A comparison of four spatial interpolation methods for modeling fine-scale surface fuel load in a mixed conifer forest with complex terrain
Advances in Forest Fire Research 2022(2022)
Abstract
Forest fuel inventory, monitoring and mapping provide the basis for fuel management activities, including assessing wildfire hazards and risk, prescribed fire planning, designing silvicultural treatments, and predicting fire behaviour and effects at various scales. One of the most significant challenges in developing accurate surface fuel maps is capturing the spatial variability of fuel load within and between different fuel components. In this paper, we compare the performance of four spatial interpolation methods for estimating and mapping fine-scale fuel load in a dry mixed conifer forest in Colorado, USA. The four approaches are: classification, multiple linear regression, ordinary kriging, and regression kriging. We chose these methods because they are commonly used in ecological studies and cover a range of SIM including, non-geostatistical, geostatistical, and hybrid approaches. All SIM methods yielded unbiased fuel load estimates, with mean error within 3% of the observations with MAPE from 100% to 40%, depending on the specific fuel com-ponent and spatial interpolation method. Our results indicate that regression kriging was able to better capture the fine-scale spatial variability in fuel load compared to other spatial interpolation methods.
MoreTranslated text
Key words
classification, multiple linear regression, ordinary kriging, regression kriging
AI Read Science
Must-Reading Tree
Example
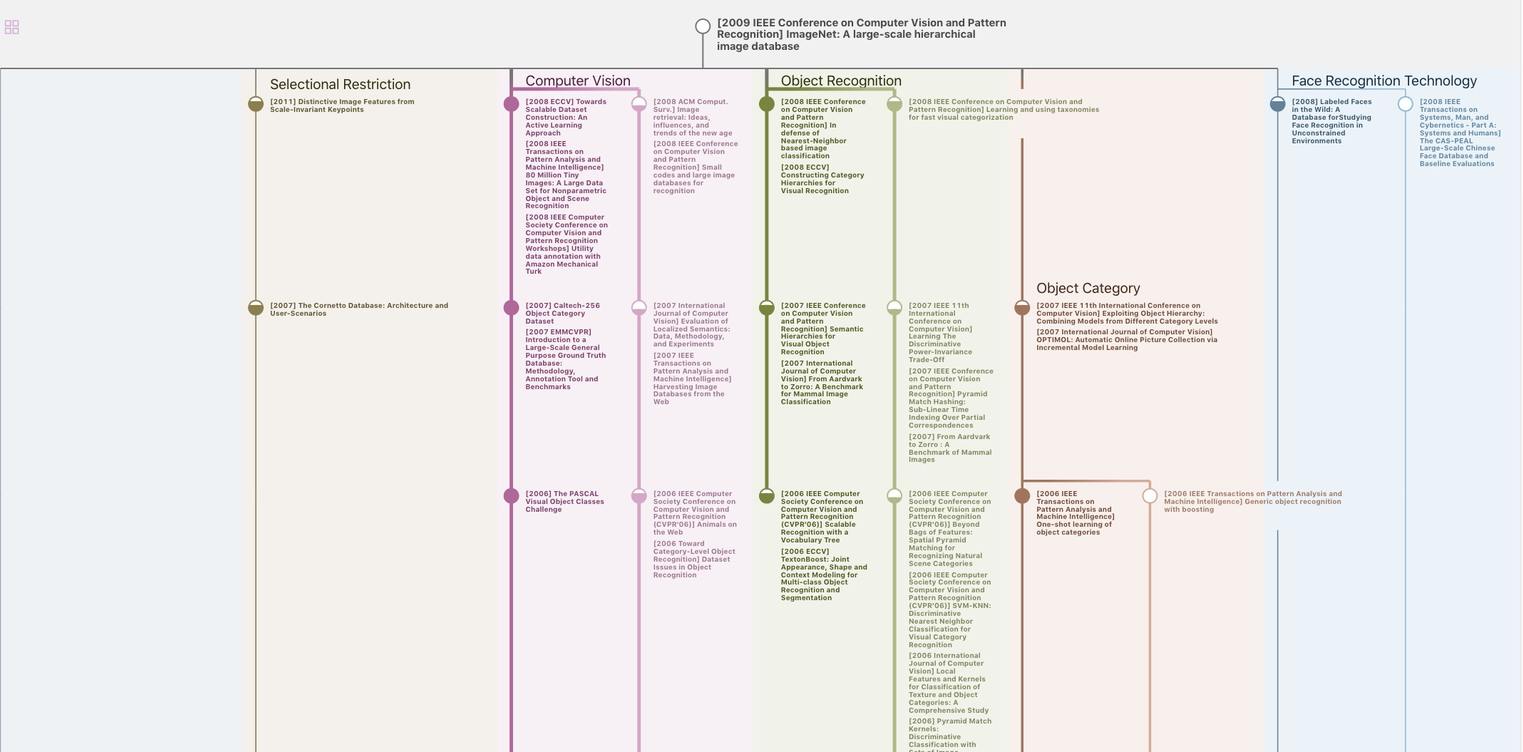
Generate MRT to find the research sequence of this paper
Chat Paper
Summary is being generated by the instructions you defined