Dynamic Channel Pruning with Adaptive Weight Learning
Authorea (Authorea)(2022)
摘要
Dynamic channel pruning is widely used in model compression to improve the efficiency of neural networks. Although dynamic pruning can remove redundant channels dynamically, the parameters still remain unchanged, which can limit the performance as the response of each neuron changes with different inputs. In this paper, we propose a novel dynamic channel pruning method with adaptive weight learning, which can adaptively adjust both the parameters and widths of the filters simultaneously. Specifically, we design an adaptive-weight convolution module, which can be customized for different inputs under the guidance of global context information to capture representative local patterns and synthesize interested features. At the same time, we utilize a channel importance prediction module to predict the saliency of each channel. Based on the channel saliency, unimportant channels can be removed dynamically to speed up the convolution. These two modules work jointly to achieve a good trade-off between model performance and computational complexity. Experiments on image classification and object detection tasks demonstrate that our method can greatly reduce the computational burden while maintaining the performance, which outperforms state-of-the-art methods.
更多查看译文
关键词
dynamic channel pruning,adaptive weight learning
AI 理解论文
溯源树
样例
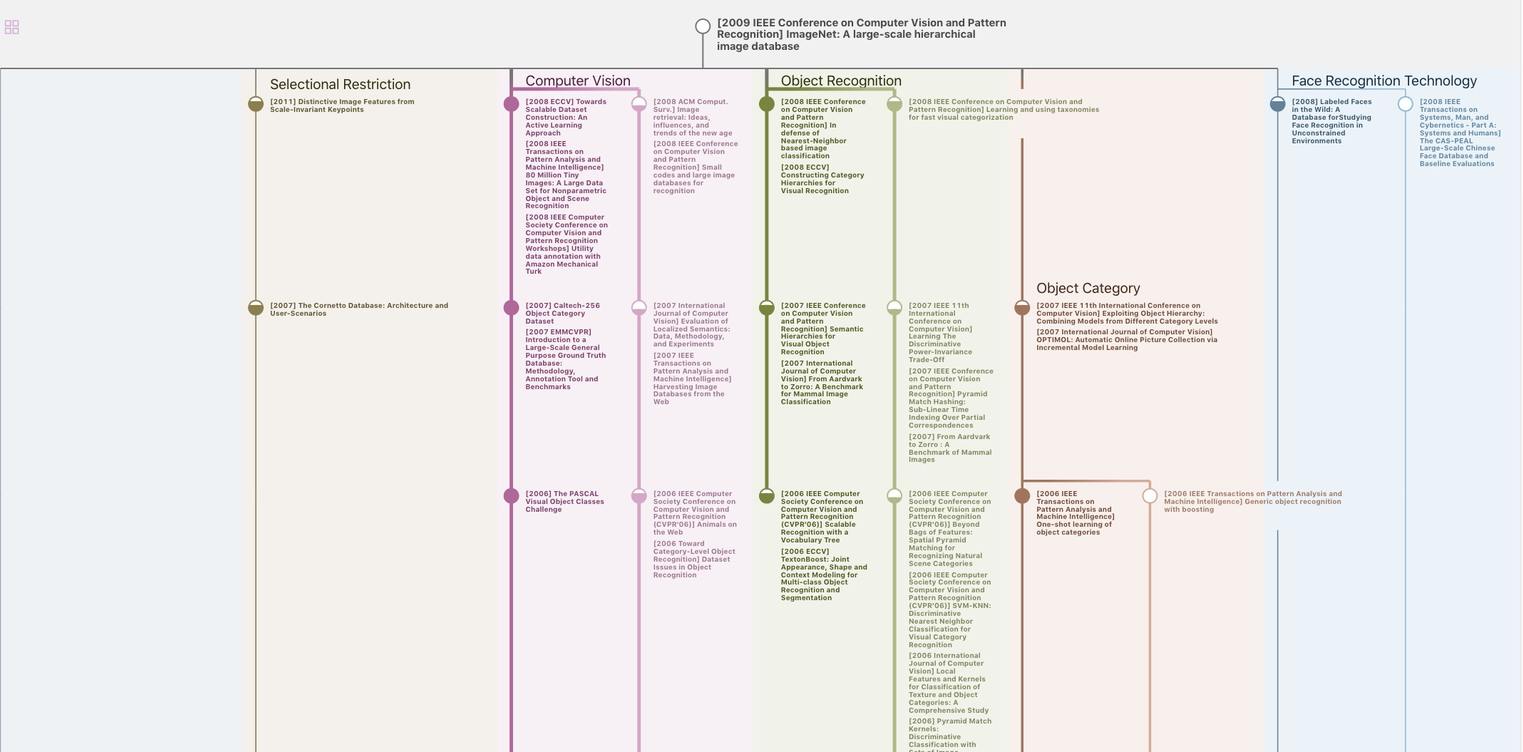
生成溯源树,研究论文发展脉络
Chat Paper
正在生成论文摘要