Sample-Efficient Deep Reinforcement Learning via Balance Sample
2022 37th Youth Academic Annual Conference of Chinese Association of Automation (YAC)(2022)
摘要
In this paper, we propose two algorithms to improve sample efficiency by focusing on late stage samples in episodes. The first algorithm is Balanced Sample Experience Replay (BSER). Unlike the traditional random sampling approach, this algorithm improves the final score and stability in environment by learning more late stage experience in the corresponding episode. The second algorithm is weight-corrected DQN (WCDQN). This algorithm differs from the traditional undifferentiated update approach by differentially updating the samples used for training to improve the final score and stability in environment. We tested both algorithms on a classic Atari game environment and demonstrated the effectiveness of the algorithms.
更多查看译文
关键词
deep reinforcement learning,balance,sample-efficient
AI 理解论文
溯源树
样例
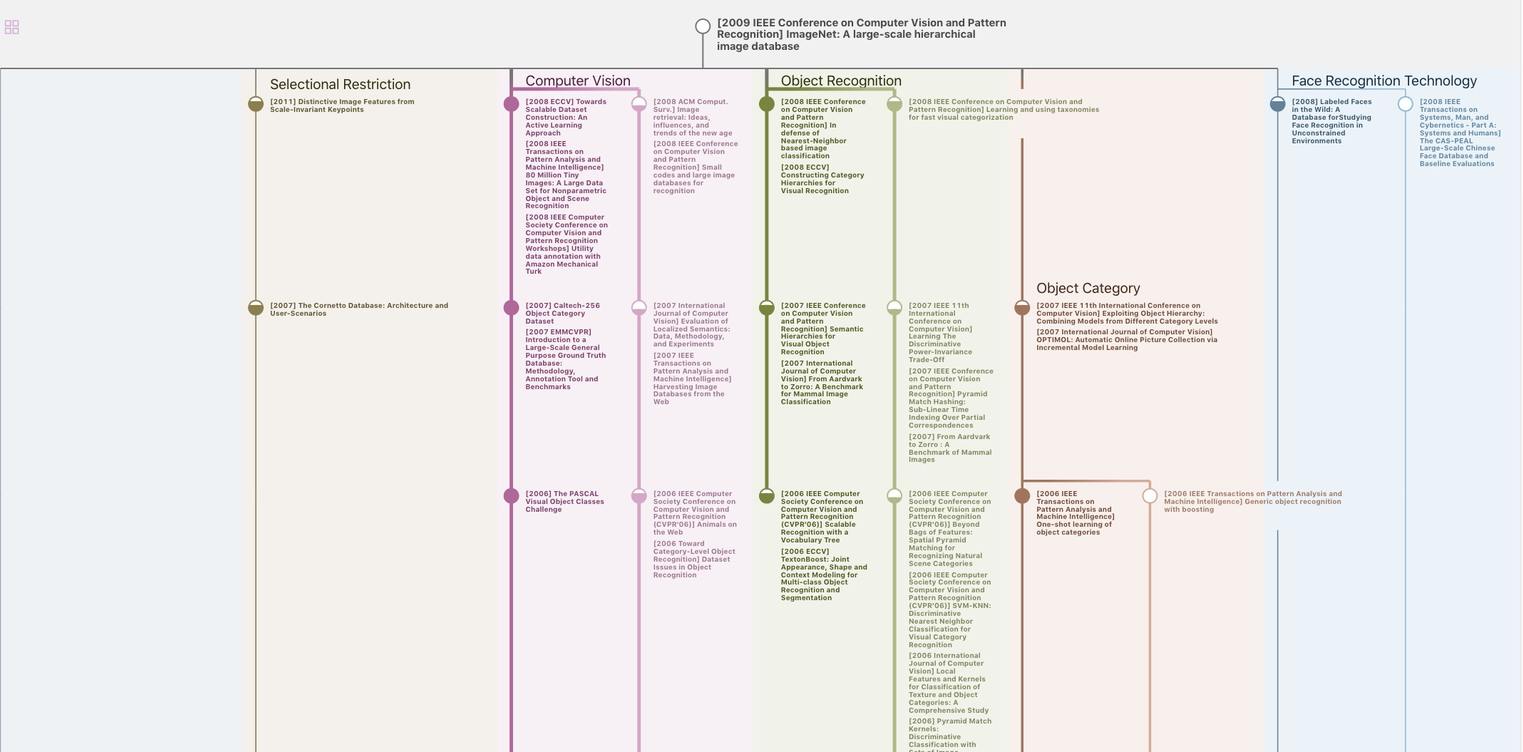
生成溯源树,研究论文发展脉络
Chat Paper
正在生成论文摘要