Novel Feature-Disentangled Autoencoder Integrating Residual Network for Industrial Soft Sensor
IEEE Transactions on Industrial Informatics(2023)
摘要
In order to overcome the low robustness and weak generalization in existing deep autoencoder (AE) for soft sensor modeling, a novel feature-disentangled AE (FDAE) integrating residual network (Resnet) (FDAE-Resnet) is proposed in this article. Different from the traditional deep AE that only can learn entangled features, the FDAE can obtain disentangled multisource features including trend features, periodic features and spatial features by a new trend-periodic long short-term memory (TPLSTM) and a novel dynamic self-attention convolutional neural network (DSACNN). The trend and periodic signals decomposed from input variables are fed into the TPLSTM to learn trend and periodic features in time and frequency domain, respectively. Then, the DSACNN is utilized to capture dynamic spatial features in spatial domain by adding a new attention mechanism. Moreover, disentangled multisource features are obtained by concatenating trend features, periodic features and spatial features together. Finally, the Resnet is utilized to build the soft sensor model by establishing the relationship between disentangled multisources features and outputs. To illustrate the effectiveness and superiority of the proposed method, the FDAE-Resnet is applied in the actual polypropylene process industry for melt index modeling. The experiment results show that compared with other state-of-the-art methods, the FDAE-Resnet can reduce the root mean square error by 26.2% and the mean absolute percentage error by 38.2% on average in the changed working conditions, respectively.
更多查看译文
关键词
Deep learning (DL),disentangled multisource features,process industry,soft sensor modeling
AI 理解论文
溯源树
样例
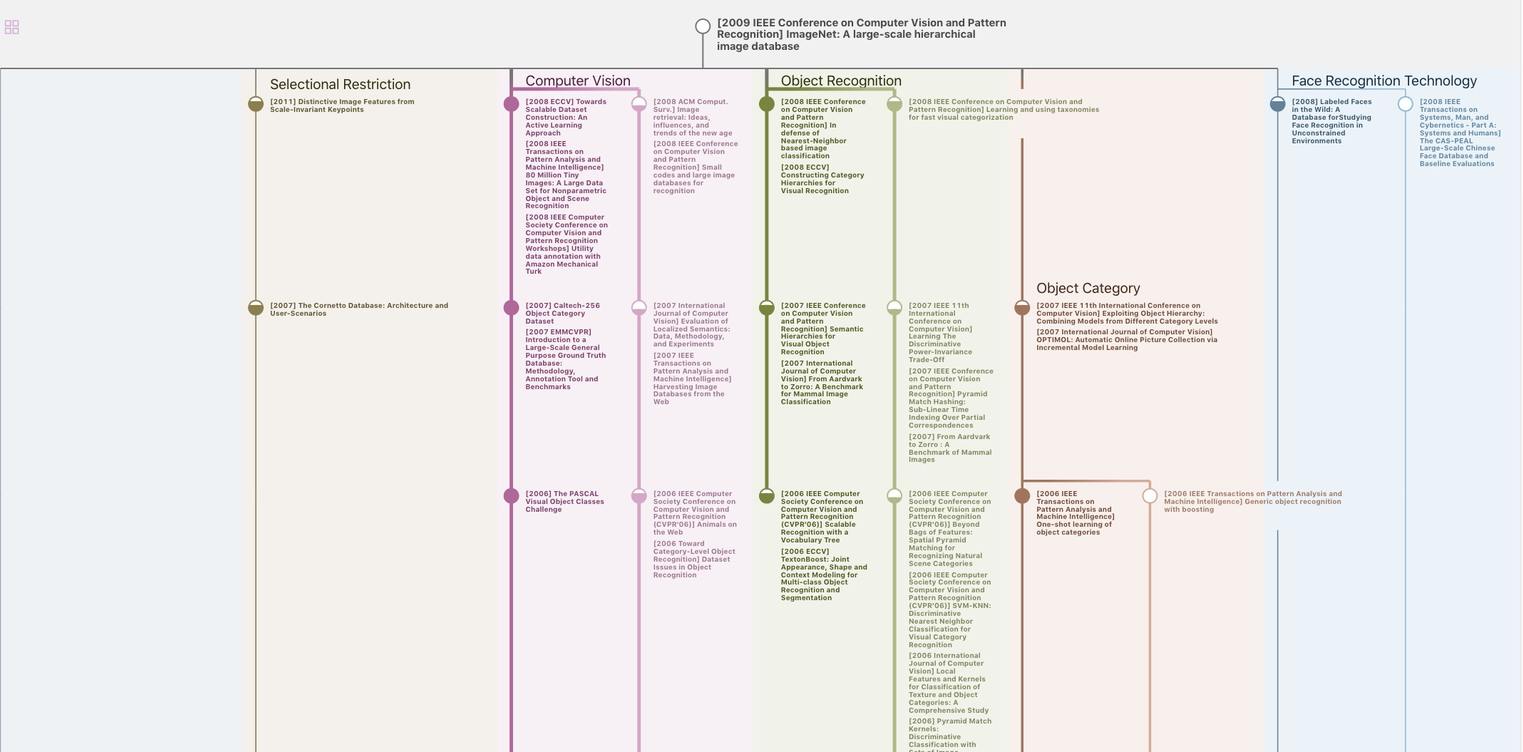
生成溯源树,研究论文发展脉络
Chat Paper
正在生成论文摘要