Cezsar: A Contrastive Embedding Method for Zero-Shot Action Recognition
SSRN Electronic Journal(2023)
Abstract
This paper proposes a novel Zero-Shot Action Recognition (ZSAR) method based on contrastive learning. We aim to reduce the semantic gap and domain shift problems in ZSAR by learning a joint embedding space. This space is learned by aligning visual appearance with their corresponding description in natural language. Our motivation comes from recent works that reported impressive results by representing videos and labels with descriptive sentences embedded with pre-trained models on the paraphrasing task. We design an automatic negative sampling procedure to augment the training dataset and generate unpaired data, i.e., visual appearance and unrelated descriptions. Then, we train an encoding model to retain positive pairs as close as possible and negative ones as far as possible. Using our negative sampling procedure, we can overcome the data limitation to perform contrastive learning and train the model in a few hours in a conventional Graphics Processing Unit (GPU). The text encoding ability of our model allowed us to use semantic side information as WordNet definitions of recognized objects in scenes and descriptions from video captioning methods. Our results are state of the art on the UCF-101 and Kinetics-400 datasets under several split configurations. Our code is available at https://github.com/valterlej/cezsar.
MoreTranslated text
Key words
action,recognition,contrastive,zero-shot
AI Read Science
Must-Reading Tree
Example
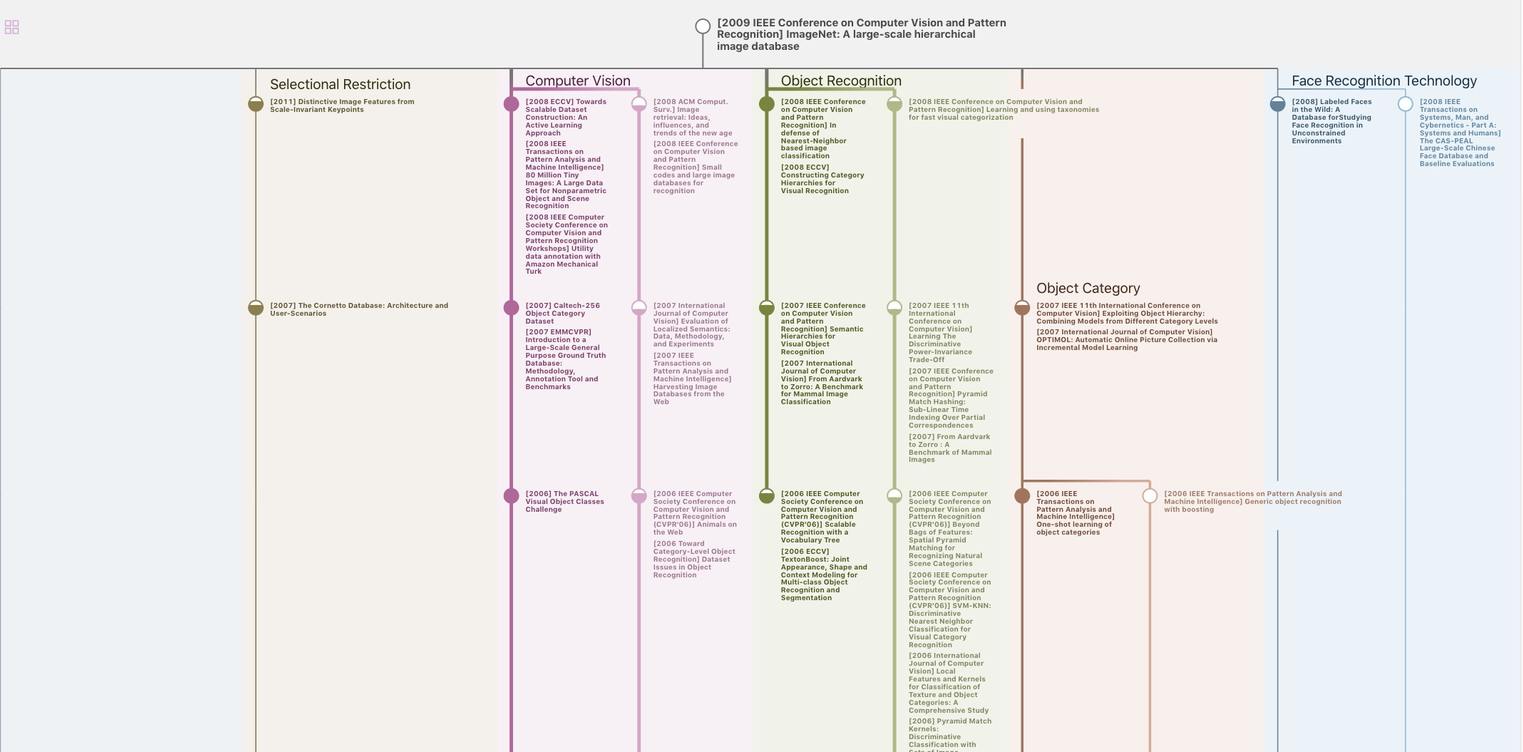
Generate MRT to find the research sequence of this paper
Chat Paper
Summary is being generated by the instructions you defined