Benchmarking of Machine Learning Methods for Multiscale Thermal Simulation of Integrated Circuits
IEEE Transactions on Computer-Aided Design of Integrated Circuits and Systems(2023)
摘要
Multiscale thermal analysis in integrated systems is required for capturing both device-level and circuit-level dynamics. Traditional analysis with finite element (FE) models can be accelerated by using machine learning (ML) methods. In this article, a performance benchmarking between three ML methods for thermal simulation is carried out: 1) artificial neural networks (ANNs); 2) proper orthogonal decomposition with radial basis functions (POD-RBFs); and 3) finally, POD-RBF-ANN is used as a hybrid ML method. The (dis)advantages of the different methods are demonstrated for the thermal simulation of a multiscale photonic chip. The ML models are trained with FE data for both linear and nonlinear dynamics and are tested for inter- and extra-polation prediction accuracy. A computational speed increase with a factor >7500 compared to FE is obtained. Furthermore, ANNs prove to be the best suited for the simulation of nonlinear dynamics. POD-RBF is the best method for minimizing training time and combining the best of both methods in POD-RBF-ANN creates an ML model with a short training phase and highly accurate predictions.
更多查看译文
关键词
Machine learning (ML), silicon photonics, thermal modeling
AI 理解论文
溯源树
样例
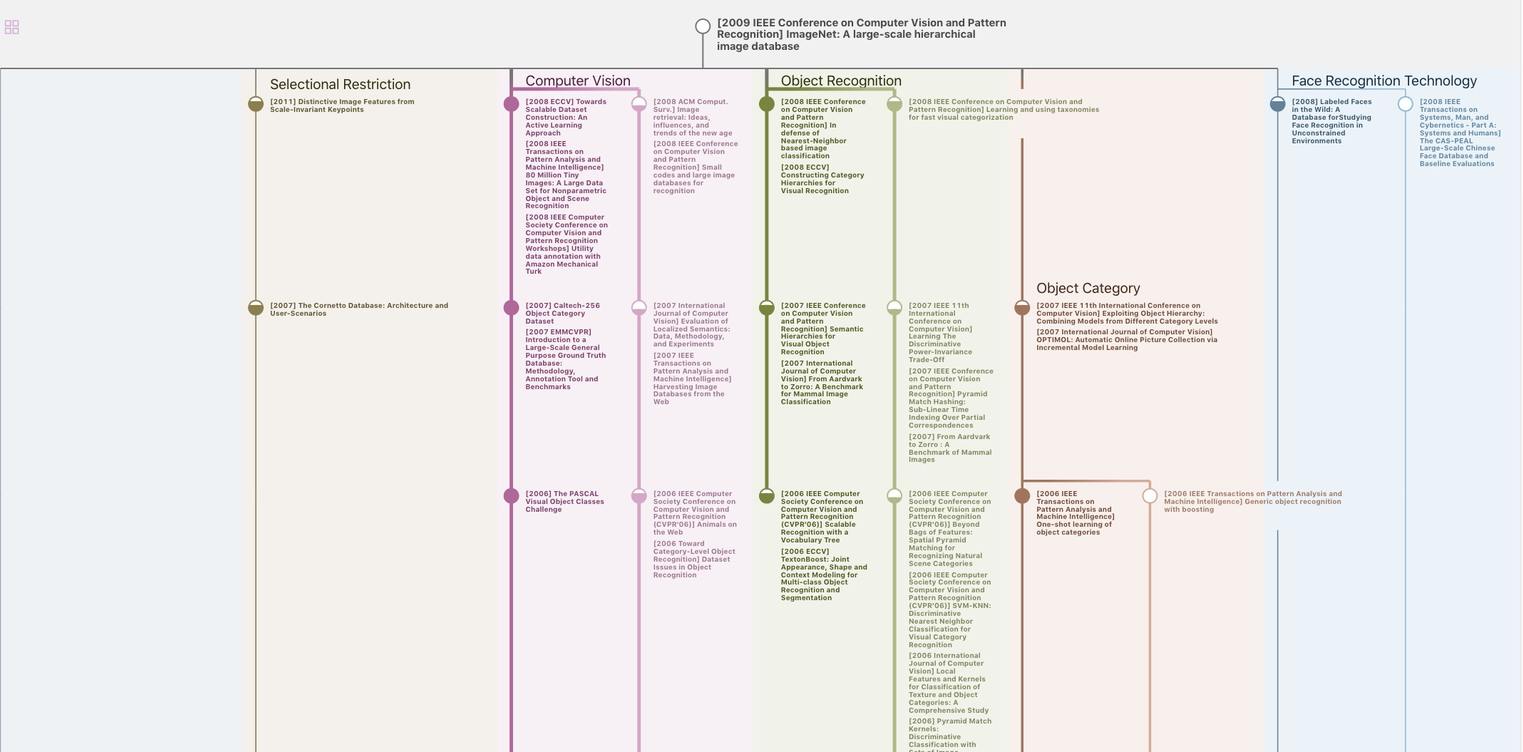
生成溯源树,研究论文发展脉络
Chat Paper
正在生成论文摘要