Data-Driven Probabilistic Dynamic Analysis for Power Systems with Correlated Renewable Energy Sources based on Polynomial Chaos Expansion
2022 IEEE Power & Energy Society General Meeting (PESGM)(2022)
Abstract
Renewable energy sources (RES) is an emerging and fast-growing power resource worldwide and may cause uncertainty issues in power systems. This paper proposes a probabilistic analysis algorithm to quantify the uncertainty characteristics of power system dynamic stability with multiple correlated renewable generators by using an efficient tool named polynomial chaos expansion (PCE). The actual historical data of RES can be utilized directly to establish the probabilistic model based on kernel density estimation (KDE). The correlation among adjacent renewable generators could greatly affect the uncertainty results, but traditional PCE method is only suitable for uncorrelated input variables. The proposed algorithm extends the application of PCE method by adopting Nataf transformation to deal with the correlation among renewable generators. Simulations are performed on IEEE 39 bus system, and the probabilistic results reveal the accuracy and efficiency of proposed approach compared with Monte Carlo simulation (MCS).
MoreTranslated text
Key words
probabilistic dynamic analysis,polynomial chaos expansion,correlated renewable energy sources,power systems,renewable energy,data-driven
AI Read Science
Must-Reading Tree
Example
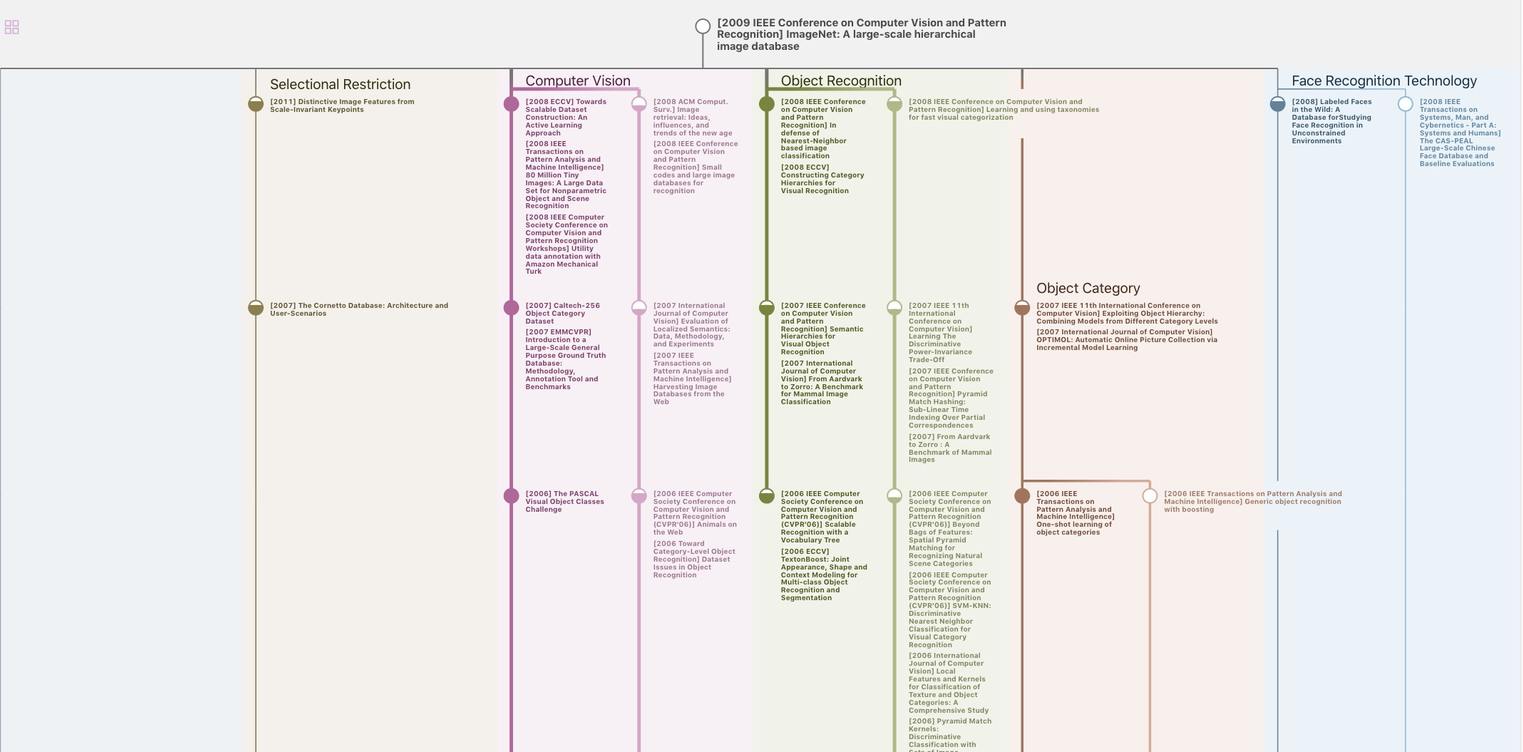
Generate MRT to find the research sequence of this paper
Chat Paper
Summary is being generated by the instructions you defined