overall, these GS methods are expected to improve sugarcane breeding efficiency.
更多查看译文
关键词
genomic prediction,agronomic traits,sugarcane,quantitative genetics,machine learning
AI 理解论文
溯源树
样例
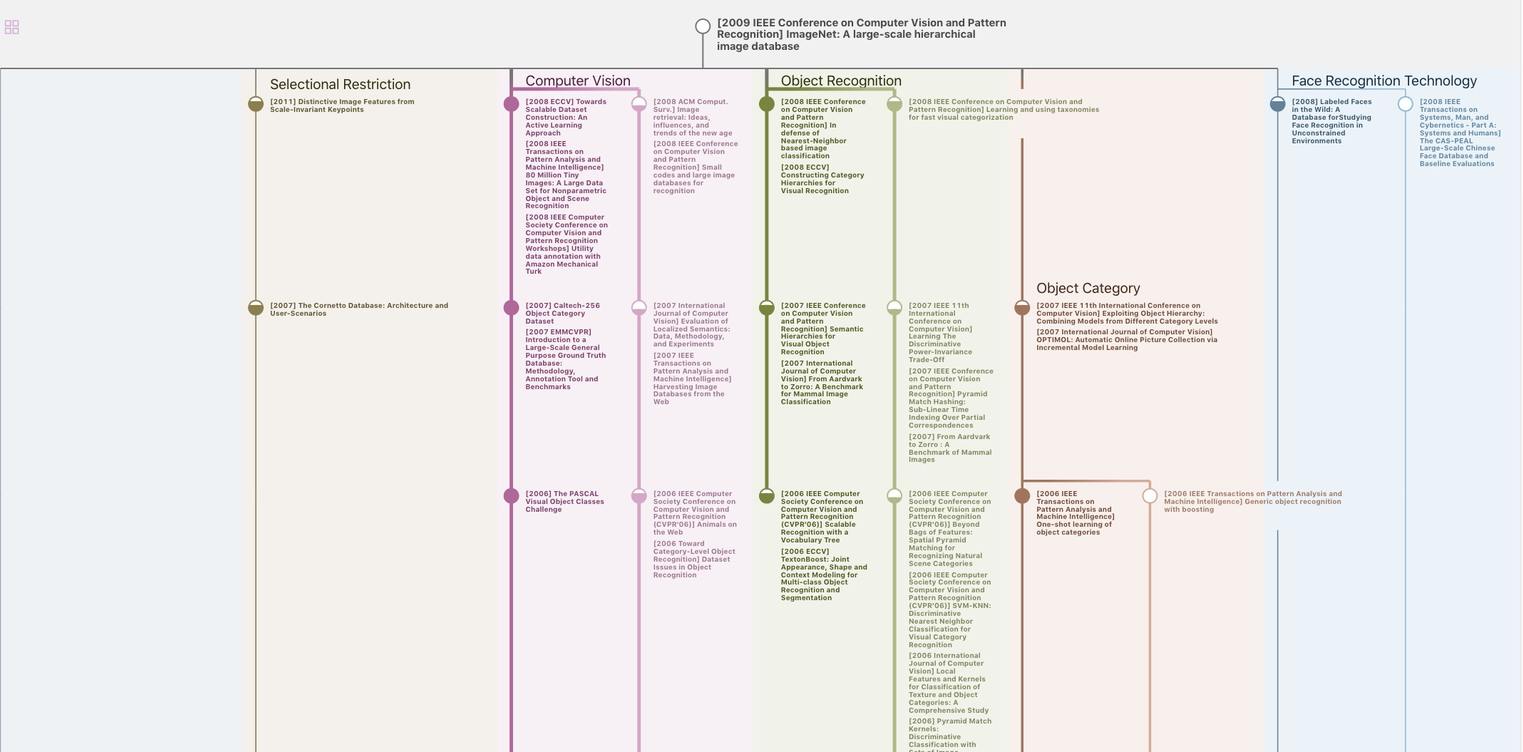
生成溯源树,研究论文发展脉络
Chat Paper
正在生成论文摘要