Selecting Climatic Variables to Model Plant Disease Risk
SSRN Electronic Journal(2023)
摘要
Predicting the risk of a disease in a pathosystem based on a set of climatic variables usually requires handling a great amount of input variables; thus, many of them are often irrelevant and redundant. Therefore, the aim of this work was to compare procedures to select variables and fit climate-based models to predict the occurrence of a disease in a pathosystem. Four selection methods were compared in terms of the predictive capacity of the fitted model: stepwise (S) (with backward, forward, and both selection options), and other common ML methods: filter (F), genetic algorithm (GA) and Boruta (B). The disease risk predictors were constructed with a logistic regression model and with the random forest ML algorithm, using all the variables available and the subgroups of variables selected by each method. Data of three pathosystems were processed: two involving Begomovirus –one in common bean (Phaseolus vulgaris L) and the other in soybean (Glicine max)– and the third involving Mal de Rio Cuarto virus in maize (Zea mays L.). The data sets differed in sample size and number of variables, and were obtained from several years of crop monitoring. The logistic regression model with the stepwise-forward option, fitted alone or associated with other statistical criteria (VIF and P-value) to reduce the model, outperformed the other algorithms using climatic variables as model inputs. The accuracy of the random forest classifier did not vary among the different subgroups of climatic variables. The stepwise-forward regression, implemented as wrapper, yielded the most accurate predictive model.
更多查看译文
关键词
climatic variables,plant,disease
AI 理解论文
溯源树
样例
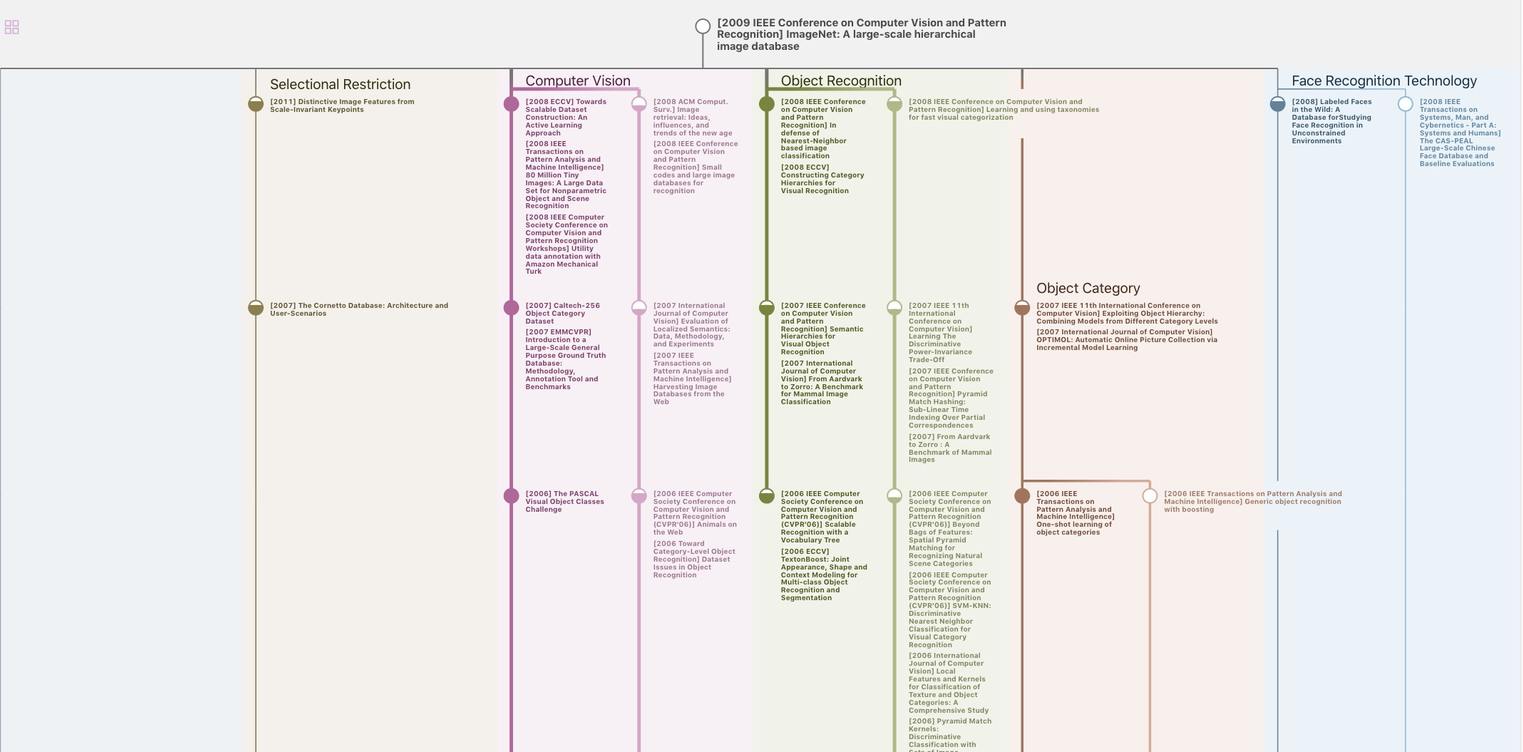
生成溯源树,研究论文发展脉络
Chat Paper
正在生成论文摘要