Mortality prediction of surgical intensive care unit patients using deep learning-based survival models
Malaysian Journal of Science(2022)
Abstract
Mortality prediction in surgical intensive care units (SICUs) is considered to be among the most critical steps in enforcing efficient treatment policies. This study aims to evaluate the performance of various deep learning models in predicting the mortality of patients admitted to SICUs. The survival of 2,225 adult patients admitted to SICUs was modeled using five salient deep learning-based survival models, namely, Cox-CC, Cox-Time, DeepSurv, DeepHit, and N-MTLR. The data were extracted from the Medical Information Mart for Intensive Care II (MIMIC-II) database. The performance of the models was compared using the time-dependent concordance index (Ctd-index) and integrated Brier score (IBS). From among the five models, DeepSurv achieved the most accurate prediction, while Cox-Time demonstrated the least optimal predictive ability. For DeepSurv, Cox-CC, DeepHit, N-MTLR, and Cox-Time, the mean Ctd -index was 0.773, 0.767, 0.765, 0.732, and 0.659, and the mean IBS was 0.181, 0.192, 0.195, 0.212, and 0.225, respectively. DeepSurv, Cox-CC, and DeepHit yielded comparable performance. Deep learning models are free from the stringent assumptions inherent in standard survival models. Hence, these models are considered flexible alternatives to the standard approaches in scalable, real-world survival problems.
MoreTranslated text
Key words
surgical intensive care unit,intensive care unit,mortality,survival,learning-based
AI Read Science
Must-Reading Tree
Example
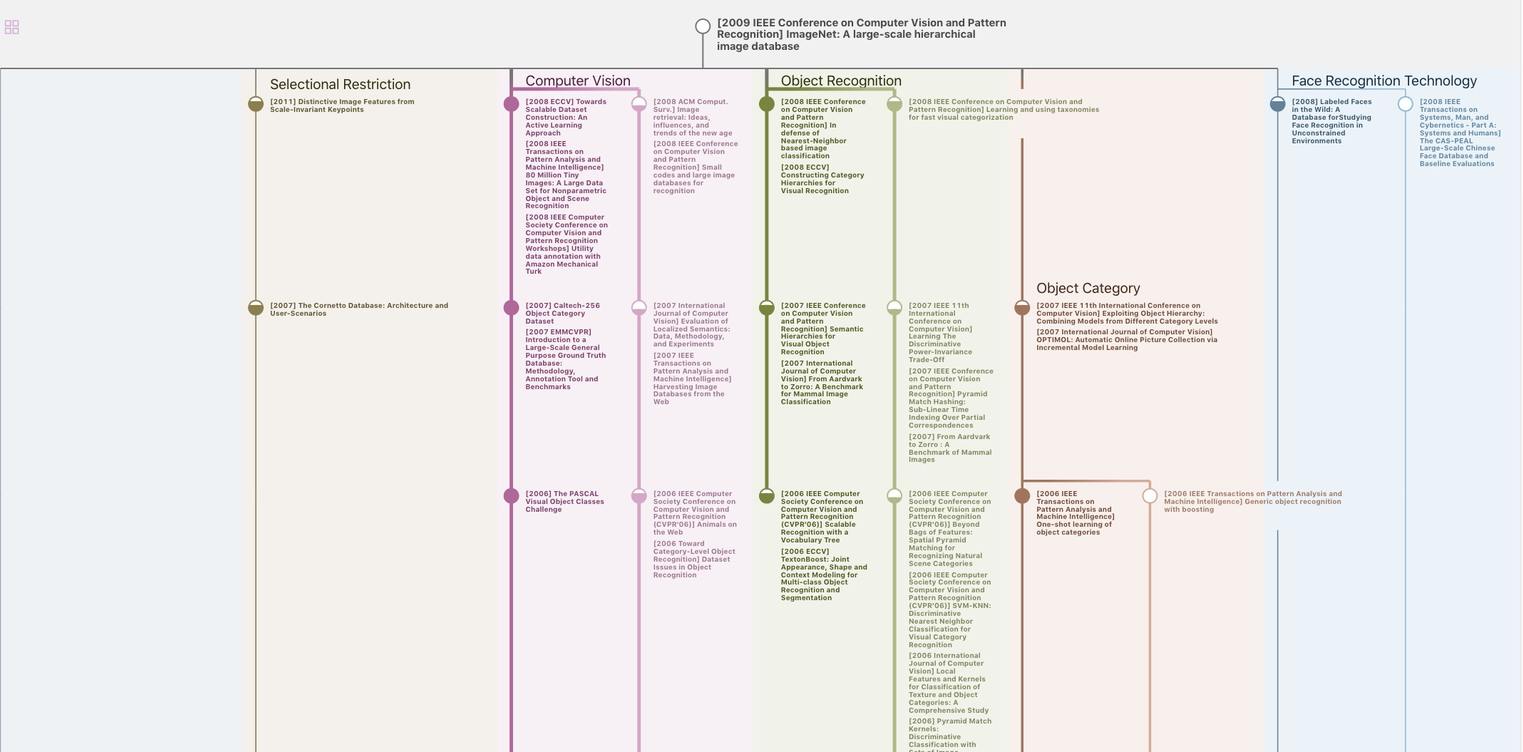
Generate MRT to find the research sequence of this paper
Chat Paper
Summary is being generated by the instructions you defined