Domain Discrepancy-Guided Contrastive Feature Learning for Few-Shot Industrial Fault Diagnosis Under Variable Working Conditions
IEEE Transactions on Industrial Informatics(2023)
摘要
Recent advances in data-driven methods have significantly promoted intelligent fault diagnostics for varied industrial applications. However, due to the limitations of machine fault data and the varied scenarios in the context of industrial working conditions, existing diagnostic models can hardly achieve satisfactory results. In this article, we propose a domain discrepancy-guided contrastive feature learning framework for few-shot fault diagnosis under varied working conditions. Unlike the conventional contrastive learning paradigm using manually augmented data, a sample pairs construction is implemented based on the differences between domain distributions for data acquired under different working conditions. The similarity contrast learns the domain-invariant features from a small number of sample pairs. The learned fault features can then be used for fault identification without parameter fine-tuning. In two case studies, we validated the performance of the proposed framework with small training samples under varying speeds, loads, and significant noises. Compared with the state-of-the-art methods, the proposed solution achieved higher diagnostic accuracy for the targeted applications.
更多查看译文
关键词
Contrastive learning,intelligent fault diagnosis,small samples,variable working conditions
AI 理解论文
溯源树
样例
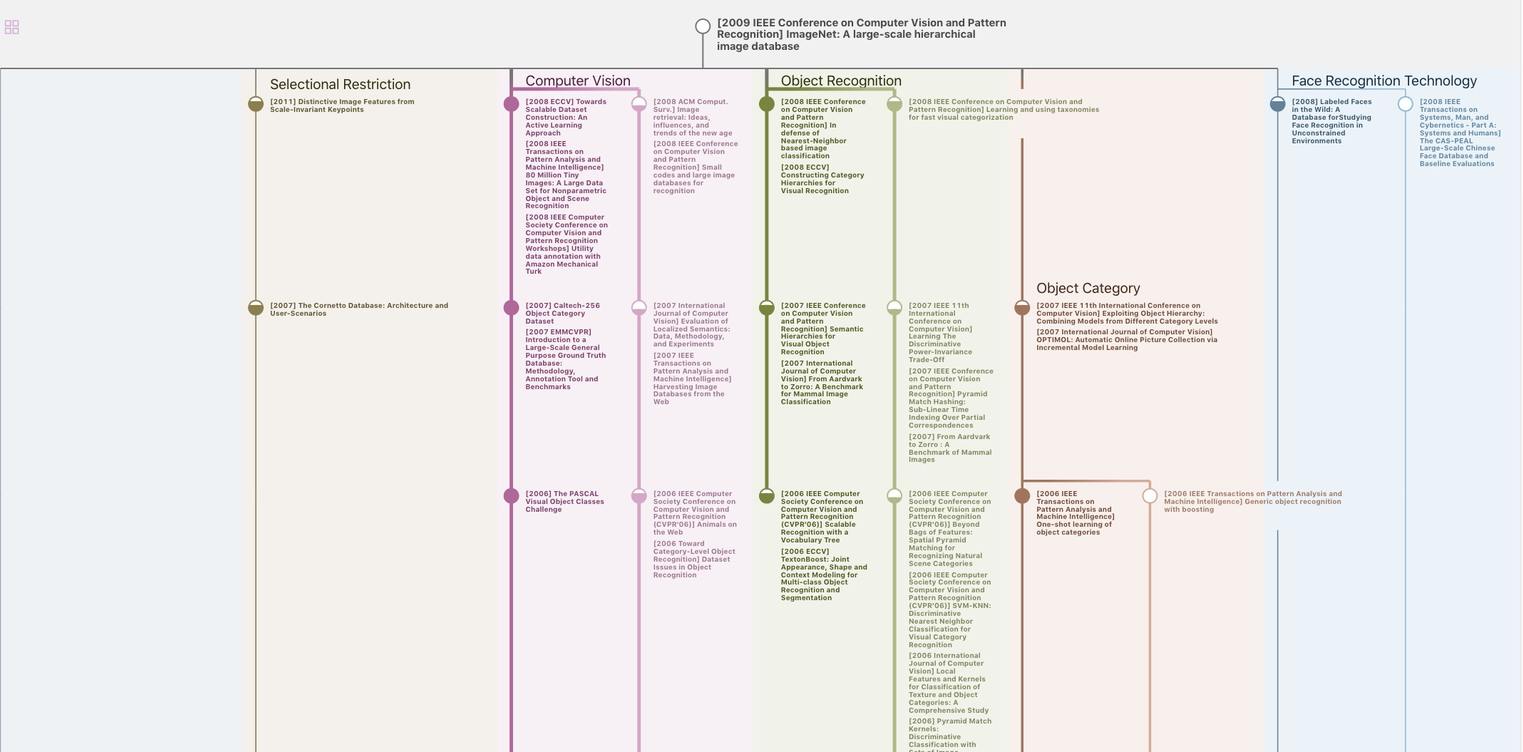
生成溯源树,研究论文发展脉络
Chat Paper
正在生成论文摘要