Robust Resilient H ∞ State Estimation for Time-varying Recurrent Neural Networks Subject to Probabilistic Quantization Under Variance Constraint
International Journal of Control, Automation and Systems(2023)
摘要
This paper is concerned with the robust resilient H ∞ state estimation problem for time-varying recurrent neural networks (TVRNNs) with probabilistic quantization under variance constraint. Here, a situation is considered where the signals are quantized before entering the network, and the occurrence probability is assumed to be known. In addition, during the design of the state estimation algorithm, the additive variation of the estimator gain is considered to reflect the parameter deviation that may occur during the execution. The main purpose is to design a finite-horizon resilient state estimation algorithm such that, in the presence of probabilistic quantization and estimator gain perturbation, some sufficient criteria are obtained for the estimation error system to satisfy the prescribed H ∞ performance requirement within the finite-horizon and the error variance boundedness. Finally, a numerical example is conducted to verify the feasibility of the presented estimation algorithm against the probabilistic quantization and estimator gain perturbation.
更多查看译文
关键词
H ∞ performance,probabilistic quantization,resilient state estimator,time-varying recurrent neural networks,variance constraint
AI 理解论文
溯源树
样例
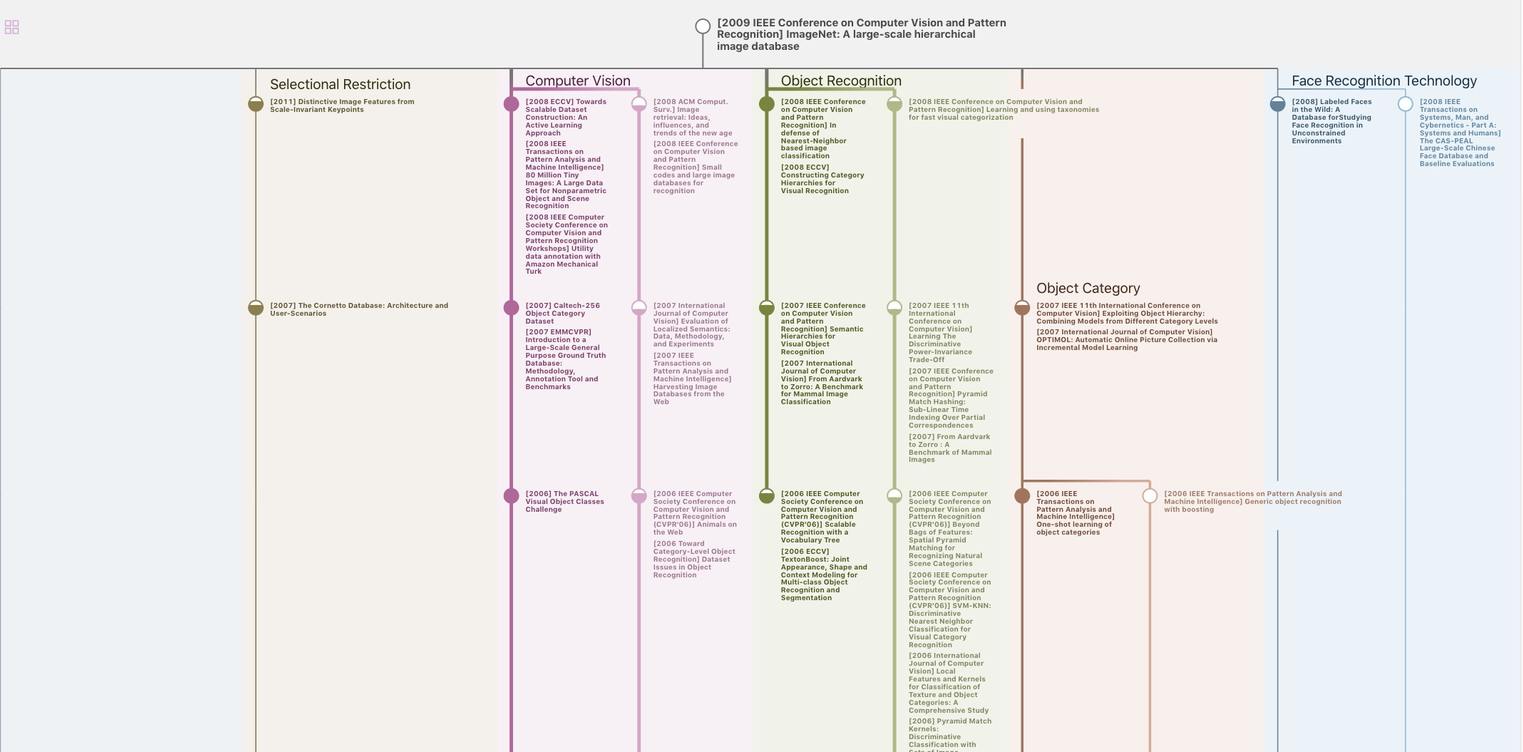
生成溯源树,研究论文发展脉络
Chat Paper
正在生成论文摘要