Coordinate Embedding Transformer Model for Optical Music Recognition on Monophonic Scores
2022 12th International Conference on CYBER Technology in Automation, Control, and Intelligent Systems (CYBER)(2022)
摘要
Optical Music Recognition (OMR) is an image recognition task that researchers try to teach computers to read music notation. In recent years, the convolution recursive neural network algorithm achieves great success in music symbols recognition tasks, especially in the monophonic score. However, some challenges remain in music symbol recognition, such as the notes in different positions of the staff which have the same image features represent different meanings. It is hard to distinguish the notes only with the way of convolution. In addition, context relationship is usually used to improve the overall accuracy of the music symbol recognition. In this paper, we propose a Coordinate Embedding Transformer model(CETr). We add pixel coordinates into feature patches to make the note positions of the staff participate in training and predicting, which can increase the difference between two notes with the different positions of the staff. Due to the Transformer which is designed for sequence modeling and transduction tasks being reliable to deal with the context relationship in a music score, we leverage the Transformer architecture for symbols-level score generation. Experiments show that the CETr model outperforms the current state-of-the-art models on both clean and distorted monophonic scores.
更多查看译文
关键词
music score,symbols-level score generation,monophonic scores,optical music recognition,image recognition,music notation,music symbol recognition,sequence modeling,transduction tasks,convolution recursive neural network,computer teaching,coordinate embedding transformer model,CETr model
AI 理解论文
溯源树
样例
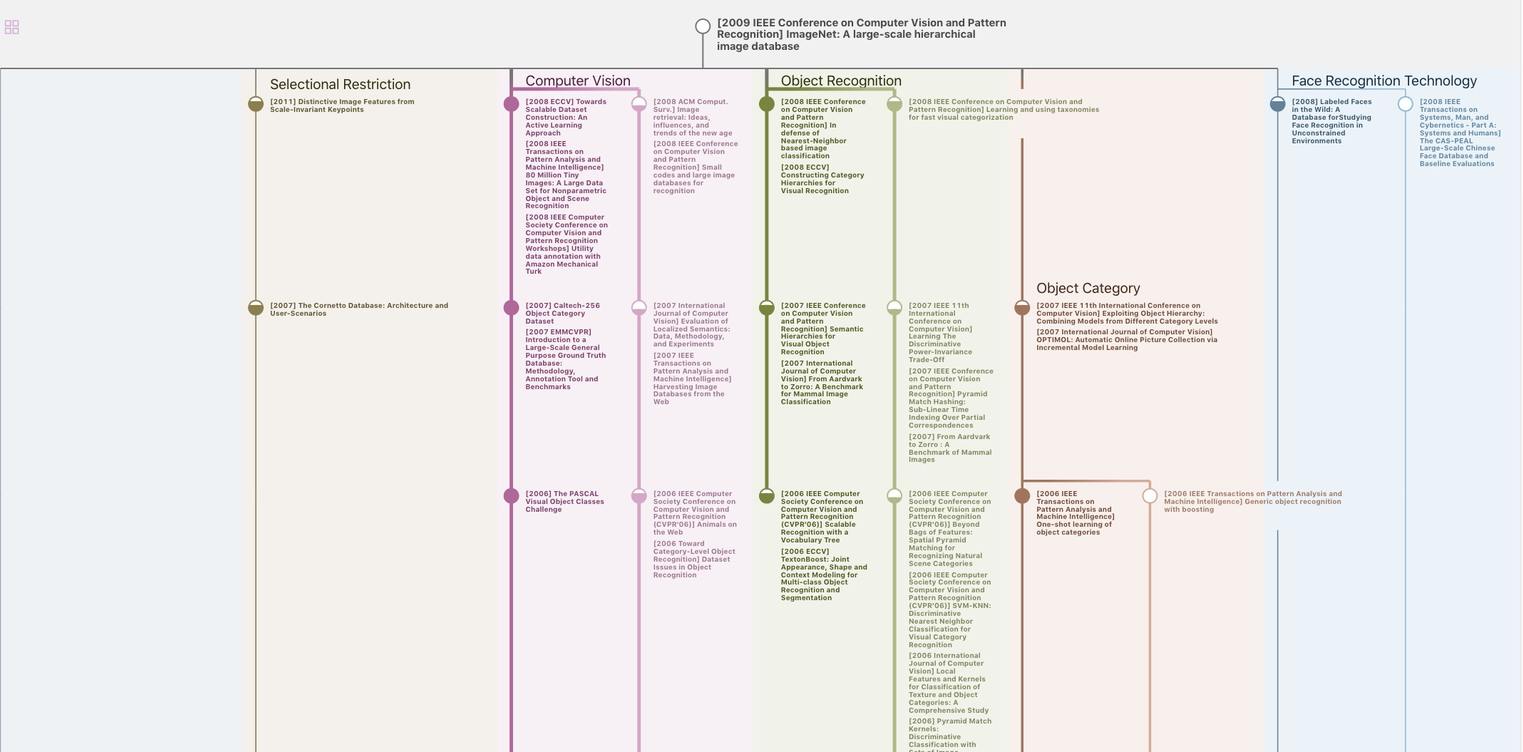
生成溯源树,研究论文发展脉络
Chat Paper
正在生成论文摘要