Deep Reinforcement Learning-based Intelligent Agent for Autonomous Air Combat
2022 IEEE/AIAA 41st Digital Avionics Systems Conference (DASC)(2022)
Abstract
In future aerial combat, more tactical flight maneuvers are necessary for aerial engagement. It is challenging to solve all the unpredictable air combat scenarios only with the conventional rule-based flight maneuvers. This study aims to design an intelligent agent model to perform autonomous air combat, focusing on the close dogfight scenarios. We apply the deep reinforcement learning techniques to allow the ownship aircraft to learn the offensive maneuvers for tracking and shooting down the target aircraft. We use two representative state-of-the-art algorithms to train our agent's policy model, Proximal Policy Optimization (PPO) and Soft Actor-Critic (SAC). The agent model learns the combat strategy in a realistic flight environment, Digital Combat Simulator (DCS), which has high fidelity for simulating air combat scenarios. To verify our proposed approach, we design baseline policy models in terms of the learning algorithms and time-delayed state transition methods. In the evaluation, the suggested policy model is able to handle the delayed state transition of the aircraft system and shows better performance for target tracking in the various air combat scenarios.
MoreTranslated text
Key words
intelligent agent,combat,learning-based
AI Read Science
Must-Reading Tree
Example
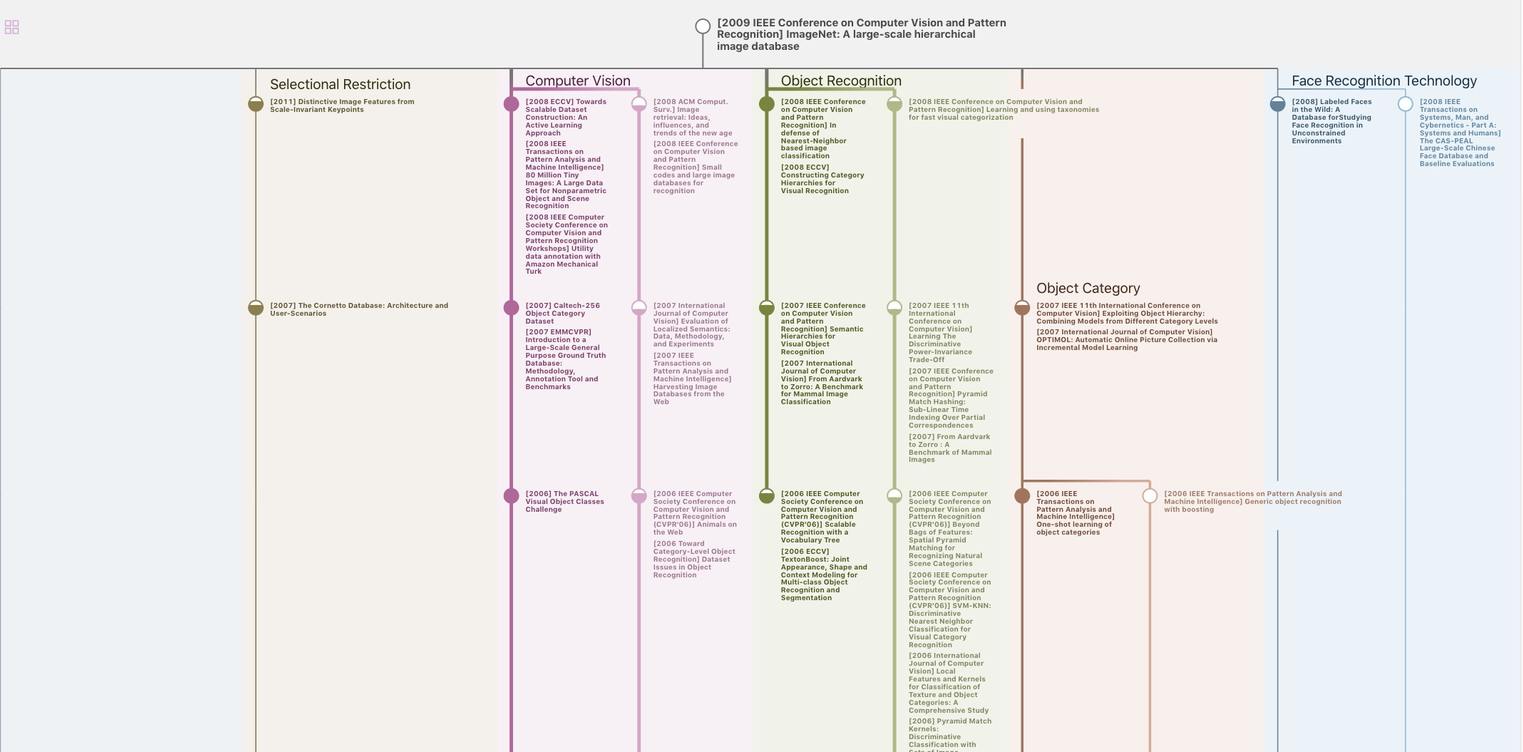
Generate MRT to find the research sequence of this paper
Chat Paper
Summary is being generated by the instructions you defined